In-Depth Insights on Business Forecast Systems
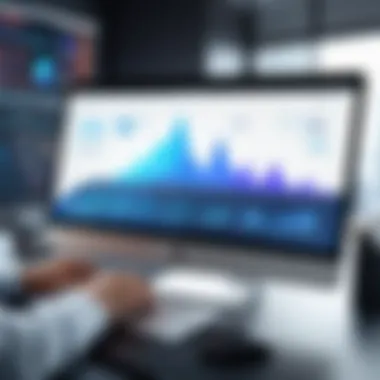
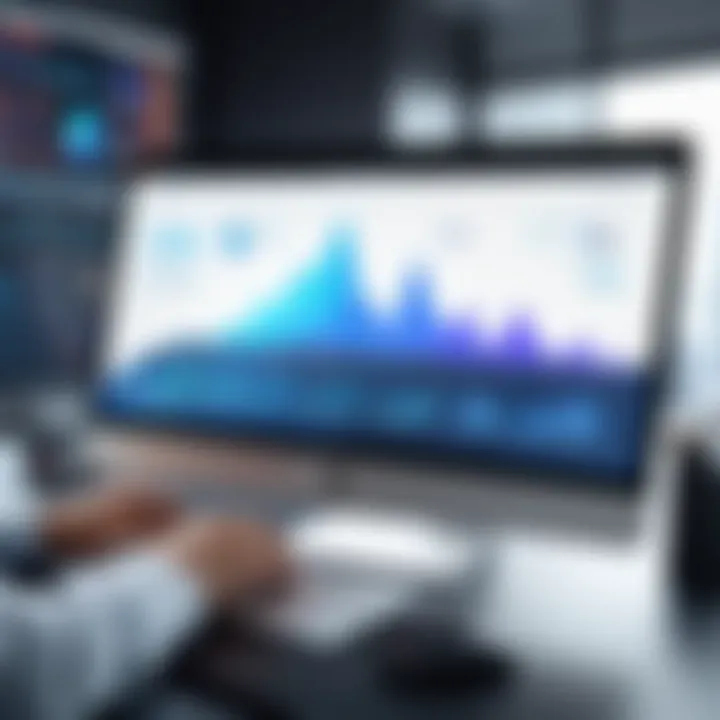
Intro
In the realm of commerce, the ability to anticipate future trends, behaviors, and outcomes can make or break an enterprise. Business forecast systems serve as vital instruments that help organizations transcend mere guesswork, relying on structured methods and advanced analytics instead. From retail to manufacturing, understanding demand fluctuations and market dynamics is pivotal for maintaining a competitive edge.
The methodologies employed within forecasting range from time-tested models to cutting-edge artificial intelligence approaches. Each system presents its own set of advantages and potential pitfalls, thus creating a rich landscape of options for decision-makers.
This guide carefully unpacks the multiple layers of business forecasting, highlighting why systems are indispensable in todayâs fast-paced, data-driven world. Readers are encouraged to consider how effective forecasting can not only refine operational strategies but also provide deeper insights into customer behaviors.
Overview of Software
Purpose and Use Cases
At the core of every successful business forecast system lies the goal of enhancing decision-making capabilities. By converting historical data into actionable insights, these systems can effectively analyze trends and project future business conditions.
Some common use cases include:
- Sales Forecasting: Accuracy in predicting future sales is crucial for inventory management and resource allocation.
- Financial Planning: Businesses can make more informed budgetary choices through reliable income projections.
- Supply Chain Management: Anticipating demand spikes can optimize inventory levels, reducing costs associated with overstock or shortages.
Key Features
When exploring business forecast systems, itâs important to grasp the features that set apart high-performing solutions from the rest.
Here are some essential attributes to consider:
- Data Integration Capabilities: The ability to connect with various data sources is critical for comprehensive analysis.
- Scenario Analysis: Advanced systems allow users to create multiple scenarios based on varying assumptions to gauge potential impacts.
- User-Friendly Dashboards: Visual representations of data can enhance interpretability and facilitate quicker decision-making.
In-Depth Review
Performance Analysis
Performance can be measured through a variety of metrics, notably accuracy and speed of forecasts. Systems that utilize machine learning methods often adapt better and provide increasingly accurate predictions as new data emerges.
Some industry benchmarks to consider include:
- Mean Absolute Percentage Error (MAPE): A key metric that reflects forecasting precision.
- Lead Time Reduction: Time saved during the forecasting phase can directly impact operational efficiency.
User Interface and Experience
A well-designed user interface plays a significant role in maximizing a systemâs effectiveness. Platforms that prioritize user experience inevitably encourage broader adoption within organizations. Features worth noting include:
- Intuitive Navigation: Users should be able to find necessary functionalities effortlessly.
