AWS SageMaker vs Azure ML: A Comprehensive Comparison
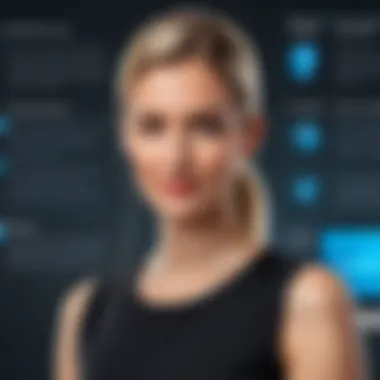
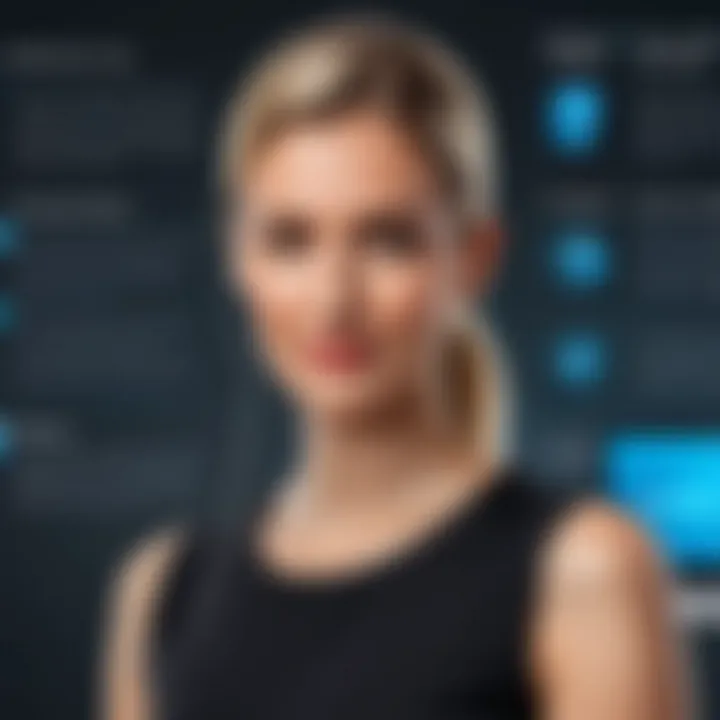
Intro
In the rapidly changing landscape of machine learning and artificial intelligence, organizations find themselves in a scramble to harness the potential of cloud-based platforms. Two giants in the realm of cloud services – AWS SageMaker and Azure Machine Learning – stand out for their robust features and functionalities. But as businesses dive deeper into the world of AI, it's crucial to grasp the nuances of these powerful tools.
Understanding these platforms doesn't just help in picking the right one; it shapes how teams approach their machine learning projects and ultimately impacts their operational success.
In this comparative analysis, we will break down each platform by examining its purpose, key functionalities, and user experience to help demystify the strengths and weaknesses of each.
Overview of Software
Purpose and Use Cases
Both AWS SageMaker and Azure Machine Learning serve a similar purpose: to provide developers and data scientists with the resources they need to build, train, and deploy machine learning models. However, they do so through slightly different lenses.
AWS SageMaker is renowned for its versatility, supporting a range of use cases from predictive analytics to natural language processing. It’s particularly favored by companies that are already within the Amazon ecosystem.
On the flip side, Azure Machine Learning caters to businesses that might have a more Microsoft-centric workflow. It seamlessly integrates with tools like Microsoft Excel and Azure DevOps, making it appealing for enterprises with existing investments in Microsoft products.
Key Features
In diving into the specific features, several aspects deserve attention:
- Built-In Algorithms: AWS SageMaker provides a suite of built-in algorithms and also allows users to bring their own, offering flexibility for specialized needs. Azure Machine Learning also provides numerous algorithms but emphasizes a no-code design approach, appealing to less technical users.
- Model Deployment: Both platforms allow for easy deployment and scaling of models. AWS has an edge with its managed services that promote faster model optimization and deployment. Azure, however, excels in automation features that streamline the deployment process through DevOps integration.
- Cost Structure: When it comes to pricing, AWS generally operates on a pay-as-you-go model while providing free tiers for new users. Azure's pricing also varies based on usage but might include additional costs for premium support. Organizations should consider their expected utilization carefully, as costs can escalate.
"Understanding these platforms doesn’t just help in picking the right one; it shapes how teams approach their machine learning projects and ultimately impacts their operational success."
In-Depth Review
Performance Analysis
Performance can be a game-changer, especially in time-sensitive applications. AWS SageMaker boasts faster training times due to its superior infrastructure and optimization techniques. Many users report that it handles large datasets with ease and gives quick results.
Azure Machine Learning, while equally competent, might lag in performance for exceedingly large datasets. However, it shines in collaborative features, allowing multiple users to work on projects simultaneously without a hitch.