- Customization Options: Tailored dashboards that reflect user-specific needs can significantly enhance engagement.
"A good user experience is not just about making the software easy to use; itâs about making it useful in the real world of business challenges."
End
As we traverse the intricacies of business forecast systems, it becomes abundantly clear that they are not merely technological tools but essential partners in strategic planning. The right system, when aligned with organizational goals, can offer a decisive advantage in an era rich with data yet fraught with uncertainty.
Prelims to Business Forecast Systems
In todayâs ever-evolving economic landscape, the role of business forecasting systems cannot be overstated. These systems serve as essential tools for organizations, allowing them to anticipate market trends, manage resources more effectively, and make informed strategic decisions. Forecasting is not just about predicting what lies ahead; itâs about understanding the dynamics that shape business environments and enabling companies to act accordingly.
Definition and Purpose
Business forecast systems encompass a variety of methodologies and approaches aimed at predicting future market conditions based on historical data and trend analysis. At their core, these systems focus on gathering and analyzing data from diverse sources to generate insights that guide strategic planning. This involves statistical techniques as well as models integrating expert opinions, allowing for a nuanced view of potential outcomes.
For instance, a company might use forecasting to predict sales for a new product based on previous launches, market research, and seasonal buying patterns. Such insights help in managing inventory, adjusting marketing strategies, and optimizing supply chains. In essence, the purpose of these systems is to minimize uncertainty, providing a structured framework for decision-making in an unpredictable world.
Importance in Modern Business
In the current fast-paced business environment, where change is the only constant, having a robust forecasting system is critical. Firms utilizing these systems can benefit in several significant ways:
- Enhanced Strategic Planning: With accurate forecasts, businesses can align their resources and strategies to meet anticipated demand, thus gaining a competitive edge.
- Operational Efficiency: By predicting future events, companies can streamline operations and reduce costs, especially in manufacturing, logistics, and inventory management.
- Risk Management: Effective forecasting aids in identifying potential risks and challenges in advance, allowing firms to devise mitigation strategies proactively.
- Resource Allocation: Understanding market trends can lead to better allocation of financial and human resources, ensuring that efforts are focused on areas with the highest potential returns.
"A good forecast is like a compass; it keeps you on the right path through the fog of uncertainty."
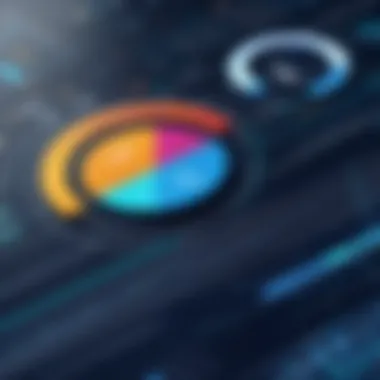
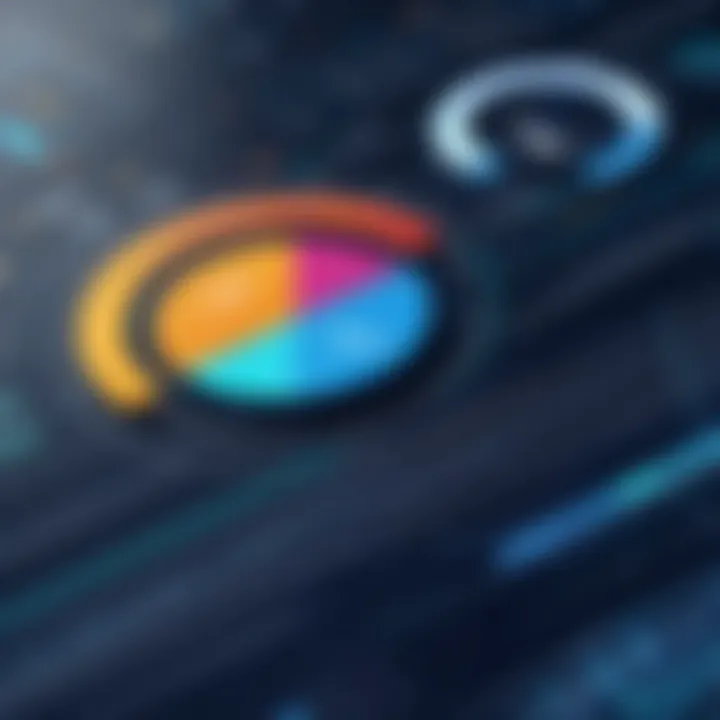
As we delve deeper into the various aspects of business forecasting throughout this article, it becomes evident that the integration of data-driven insights into decision-making is not merely beneficial, but necessary for sustained success. Without such systems, businesses may find themselves navigating without a map, reactive rather than proactive in the face of market dynamics.
Historical Context of Forecasting
Understanding how business forecasting has evolved over the years is essential for grasping its current methodologies and relevance in todayâs economy. The historical context not only sheds light on the changes in techniques and practices but also illustrates how the interplay between aspects like technology, economic conditions, and societal priorities shaped forecasting into what it is now. Factors such as these underline the strategies businesses adopt today and how past experiences inform future projections.
Evolution of Forecasting Techniques
Forecasting has undergone a notable metamorphosis from simple techniques employed in ancient civilizations to the sophisticated models used by corporations today. Initially, methods were predominantly qualitative, relying on human intuition and observation. Ancient traders, for example, would forecast the demand for goods by gauging previous sales and understanding seasonal trends. Fast forward to the 18th century; the Industrial Revolution marked a significant turning point, introducing data collection and statistical methods, making predictions slightly more reliable. The advent of the 20th century brought about the creation of formalized techniques such as time series analysis and economic indicators, positioning forecasting as a vital cog in strategic planning.
In more recent years, the focus has shifted to more complex forecasting methods. With techniques such as regression analysis and econometric modeling becoming standard practice, businesses improved their precision in predicting demand and supply. Furthermore, qualitative techniques have specialized and combined with quantitative methods to produce hybrid approaches, thus broadening the scope of analytical capabilities.
Influence of Technology on Forecasting
Technological advancements have dramatically influenced forecasting methodologies. In the age of rapid innovation, tools such as software for data analysis and processing have revolutionized the way businesses can now predict market behaviors. For instance, platforms like Tableau or Microsoft Power BI allow professionals to turn massive datasets into comprehensible visualizations, facilitating better decision-making.
The integration of Artificial Intelligence (AI) and Machine Learning (ML) is perhaps the most significant leap in forecasting technologies. With AI algorithms sifting through data faster and with more accuracy than humans, organizations can now uncover patterns and insights that may have previously eluded analysts.
"Today, the convergence of big data and AI not only enhances forecasting accuracy but also enables real-time adjustments to predictions, ensuring that businesses remain agile in a fast-paced market."
Alongside these technologies, the emergence of cloud computing has enabled businesses to store and access vast amounts of data seamlessly, further enhancing their forecasting capabilities. The amalgamation of these technological advancements not only allows for better accuracy but also provides businesses with greater agility â an essential trait in todayâs volatile environment.
In summary, the historical context of forecasting stands as a testament to the evolution from rudimentary techniques to the sophisticated systems in play today. As businesses navigate their strategic goals, understanding this journey can provide critical insights and underscore the importance of adapting methodologies as technology and market needs continue to evolve.
Types of Forecasting Methods
Understanding the various forecasting methods is crucial for organizations striving to navigate the murky waters of business planning. Different methods cater to unique needs and contexts, ensuring that businesses make informed decisions backed by the best possible insight. The effectiveness of forecasting can determine success or failure; thus, being well-versed in different methods enhances the forecasting accuracy. It ultimately aids in strategic resource allocation, risk assessment, and operational management.
Qualitative Forecasting
Qualitative forecasting leans heavily on expert opinion and intuition rather than hard data. This method is particularly useful in scenarios where historical data is sparse or unreliable, such as during the launch of a new product or when entering a new market. Expert panels, focus groups, and market surveys often serve as the backbone of qualitative analysis.
For instance, if a tech company is developing a next-gen smartwatch, it may gather insights from potential customers and industry experts to gauge interest and anticipated features. The value here lies in the subjectivity; the human insight captures nuances that numbers alone might miss. However, while subjective measures can provide deep insights, they can also be influenced by biases. Decision-makers must be cautious and consider complementing qualitative forecasts with quantitative data when available.
Quantitative Forecasting
Quantitative forecasting revolves around analyzing numerical data to make predictions. This method employs statistical techniques and algorithms to identify trends and patterns in historical data. Itâs an ideal approach for organizations that have a wealth of historical data at their disposalâretail chains, for example.
Some common techniques include:
- Time Series Analysis: This method assesses historical data to predict future values by identifying trends.
- Regression Analysis: By examining the relationship between variables, this method can forecast potential outcomes based on changes in independent variables.
Imagine a retail company reviewing past sales data to project future inventory needs. Using quantitative methods, they can analyze seasonal trends, consumer behavior, and other metrics to arrive at accurate forecasts. Yet, numbers have their limits. Outliers and unforeseen events can skew results, so itâs key to keep an eye on contextual factors that harvest data patterns.