User Interface and Experience
User experience is critical in education and deployment. AWS SageMaker’s interface is straight-forward but can be overwhelming for first-time users due to the sheer number of options.
Azure Machine Learning offers a more visually appealing and intuitive interface, which can ease the learning curve for newcomers to machine learning. Features like drag-and-drop workflows make it simpler for teams familiar with software development but not with machine learning.
Prelims to Cloud-Based Machine Learning
In today’s digital landscape, cloud-based machine learning is more than just a buzzword; it’s a vital cog in the machinery of modern business. Companies are increasingly leaning on this technology to enhance efficiency, analyze data, and drive decision-making processes. This article seeks to unpack what cloud-based machine learning is, particularly through the lenses of AWS SageMaker and Azure Machine Learning. By understanding these platforms, businesses can make better choices that align with their unique requirements.
The Rise of Machine Learning in Business
Machine learning is no longer something reserved for tech giants. From retailers to healthcare providers, businesses across various sectors are harnessing its power to glean insights from vast datasets. For instance, e-commerce platforms utilize machine learning algorithms to tailor consumer experiences by predicting what products a user is likely to purchase based on their browsing history. In healthcare, predictive analytics aids in early disease detection, improving patient outcomes and streamlining hospital operations.
In essence, the rise of machine learning in business embodies the shift toward data-driven strategies, enabling companies to react swiftly to changes in market demands. However, the complexity of implementing these systems can be daunting. This is where cloud solutions come into play, simplifying the process significantly and allowing businesses, regardless of size, to tap into machine learning capabilities without the need for extensive infrastructure.
Overview of Cloud Platforms
Cloud platforms provide the backbone for machine learning initiatives, offering scalable resources and tools that can adapt to changing needs. AWS SageMaker and Azure Machine Learning are two prominent players in this field, each presenting unique strengths and features.
AWS SageMaker allows users to build, train, and deploy machine learning models quickly, while providing an array of built-in algorithms. Meanwhile, Azure Machine Learning emphasizes integration with other Azure services, improving data handling and model management.
Both platforms democratize machine learning, giving access to powerful tools without requiring deep technical expertise.
- Flexibility and Scalability: Users can ramp up resources as their needs grow, ensuring cost-efficiency.
- Accessibility: Cloud-based solutions remove geographic barriers, allowing global collaboration among teams.
- Advanced Security: These platforms often come with robust built-in security, ensuring data protection during the machine learning lifecycle.
"Leveraging cloud-based machine learning solutions is no longer a luxury; it’s a necessity for businesses looking to stay competitive in a data-driven world."
An Overview of AWS SageMaker
AWS SageMaker has carved a prominent niche in the realm of cloud-based machine learning, emerging as a comprehensive solution for organizations aiming to harness the power of AI. This platform's robust set of features provides users with the tools necessary for building, training, and deploying machine learning models at scale. Understanding these elements is paramount for businesses looking to integrate machine learning into their workflows effectively.
SageMaker epitomizes the notion of accessibility, making machine learning approachable even for those who might not have extensive expertise in the field. It offers a plethora of options, from pre-built algorithms to refined customization capabilities, which ensures that users can suit their projects precisely according to their requirements. Additionally, the ability to handle data of varying sizes and complexities positions SageMaker as a versatile player in the industry.
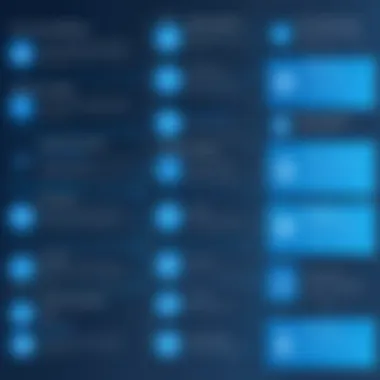
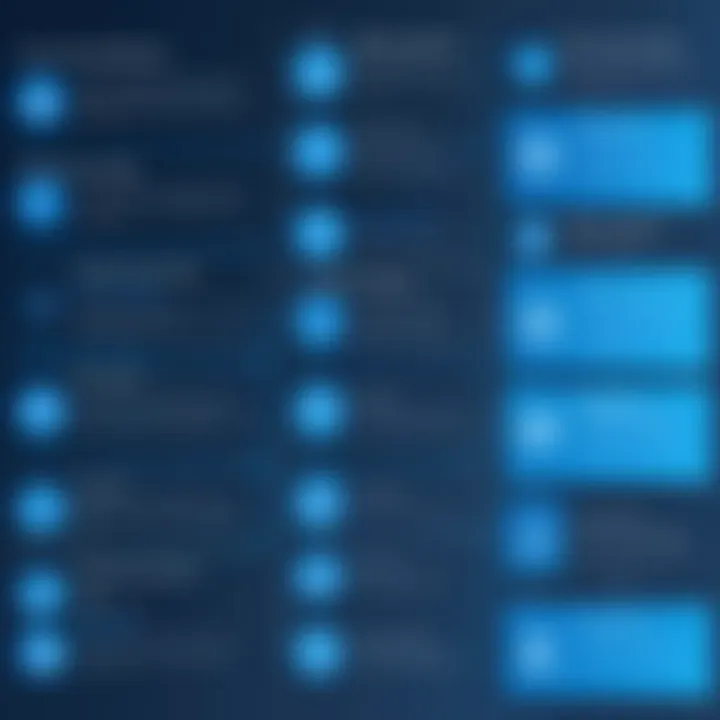
The relevance of AWS SageMaker in discussions surrounding cloud machine learning platforms can't be overstated. As organizations navigate the waters of digital transformation, having access to an efficiently integrated platform helps reduce both the time to market and potential resource costs associated with machine learning projects.