Causal Forecasting
Causal forecasting, as the name suggests, looks for cause-and-effect relationships in data. This approach assumes that certain factors directly influence outcomes and seeks to quantify these influences. For example, a beverage company might examine the correlation between temperature changes and soda sales. They might find that higher temperatures spur increased demand for cold drinks during summer months.
Some methods employed in causal forecasting include:
- Econometric Models: These models combine economic theory with statistical techniques to reveal relationships among variables.
- Leading Indicators: Observing a set of indicators that typically precede economic performance can provide insights. For instance, an increase in consumer sentiment may forecast higher spending.
Implementing causal forecasting can deepen understanding of market dynamics but requires a sound methodology to validate these relationships. The selected causal factors must be logical and verified through rigorous analysis to avoid deriving misleading conclusions.
The Role of Data in Forecasting
When we talk about business forecast systems, one cannot overlook the significance of data. Ultimately, forecasts stem from data. It acts as the lifeblood of any accurate prediction. A well-structured approach to data utilization can make or break a forecasting effort. As businesses navigate a landscape filled with uncertainties, having reliable data is more than just a nice-to-have; it becomes a necessity.
Sources of Data
Data doesn't just appear out of thin air; it's harvested from various channels that can provide a wealth of information. Some of the primary sources include:
- Market Research: Companies often conduct surveys and focus groups to gauge consumer opinions. This grassroots insight is invaluable for accurate forecasting.
- Historical Sales Data: Analyzing past sales trends allows businesses to identify patterns that can guide future predictions. For instance, holiday seasons often see a spike in specific product categories.
- Economic Indicators: Broader economic data, such as GDP growth rates or unemployment statistics, can inform business forecasts. Knowing how these variables interact with consumer behavior helps refine predictions.
- Social Media: Platforms like Facebook and Twitter provide real-time data reflecting public sentiment. Businesses can pull trends from social discussions to anticipate market movements.
Just imagine if a toy manufacturer used social media analytics to gauge childrenâs interests. They could tailor their inventory to meet anticipated demand well ahead of the holiday rush.
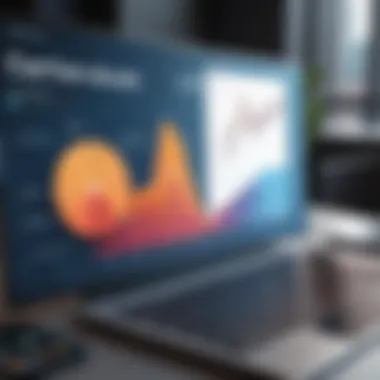
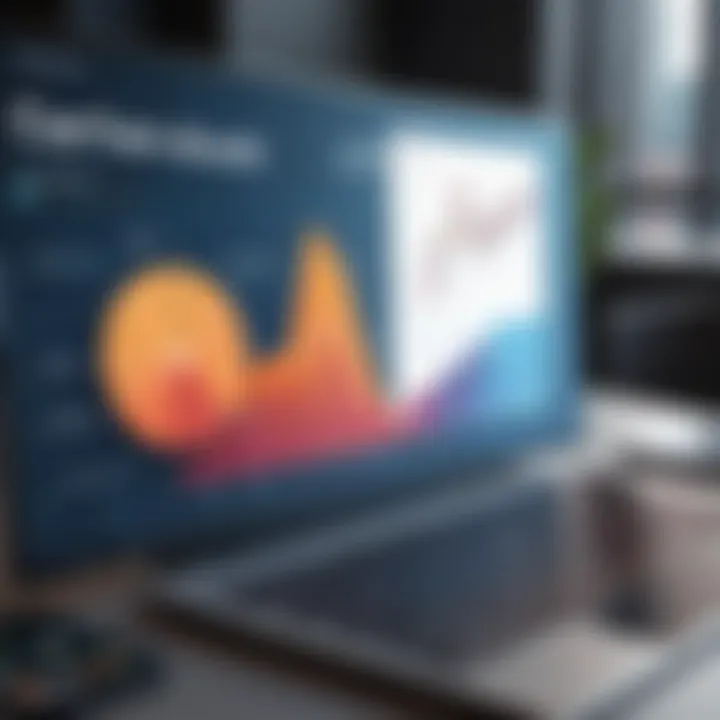
Data Quality and Integrity
While sourcing data is crucial, ensuring its quality and integrity is just as paramount. Poor data can lead to misguided forecasts that have repercussions across the organization. Consider these elements:
- Accuracy: The data must reflect the real-world scenario. Faulty figures can send businesses down the wrong path, resulting in inventory shortages or overstock situations.