Key Features of SageMaker
AWS SageMaker is a powerhouse when it comes to features that elevate the machine learning experience. Some of its standout elements include:
- Integrated Jupyter Notebooks: With SageMaker’s built-in Jupyter notebooks, users can conduct exploratory analysis, data visualization, and model prototyping all in one place. This integration streamlines the workflow considerably, saving time and increasing productivity.
- Model Training at Scale: The platform allows users to train multiple models simultaneously, leveraging the cloud's scalable architecture. SageMaker's diverse instance types cater to a variety of model sizes and complexities, ensuring optimal performance.
- Automatic Model Tuning: This feature, often referred to as hyperparameter optimization, enhances model accuracy by systematically testing various parameter combinations. Rather than guessing, developers can rely on SageMaker to find the proverbial needle in a haystack, optimizing outcomes efficiently.
- Multi-Model Endpoints: Deploying multiple models simultaneously can lead to better resource management. With this feature, organizations can host numerous models at once without the need to spin up separate endpoints for each, significantly cutting down on deployment costs.
- Built-in Algorithms: SageMaker comes equipped with a variety of built-in algorithms, facilitating the immediate application of machine learning techniques across different challenges, from image analysis to text classification.
Each of these features is designed to smooth out the bumps on the road of machine learning development, equipping users to scale their projects with confidence.
Use Cases and Applications
The versatility of AWS SageMaker shines through in its wide range of use cases across industries. Here are a few notable applications:
- Healthcare: Predictive analytics using SageMaker can lead to better patient outcomes. For instance, hospitals use it to forecast patient admission rates based on historical data, optimizing staffing and resources accordingly.
- Finance: Banks deploy SageMaker for fraud detection. By analyzing transaction patterns in real-time, institutions can identify irregularities and reduce the risk of financial crime, thus safeguarding both their interests and those of their customers.
- Retail: Companies in retail tap into SageMaker to enhance the customer experience. By employing recommendation systems powered by machine learning, businesses can provide personalized product suggestions to shoppers based on their browsing behavior and preferences.
- Manufacturing: SageMaker can be utilized for predictive maintenance in manufacturing settings. By analyzing equipment performance data, organizations can predict failures before they occur, saving costs on repairs and minimizing downtime.
These examples illustrate the extensive applicability of SageMaker in various sectors, reinforcing its tenet of proving beneficial across a wide spectrum of use cases. By embracing AWS SageMaker, organizations not only adopt an advanced machine learning platform but also position themselves at the forefront of innovation in their respective fields.
A Detailed Look at Azure Machine Learning
Azure Machine Learning stands at the forefront of cloud-based AI, providing a versatile platform that caters to a myriad of machine learning needs. The importance of this topic in our comparative analysis rests on its robust capabilities, which can empower businesses to innovate and optimize their workflows. In the rapidly evolving landscape of technology, having an understanding of Azure ML is essential for those aiming to leverage machine learning effectively. By immersing ourselves into Azure ML's framework, we can highlight several key elements that make it a contender worthy of consideration alongside AWS SageMaker.
Core Components of Azure
Azure Machine Learning is built on a foundation of core components that facilitate a streamlined process for data scientists and developers alike. At its heart lies the Azure Machine Learning workspace, an organized environment that serves as a management hub for various activities, from project initiation to model deployment. Key features include:
- Datasets: Azure ML enables users to create, manage, and share datasets, simplifying data ingestion and preparation.
- Compute Resources: The platform supports diverse compute resources, whether it's local or cloud-based, maximizing flexibility according to project requirements.
- Designer: This visual drag-and-drop interface allows users to create machine learning models without extensive coding knowledge, which can be ideal for teams with mixed skill sets.