- Timeliness: In a world that moves at breakneck speed, out-of-date data can quickly become irrelevant. Companies should prioritize accessing and analyzing the most current information available.
- Consistency: If data is inconsistent across various sources, it creates confusion. A multi-channel retail operation needs aligned data sets to create accurate forecasts.
- Completeness: Missing data can skew results. For instance, if customer feedback from one major retail outlet is absent, the overall picture might be compromised.
"You canât manage what you canât measure." This quote underscores the importance of high-quality data. Without it, forecasting becomes guesswork rather than an informed process.
The consequences of ignoring data quality are clear. A leading electronics retailer once suffered heavy losses because they misinterpreted customer return patterns, largely due to poor data integrity.
In summary, the heart of successful business forecasting lies in the sources and quality of data employed. Organizations that prioritize robust data systems will likely find themselves ahead in the forecasting game, navigating uncertainties with confidence.
Tools and Software for Business Forecasting
In the modern business landscape, the reliance on accurate forecasts cannot be overstated. Tools and software for business forecasting serve as the backbone of effective predictionsâoffering capabilities that transform raw data into actionable insights. As companies face an ever-increasing volume of information, selecting the right software becomes vital. These tools not only help in analyzing data trends but also enable organizations to remain agile in a dynamic marketplace.
Overview of Available Tools
When it comes to the world of forecasting tools, a multitude of options lay sprawled out. Each offering unique functionalities tailored to diverse needs in varied industries. Some notable examples include:
- Microsoft Excel: Though not specialized, its versatility makes it a staple in many organizations. Users can create customized models using its powerful functions.
- Tableau: This tool excels at visualizing data, allowing businesses to see patterns clearly. Users can integrate live data connections for real-time analysis.
- Oracle Crystal Ball: Specifically designed for forecasting, it uses simulation to help businesses make better choices based on risk analysis.
- IBM Planning Analytics: Merging AI with predictive analytics, this offers insights into trends and can help forecast outcomes through collaborative processes.
- SAP Integrated Business Planning: With real-time data processing, this tool is vital for organizations wanting to streamline supply chain operations along with forecast accuracy.
Each of these tools brings something to the table, and many organizations might find themselves exploring their synergies.
Criteria for Tool Selection
Choosing the right forecasting software can be akin to finding a needle in a haystack. It's crucial to lay out clear criteria to make the selection process smoother. Here are some pointers to consider:
- Ease of Use: Tools that are user-friendly can require less training time, allowing teams to get up and running swiftly.
- Integration: The ability of the tool to seamlessly integrate with existing systems holds value. It reduces disruptions and enhances workflow.
- Scalability: As a business grows, so does its forecasting needs. Selecting a tool that can scale accordingly is essential for long-term sustainability.
- Cost: Budget constraints always play major role. It's important to weigh the benefits against the costs, ensuring that the investment yields proper returns.
- Support and Community: A tool backed by an active support network or community can be lifesaving when challenges arise. Resources like forums or customer service can add significant value.
Challenges in Business Forecasting
Navigating the complexities of business forecasting comes with its fair share of hurdles. To effectively tailor forecasting systems, it is crucial to fully understand the challenges businesses face. In this section, we delve deeper into two major obstacles: uncertainty and assumptions, as well as resistance to forecasting practices. Recognizing these issues not only allows organizations to address them strategically but also aids in refining their forecasting methodologies for better outcomes.
Uncertainty and Assumptions
Uncertainty can be a thorn in the side of even the best-planned forecasts. The future, by its nature, remains unpredictable and is influenced by countless factors that can disrupt even the most carefully constructed models. For instance, let's say a business relies heavily on customer behavior trends to project future sales. If a sudden economic decline occurs, or a competitive threat emergesâwhich can be as unexpected as a summer thunderstormâit can throw those predictions into disarray.
The foundations of forecasting often rest on certain assumptions. These might involve factors such as market stability, customer loyalty, or even technological reliability. However, these assumptions can sometimes lead to blind spots. Businesses that become overly reliant on consistent historical data may fail to anticipate changes driven by shifting demographics or unexpected innovations. For example, consider how quickly online shopping evolved during the pandemic, fundamentally altering consumer habits. Relying strictly on historical sales data would hardly equip a business to handle that shift.
In essence, acknowledging uncertainty and examining assumptions is not just an academic exercise; itâs vital for creating robust forecasting systems that can weather the unpredictable storms ahead. Incorporating scenario planningâa strategy that considers multiple future outcomesâcan be a way to confront this uncertainty head-on and prepare for varying possibilities.
Resistance to Forecasting Practices
Despite the proven advantages that effective forecasting can yield, some organizations are hesitant, even resistant, to adopt such practices. This reluctance can stem from a few sources. First, there may be a lack of trust in the forecasting process itself. Stakeholders may question the reliability of the models used and the accuracy of the data fed into them. As the saying goes, "Trust but verify!" In the case of forecasting, if the underlying data sources are deemed questionable, the entire forecast could lose credibility.
Moreover, the culture within an organization plays a critical role. Some companies may have a legacy mentality that dismisses quantitative analysis in favor of intuition-based decision-making. This can create friction when trying to integrate new forecasting tools or methodologies. Educating teams about the benefits and tangible successes of forecasting practices can bridge this gap.
To combat this resistance, it is essential to demonstrate the value of accurate forecasting. Sharing case studies of successful implementations can showcase how other businesses have transformed their operations through effective forecasting. Highlighting not just the results but also the learnings gleaned from these experiences can slowly chip away at the reluctance.
In summary, while uncertainty and resistance pose significant challenges in business forecasting, understanding these elements enables organizations to sharpen their foresight strategies. This, in turn, fortifies overall business resilience in a competitive landscape.
Implementing a Business Forecast System
Implementing a business forecast system holds significant weight in ensuring a company's strategic decisions are well-informed. It essentially lays the groundwork for anticipating market fluctuations, consumer behavior, and internal performance metrics. Without a robust system, businesses might find themselves navigating through murky waters, guessing what lies ahead instead of preparing for it. This is where precision in forecasting transcends mere guesswork; it becomes a strategic advantage.
A tailored business forecast system can enhance agility. Imagine a company that predicts rising demand for a product; it can optimize inventory levels, thereby reducing storage costs and increasing sales potential. Moreover, by assessing forecasting processes, decision-makers can align resources more effectively, thus carving a space for innovation even within tight budgets.
Additionally, a comprehensive forecast system takes away a lot of the uncertainty involved in planning. This boosts confidence in both leadership and teams. When everyoneâs on the same page, it breeds synergistic efforts towards common goals. However, this isn't merely about choosing a forecasting method; it requires a nurturing environment where data sharing is routine and encouraged across departments.
Indeed, there are challenges in implementation, such as resistance to change. However, addressing these concerns constructively can yield a culture that embraces data-driven decisions.
Steps to Implementation
When it comes to implementing a business forecast system, one must tread carefully but with purpose. Hereâs a closer look at the steps involved:
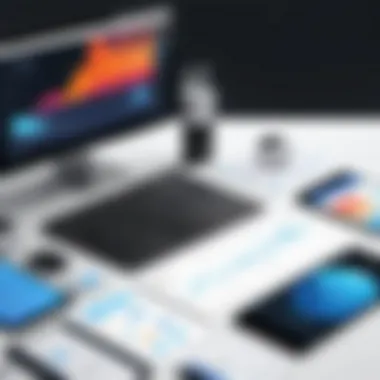
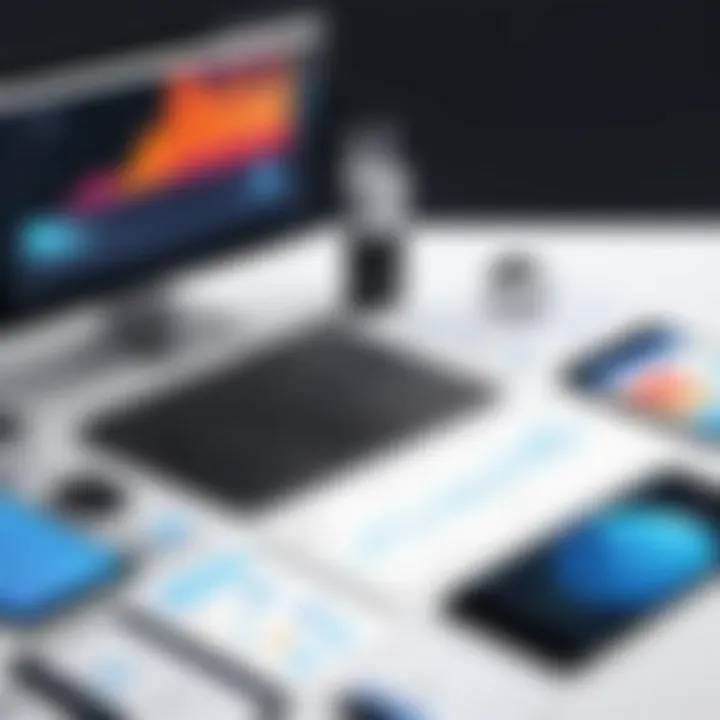
- Define Clear Objectives: Begin by identifying the specific goals you aim to achieve with your forecast system. Are you predicting sales growth, market trends, or resource needs? Referential clarity can guide future steps.