- Automated Machine Learning (AutoML): This feature streamlines the process of model selection and hyperparameter tuning, enabling quicker iterations and reducing the burden on users.
- Integration with Azure services: Azure ML seamlessly integrates with other Azure services such as Azure Data Lake, making data management and model training a more cohesive experience.
These components blend to form a powerful ecosystem that accelerates the journey from data to deployable insights. They emphasize Azure ML's commitment to making machine learning accessible and efficient, whether one is just starting or looking to scale up.
Practical Applications in Business
Azure Machine Learning serves as a tool that businesses can leverage to tackle a variety of challenges across different industries. Here are some notable practical applications:
- Predictive Maintenance: Manufacturing firms can utilize Azure ML to analyze sensor data from machinery, predicting failures before they occur and reducing downtime.
- Customer Insights: Retail businesses can harness machine learning algorithms to analyze customer behavior, offering personalized recommendations and enhancing customer satisfaction.
- Fraud Detection: Financial institutions can deploy models that analyze transaction patterns, helping in the identification of potentially fraudulent activities in real time.
- Supply Chain Optimization: By predicting demand and optimizing inventory levels, companies can significantly enhance their supply chain efficiency.
Emerging technologies are continually altering the course of business strategies, and Azure ML ensures that organizations can stay competitive. The case studies from firms employing Azure Machine Learning show the tangible effect it can have — from cost savings to improved decision-making.
"The proactive use of advanced analytics not only enables organizations to make informed decisions but also fosters a culture of innovation."
In summary, Azure Machine Learning provides a structured yet flexible approach to machine learning. Its core components empower users to experiment and implement solutions that drive real business value. As we explore further comparisons with AWS SageMaker, having a clear understanding of Azure ML will underscore the unique advantages and challenges each platform presents.
Comparative Metrics: AWS SageMaker vs Azure Machine Learning
Understanding the comparative metrics between AWS SageMaker and Azure Machine Learning is crucial for organizations that are looking to harness the power of AI. As these platforms evolve, they offer various features, tools, and integrations that can dramatically influence project outcomes. Companies considering machine learning solutions must account for specific considerations like cost, usability, and support. This section embarks on a thorough analysis of pivotal elements that can guide enterprises in selecting the platform that best fits their operational demands.
Cost Analysis
The first hurdle often faced when choosing between AWS SageMaker and Azure Machine Learning is determining the financial implications. Budget constraints are common in both large enterprises and startups. Understanding the pricing structure is essential for project viability.
AWS SageMaker employs a pay-as-you-go model. Users incur charges based on several factors including compute resources, storage, and data transfer. This model can be beneficial for projects that fluctuate in scale, allowing firms to pay only for what they use. The cost can add up, especially if not carefully monitored.
In contrast, Azure Machine Learning also follows a consumption-based pricing approach. However, it offers additional pre-paid options, which enable organizations to purchase reserved capacity at a reduced rate. This can work wonders for long-term projects that require consistent resource allocation.
Both platforms provide calculators to estimate costs, yet real-world expenses can differ significantly from initial estimates. Hence, a thorough project analysis with detailed resource forecasting is crucial to avoid unanticipated expenses.
Usability and Learning Curve
Usability can significantly affect the onboarding process for teams new to machine learning. AWS SageMaker is known for its user-friendly interface but can become complex due to its vast array of features. For developers familiar with Python, the SageMaker Studio offers an integrated development environment that simplifies model creation.
On the flip side, Azure Machine Learning boasts a diverse set of tools that cater to varying levels of expertise. It's designed to be approachable for beginners with its drag-and-drop interface. However, those who dive deeper may find the specialized capabilities challenging to master without prior exposure to Azure's ecosystem.
Choosing a platform that aligns with your team's existing skill sets can reduce the learning curve and foster a more efficient development process.
Integration with Other Services
When it comes to integrating machine learning services with other applications, both platforms bring unique strengths to the table. AWS SageMaker seamlessly ties into many of AWS's robust offerings like AWS Lambda and Amazon S3, allowing easy data ingestion and model deployment. This ecosystem cohesiveness is beneficial for teams already entrenched in the AWS landscape.

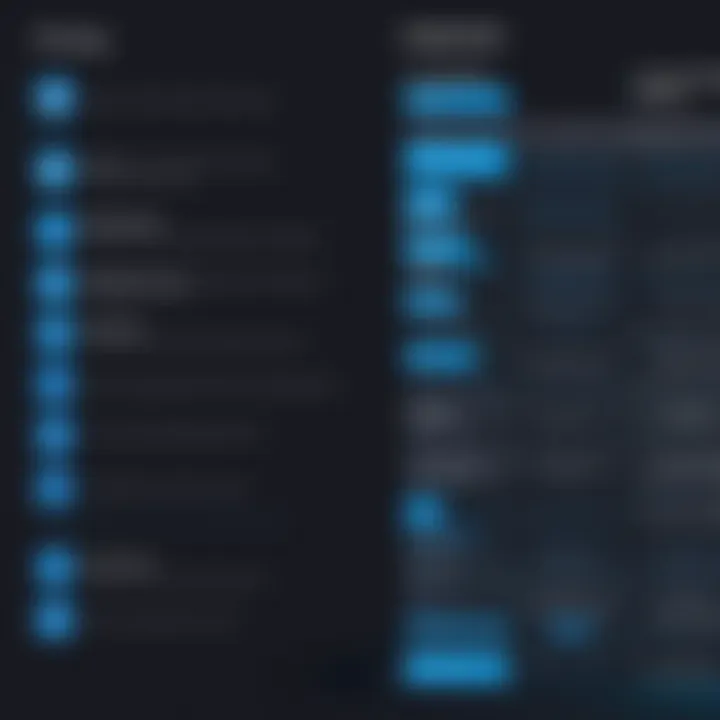
Azure Machine Learning, however, shines with its Power Platform and Microsoft ecosystem integrations. For organizations utilizing Office 365 or Dynamics 365, the interoperability can increase productivity. Additionally, Azure has built-in connectors for hundreds of third-party applications, streamlining the flow of data between systems. Choosing the right platform usually rests on existing infrastructure considerations.
Support and Documentation
Support structure and documentation play vital roles in facilitating a smooth user experience. AWS provides a comprehensive suite of documentation, ranging from guides to tutorials and forums to address common queries. It maintains active, robust customer support for users reaching out for help. However, navigating through extensive documentation may sometimes feel overwhelming due to its sheer volume.
Azure Machine Learning, on the other hand, tends to be more user-friendly when it comes to documentation. Microsoft has invested in creating clear, concise guides, often complemented by examples and community contributions. Additionally, Azure's support plans are tiered, offering different levels of assistance depending on organizational needs.
In summary, the choice between AWS SageMaker and Azure Machine Learning extends beyond surface-level metrics. Potential users must closely consider costs, ease of use, integration capabilities, and the quality of support and documentation. These comparative metrics serve as a straightforward guide to help organizations make informed decisions tailored to their specific machine learning ambitions.
Machine Learning Tools and Frameworks Comparison
When diving into machine learning, selecting the right tools and frameworks is like choosing the right ingredients for a gourmet dish. It’s essential because these tools directly influence how smoothly the development, testing, and deployment of models go. Both AWS SageMaker and Azure Machine Learning provide a buffet of options catering to various needs, making this comparison crucial for businesses and developers alike.
Understanding the features of different machine learning tools helps teams align their project goals with the right technology. Here are some key elements to consider when comparing these platforms:
- Model Creation: Different tools offer unique functionalities for building and training models. This selection often affects performance and efficiency.
- Ease of Integration: It’s not just about creating models but also how they fit into existing workflows or systems. Good tools allow for seamless integration, saving time and effort.
- Support for Algorithms and Libraries: The variety of frameworks supported plays a significant role in the flexibility and capability of each platform.
Ultimately, comparing these tools helps users capitalize on their strengths, ensuring they’re not just investing in a software solution, but a platform backed by rich capabilities, tailored for their specific needs.
Model Creation and Training Tools
The process of model creation is where the magic begins. In the realm of AWS SageMaker, the platform offers various built-in algorithms that cater to multiple tasks ranging from regression to classification. It also supports popular frameworks such as TensorFlow, PyTorch, and Apache MXNet. This flexibility allows developers to choose their preferred environment or leverage existing codebases without a hassle.
On the other hand, Azure Machine Learning also boasts a rich set of tools for model training. The platform enhances the user experience through features like automated machine learning (AutoML), where the system intelligently selects the best models and hyperparameters based on the given datasets. This can be a game changer for those who may not have in-depth expertise in choosing the best algorithms.
Additionally, both platforms provide Jupyter notebook support, fostering an interactive environment for experimentation. The ease of access for real-time coding and visualization enables users to iterate quickly and refine their models. Cloud-based options mean no local setup issues, which is always a plus.
Deployment and Management Features
Once a model is trained, the next stage is deployment. This phase is crucial, as it determines how well a model performs in a live environment. AWS SageMaker shines here with its one-click deployment feature, allowing users to rapidly deploy their models into production without extensive setup.
Moreover, SageMaker provides capabilities like multi-model endpoints, enabling multiple models to be hosted on a single endpoint. This can optimize costs and resource usage, offering scalability in line with demand.
Similarly, Azure Machine Learning offers robust deployment features. It includes mechanisms for continuous integration and continuous delivery (CI/CD) which streamline ongoing updates, a necessity for keeping models relevant over time. Azure also excels with its integration to Azure DevOps, allowing teams to automate their deployment processes effectively.