- Choose the Right Methodology: Depending on your objectives, select an appropriate forecasting method. It could be qualitative, quantitative, or a causal approach, so know your audience and their expectations.
- Gather Relevant Data: Data serves as the backbone of any forecasting system. Collecting high-quality data from internal and external sources is essential.
- Select Appropriate Tools: Utilize software that complements your methodology and aligns with your business's scale. Tools like Tableau or Microsoft Excel can be effective, depending on complexity.
- Develop a Prototype: Before full-scale implementation, pilot your forecasting system. This helps identify potential pitfalls while allowing tweaks to the approach without going overboard.
- Train Your Team: Investing in training is paramount. If team members are well-versed in the system, they can utilize it effectively, thereby achieving the desired outcomes faster.
- Continuous Evaluation: Post-implementation, keep a keen eye on the performance of the forecasting system. Regularly review and adjust methodologies as market conditions evolve.
Integration with Existing Systems
The integration of a business forecast system with existing corporate structures can be a daunting task, yet it carries significant advantages. First and foremost, bringing new tools into the fold requires deep synchronization with current processes, which can facilitate a smoother transition without disrupting ongoing operations.
To achieve seamless integration, businesses should:
- Assess Current Systems: Conduct a thorough analysis of existing systems. What tools or software are in place? Identifying the gaps will help bridge new functionality effectively.
- Prioritize Compatibility: Not all tools play well with one another. Opt for software solutions that can easily connect with current databases and resource management systems.
- Employee Involvement: Engage staff from various departments early on. This collective input can surface hidden issues and lead to a more holistic integration strategy.
- Establish Clear Protocols: Define and document new procedures for using the forecasting system alongside existing platforms. This can safeguard against confusion and ensure clarity in operations.
In summary, successfully implementing and integrating a business forecast system isnât just a technical task; it's about fostering a collective vision that values data and prepares the company for its future endeavors.
Case Studies of Successful Forecasting
Understanding real-world applications of forecasting can offer invaluable insights for businesses. Case studies reveal how theoretical concepts play out in practice under the pressures of market dynamics. Companies that have implemented successful forecasting systems often showcase the sheer power of precise planning and proactive strategy.
Industry-Specific Applications
Different sectors face unique challenges and thus tailor their forecasting methods accordingly. Here are a few examples:
- Retail
For retail giants like Walmart, forecasting isn't just a number game; it's a cornerstone of their operational efficiency. By analyzing past sales data and external factors like weather patterns or holiday seasons, Walmart is able to manage inventory levels better, ensuring that shelves stay stocked but not overfilled. Such agility allows them to respond quickly to shifting consumer behaviors, translating into increased sales and customer satisfaction. - Healthcare
In healthcare, precision can be a matter of life and death. Organizations like the Mayo Clinic utilize forecasting models to predict patient admissions based on factors like seasonal illnesses, socioeconomic trends, and local demographics. By anticipating these trends, they manage their resources more effectively, ensuring that patient care remains exceptional even during peak times. - Manufacturing
Caterpillar, the heavy machinery manufacturer, relies heavily on forecasting to streamline production. By assessing demand forecasts coupled with economic indicators, Caterpillar adjusts its manufacturing schedules. This ensures they're not overproducing during downturns, thereby maximizing profits while minimizing waste. - Technology
In the fast-paced world of technology, companies like Apple utilize forecasting to predict product demand based on historical trends and market research. With limited product launches each year, accurate forecasts help them scale production without compromising quality, leading to significant savings and increased customer trust.
These examples illustrate that no matter the industry, tailor-fit forecasting applications can unlock immense potential.
Key Takeaways from Case Studies
Taking a close look at these case studies, certain themes emerge that underline the successes:
- Data-Driven Decisions: Companies that invest in robust data collection and analysis often yield dividends. The integration of historical data, market research, and even customer feedback offers a rich tapestry of insights.
- Agility and Adaptation: Organizations that remain flexible in their forecasting approachâaltering models as new data surfacesâstay ahead of the curve. This adaptability becomes crucial during unforeseen economic shifts or disruptions.
- Technology as a Catalyst: Leveraging technology like AI and machine learning not only enhances forecasting accuracy but also fosters a culture of innovation within businesses, paving the way for smarter operations.
It's clear that studying real-life examples of successful forecasting provides a treasure trove of insights, demonstrating that accuracy can drive results, enhance efficiencies, and shape strategic decisions. In a world where just "flying by the seat of your pants" doesnât cut it, these case studies equip professionals with the tools to think strategically.