In terms of management, both platforms come with monitoring tools to assess model performance post-deployment. This is vital for identifying when retraining might be necessary or for analyzing the need for further adjustments.
"Choosing the right tools for machine learning can often determine the success of an AI project. The nuanced differences between platforms can make all the difference."
Performance and Scalability Considerations
In the world of cloud-based machine learning, performance and scalability are crucial factors that often make or break organizations’ efforts to implement effective AI solutions. As businesses churn through a plethora of data, the need for efficient processing and the ability to scale resources up or down accordingly becomes paramount. Therefore, understanding the performance metrics and scalability options of AWS SageMaker and Azure Machine Learning not only equips organizations with the tools to handle current workloads but also prepares them for future demands. These considerations highlight how each platform measures performance through various benchmarks, and how their respective architectures support the elasticity needed for diverse workloads.
Benchmarking Performance
When evaluating AWS SageMaker and Azure Machine Learning, benchmarking performance brings clarity into their operational efficiency. Often, benchmarking is done through specific machine learning tasks or datasets that reflect real-world applications. The process involves assessing how quickly each platform can train models, handle large datasets, and predict outcomes, which ultimately influences the speed to market for AI applications.
AWS SageMaker is known for its ability to streamline training processes by optimizing resource allocation based on demand. For instance, when training large models, using Amazon Elastic Compute Cloud (EC2) instances allows businesses to leverage high-performing GPU instances, significantly speeding up the training time. With built-in automatic model tuning, SageMaker can also adaptively adjust parameters to find the best possible results.
In contrast, Azure Machine Learning provides a range of capabilities for monitoring and optimizing model performance. By utilizing built-in tools such as Azure Monitor, users can gain insights into model performance and adjust settings in real-time for better outcomes. Azure also offers different hardware configurations tailored for specific tasks, enabling organizations to tweak resources according to their project needs.
"Performance measures such as training duration and prediction latency often dictate how smoothly an AI project progresses and whether it meets business objectives."
Scalability Options and Limitations
Scaling efficiently is about having the right flexibility to handle fluctuations in demand without incurring unnecessary costs. Both AWS SageMaker and Azure Machine Learning offer robust scalability, yet there are nuances worth noting.
AWS SageMaker’s architecture thrives on its ability to scale horizontally. This means when workloads increase, businesses can easily spin up more instances of compute power to tackle greater demands. This capability extends to not just model training but also deployment— scaling out endpoint capacity for predictions is straightforward, allowing applications to serve multiple users or larger datasets without a hitch.
On the flip side, Azure Machine Learning offers scalability via its integrated model management system. Users can deploy models as web services that can scale to meet incoming requests, an approach that aligns well with cloud-based architectures. Nonetheless, organizations might find that certain configurations can hit limitations based on the predefined account quotas or subscription levels, which can constrain the scaling efforts in some instances.
In summary, understanding how SageMaker and Azure Machine Learning measure performance and offer scalability can lead organizations to make more informed decisions. Either of these platforms provides flexible options, but the key lies in aligning their capabilities with the unique demands of individual projects.
Security Features in Cloud Machine Learning Platforms
As businesses dive deeper into the world of cloud computing and machine learning, security in these platforms becomes not just a checkbox but a critical pillar for sustaining operational integrity. It’s like walking a tightrope – one misstep and the balance is lost, compromising sensitive data and privacy.
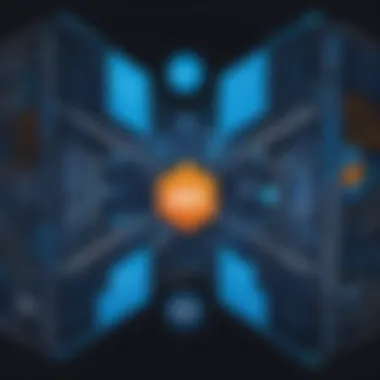
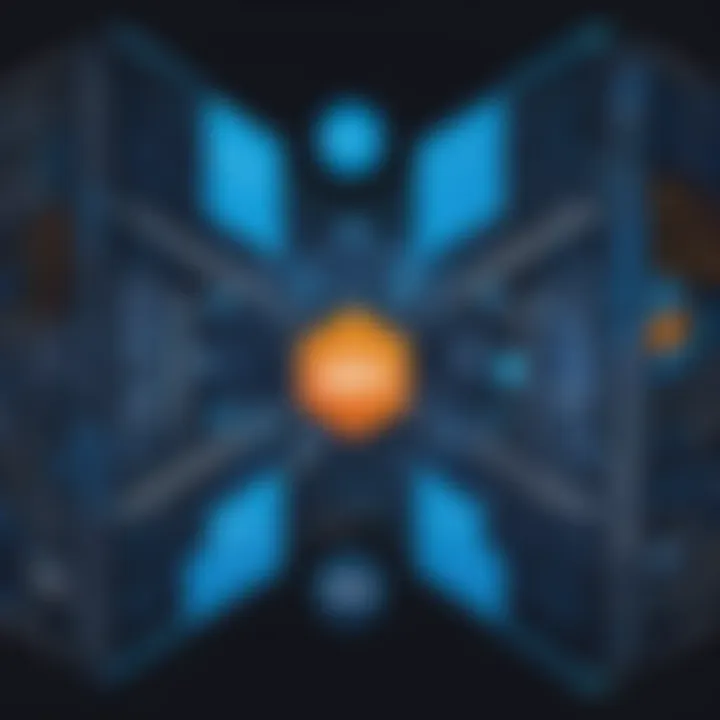
Both AWS SageMaker and Azure Machine Learning recognize this enormity, thereby embedding rigorous security features into their frameworks. The emphasis lies on protecting data at every nook and cranny—during transfer, while resting, and even in processing. Here, we’ll peel back the layers of data protection strategies and compliance protocols that keep your machine learning endeavors secure and aligned with ethical standards.
Data Protection Strategies
Data protection serves as the frontline defense against unauthorized access and potential breaches. It’s not just about locking the gates but also about ensuring those gates are constructed with the strongest metal. For AWS SageMaker, this encapsulates multiple layers:
- Encryption: SageMaker employs data encryption both at rest and in transit. AES-256 encryption is standard practice, safeguarding every byte as it travels.
- Access Control: AWS Identity and Access Management (IAM) gives you granular control over who accesses what data. This ensures only authorized users can get into sensitive areas, akin to having a club where only invited members can enter.
- Network Isolation: Virtual Private Cloud (VPC) integration allows users to isolate their resources, making them less vulnerable to external attacks.
In contrast, Azure Machine Learning doesn't lag in this aspect either:
- Data Encryption: Azure offers encryption for data at rest via Azure Storage Service Encryption and in transit using SSL/TLS protocols.
- Role-Based Access Control (RBAC): Azure's RBAC allows fine-tuned access management similar to a strict bouncer at a popular venue, ensuring that only specific roles have clearance to certain areas.
- Private Endpoints: This feature lets you securely link your Azure Machine Learning workspace to virtual networks, establishing a direct connection that’s safeguarded against public internet vulnerabilities.
This dual approach effectively creates a robust data protection strategy that not only safeguards sensitive data but also fosters trust in automated processes.
Compliance and Ethical Considerations
Ensuring compliance with various regulations such as GDPR, HIPAA, or PCI-DSS is pivotal in today’s digital landscape. A slip-up here could lead to hefty fines and tarnished reputations. The platforms are not just building secure environments but also ensuring that these environments comply with the legislation that governs data privacy.
- AWS Compliance: AWS has a plethora of compliance certifications that reassure users about their commitment. This includes industry standards and regulations. Regular audits assure customers that their data sanitation practices are up to scratch.
- Azure Compliance: Just like AWS, Azure also provides transparency into compliance status, with tools to help organizations understand and manage compliance risks effectively.
Moreover, ethical considerations are not an afterthought. Both platforms advocate for responsible AI practices. For instance, they encourage bias mitigation in algorithm training and transparency in data sourcing. When leveraging machine learning, it’s important to consider the ethical ramifications—open discussions on data biases and their implications should be an integral part of your workflow.
To summarize, the security features of both AWS SageMaker and Azure Machine Learning hold paramount importance in safeguarding data. Robust data protection strategies and compliance measures become the bedrock for trust and integrity in the world of cloud-based machine learning.
"In a world where data breaches make headline news, investing in security is no longer optional but necessary for survival."
By leveraging these security features, organizations can ensure that their journey into machine learning is fortified against potential risks.
User Experiences and Case Studies
User experiences and case studies serve a significant role in understanding how cloud-based machine learning platforms like AWS SageMaker and Azure Machine Learning operate in the real world. For practitioners in tech—be it software developers, IT professionals, or even students—these narratives provide not just practical insights but also a sense of the effectiveness of each platform. They reveal how others have navigated challenges, leveraging tools to fulfill unique project requirements. Consequently, such user-focused information becomes invaluable for those on the fence about their cloud ML choices.
Evaluating user experiences can help in various ways:
- Highlighting Practical Use Cases: Case studies showcase specific implementations, illustrating how businesses leverage ML to drive real results.
- Identifying Common Challenges: Learning from others’ difficulties can inform one’s strategy and help avoid potential pitfalls during implementation.
- Assessing Overall Satisfaction: User reviews often bring personal touches to the data, revealing the general sentiment towards the performance and usability of the platforms.