Future Trends in Business Forecasting
As we coast further into the 21st century, the landscape of business forecasting undergoes substantial transformation. The shift is driven primarily by advancements in technology, the exponential growth of data, and the need for companies to remain agile and competitive. The relevance of this section in our discussion lies not only in understanding what the future holds but also in grasping the strategic advantages that these emerging trends may provide.
Artificial Intelligence and Machine Learning
Artificial Intelligence (AI) and Machine Learning (ML) are rapidly becoming the beating heart of forecasting methodologies. With their ability to analyze vast quantities of historical data and recognize patterns at lightning speed, these technologies bring a sharp edge to predictive analytics. For instance, the retail giant Walmart employs machine learning algorithms to predict customer purchasing behavior, optimizing inventory and supply chain management accordingly.
- Enhanced Predictive Accuracy: AI and ML can process data from diverse sources such as social media, economic indicators, and weather patterns, creating more accurate and nuanced forecasts.
- Automated Insights: These technologies reduce the need for manual interventions, allowing businesses to focus on strategizing rather than sifting through numbers.
- Real-Time Adjustments: Unlike traditional methods, AI-driven systems can adapt forecasts dynamically as new data pours in, providing timely insights that are crucial for operational adjustments.
If businesses can harness AI effectively, they can not just keep up with trends but also set them, outpacing competitors who rely solely on conventional forecasting methods.
The Increasing Role of Big Data
Big Data refers to the vast volumes of varied data generated every second. Its increasing role in business forecasting cannot be overstated. Companies now have access to a treasure trove of information, and how they utilize it can mean the difference between success and failure. The shift towards data-driven decision-making reflects a broader trend in which intuition is giving way to evidence-based strategies.
- Diverse Data Sources: Businesses are tapping into datasets from customer interactions, economic segments, and even sensor data from IoT devices to enrich their forecasting models.
- Data Integration: The ability to integrate disparate data silos enables companies to create a holistic view of trends and behaviors, leading to better-informed decisions.
- Predictive Models: With advanced analytics, companies can not only forecast future trends but also simulate various scenarios, optimizing strategies for numerous possible outcomes.
"In the age of information, allowing data to inform decisions is no longer a luxury; itâs a necessity for sustainable growth."
The End
Wrapping it all up, the conclusion is like the cherry on top of a well-made sundae of knowledge. This article has delved deep into the nuances of business forecast systems, highlighting their crucial role in strategic decision-making. For professionals and students alike, understanding forecasting isnât just a nice-to-have; it's downright essential in todayâs rapidly shifting business landscape.
Summary of Key Insights
Weâve touched on a variety of points throughout the discourse:
- Integration of Technology: The influence of technology, particularly artificial intelligence and big data, has been pivotal in refining forecasting methods. Machine learning algorithms can process vast amounts of data, identifying patterns that would be impossible for humans to discern alone.
- Importance of Data Quality: High-quality data is the backbone of any forecasting effort. Without solid information, forecasts are about as useful as a chocolate teapot.
- Challenges: Every system has its hurdles to clear. The unspoken resistance to adopting forecasting practices can hinder potential gains, emphasizing the necessity for change management strategies.
- Practical Applications: Real-world case studies underscore the effectiveness of tailored forecasting methods across various sectors. For example, the retail industry utilizes sophisticated forecasting to manage inventory and predict consumer demand, showcasing how pertinent methods can directly impact revenue.
This summary brings to the forefront the major takeaways that are crucial for harnessing the power of forecasting in business contexts.
Final Thoughts on Business Forecasting
Stepping back, itâs clear that business forecasting is not merely an academic exercise; it's a critical practice that can shape the very core of a businessâs success. Companies that embrace and implement effective forecasting methods often find themselves ahead of the curve, capable of adapting to market demands with agility. However, it requires a mindset shift. Businesses should view forecasting as a dynamic, ongoing process rather than a one-off project.
Furthermore, the understanding of qualitative and quantitative methods, combined with the right tools, can create a robust forecasting framework. Organizations need to cultivate a culture that encourages data-driven decision-making while being open to reevaluating their approaches as new technologies emerge.
In essence, stepping into the realm of business forecasting is akin to taking a leap into a treasure trove of insights, ideals, and improvements. For software developers and IT professionals, mastering these systems suggests navigating an ever-evolving digital landscape, separating the wheat from the chaff, and ultimately driving organizational success. As we look ahead, the future is ripe with possibilities, inviting proactive engagement in the ever-intriguing world of forecasting.