Success Stories with AWS SageMaker
AWS SageMaker boasts numerous success stories that testify to its potential. For instance, a healthcare startup employed SageMaker for developing predictive models aimed at assessing patient outcomes. Using SageMaker’s built-in algorithms, the team was able to streamline model training times dramatically, reducing weeks of work into mere hours. As a result, they rolled out their solution to healthcare providers quicker, directly impacting patient care.
Another company in the finance sector used SageMaker’s capabilities to develop a risk assessment tool. The organization integrated several data sources, and thanks to SageMaker’s robust framework, they could parallel process large datasets, which ultimately led to improved predictive accuracy in their risk evaluations. Both of these narratives signal that AWS SageMaker is a viable option for those aiming to build complex ML solutions.
Case Studies of Azure Machine Learning Implementations
On the other hand, Azure Machine Learning also has its fair share of success stories. For example, a retail business sought to enhance its customer engagement strategies. By leveraging Azure ML, they created models that analyzed customer purchase patterns which enabled them to tailor marketing campaigns effectively. The outcome was a notable uptick in their customer conversion rates.
Another case in the manufacturing sector highlights how Azure’s capabilities offered predictive maintenance for equipment. By employing Azure ML, engineers were able to analyze historical data to predict failures before they occurred. This allowed for timely interventions that prevented costly downtimes. The stories illustrate Azure’s powerful analytical capabilities that businesses in various sectors can leverage efficiently.
"Success stories provide crucial insights that can shape the direction of future projects and decision-making within organizations."
Each success story serves as a blueprint, detailing what worked and the hurdles faced along the way. They shed light on how effective AWS SageMaker and Azure Machine Learning can be when put into practice, guiding future adopters to make informed decisions tailored to their specific needs.
Concluding Remarks
The concluding remarks of this article serve as a pivotal synthesis of the deep dive into AWS SageMaker and Azure Machine Learning. It’s not just about summarizing what has been explored but solidifying the understanding of how these platforms can be leveraged for machine learning endeavors. As many businesses shift toward cloud-based solutions, the ability to make well-informed choices can’t be overstated. With the myriad of features and functionalities outlined in previous sections, connecting the dots here acts as a compass, guiding users to effectively navigate this complex landscape.
Understanding the key findings allows organizations to weigh the pros and cons of each platform based on specific needs. While pricing structures, usability, and integration capabilities are important, the nuances that differentiate AWS SageMaker from Azure Machine Learning can significantly influence real-world applications. Users must think about what tools resonate most with their workflows and which platform’s strengths align with their future aspirations in automation and AI.
"Choosing the right platform today shapes the results you’ll achieve tomorrow."
Summary of Findings
In comparing AWS SageMaker and Azure Machine Learning, several critical observations emerged:
- Cost Efficiency: Azure Machine Learning tends to have lower starting costs, which can be appealing for startups and small businesses. Conversely, SageMaker offers a flexible pay-as-you-go model, potentially more beneficial for larger enterprises with variable usage patterns.
- Usability: Both platforms showcase user-friendly interfaces but differ in their user experience. SageMaker is often praised for its comprehensive documentation and clear tutorials, while Azure’s integrations with other Microsoft services can streamline use for those already embedded in that ecosystem.
- Integration Potential: With Azure Machine Learning, seamless integrations with other Azure services stand out. SageMaker shines with Amazon's range of offerings, allowing users to build more extensive applications by linking to tools like Amazon S3 and DynamoDB.
- Community and Support: Both platforms enjoy strong community support, yet the AWS community has been more established over the years. In contrast, Azure’s support may be seen as superior for those needing assistance within Microsoft’s cloud offerings.
These findings illuminate potential pathways for decision-makers, especially in tech-driven businesses seeking to harness the prowess of machine learning effectively.
Final Recommendations
Given the landscape painted throughout this article, here are a few recommendations tailored to different scenarios:
- For Startups and Innovators: Embrace Azure Machine Learning for its cost-effective entry point and supportive ecosystem if your business is already using Microsoft products. This combination could lead to more efficient deployment and maintenance.
- For Established Enterprises: Opt for AWS SageMaker as it offers robust tools and frameworks for scaling projects and a broader suite of supportive services if you seek a comprehensive machine learning solution.
- Data-Driven Companies: If your focus is deeply rooted in data analytics, consider diving into Azure’s offerings, especially if your team has expertise within Microsoft environments.
- Community Engagement: Whichever option you choose, actively engage with existing user communities on forums like Reddit or organizational pages on Facebook. These platforms are treasure troves of real-life insights and experiences that can enhance your understanding.
Navigating the machine learning landscape doesn’t have to be daunting. By synthesizing the insights drawn from AWS SageMaker and Azure Machine Learning, organizations can align their strategies toward generating impactful results that drive their business forward.