Exploring Predictive Budgeting: An In-Depth Overview

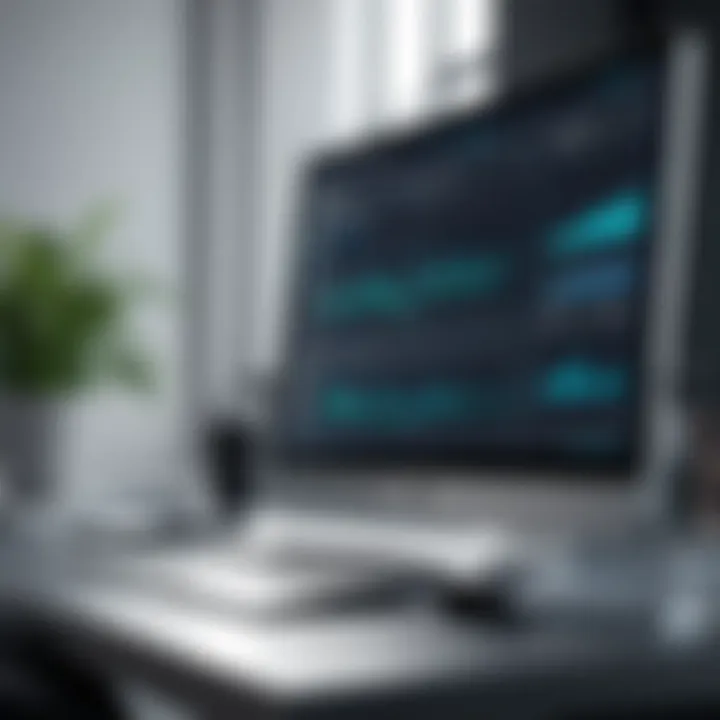
Intro
Predictive budgeting represents a paradigm shift in the way organizations approach financial planning. This method harnesses advanced analytical techniques and technology to forecast future budgets based on historic data and trends. Unlike traditional budgeting methods that rely heavily on past performance and static assumptions, predictive budgeting merges statistical modeling with real-time data to refine financial projections continuously. Its adoption is particularly significant in today's fast-paced business environment, as it equips firms to make more informed decisions.
The relevance of predictive budgeting extends across various sectors. Businesses, non-profits, and even government entities have begun incorporating advanced technological tools into their budgeting processes. As this trend grows, understanding the underlying methodologies, advantages, and challenges has become essential for professionals and scholars alike.
In this article, we will explore the methodologies employed in predictive budgeting, its applications, and its role in enhancing decision-making. We will also assess the integration of technology, particularly data analytics, which is crucial for maximizing budgeting accuracy. By examining the potential challenges associated with predictive budgeting, we aim to deliver a balanced perspective on this emerging financial practice.
Overview of Software
As predictive budgeting gains traction, several software solutions have emerged to facilitate its implementation. These software options are pivotal in streamlining the budgeting process and ensuring accuracy through real-time data analysis.
Purpose and Use Cases
Predictive budgeting software serves multiple purposes, including:
- Forecasting Financial Performance: Using historical data to predict future financial outcomes.
- Resource Allocation: Helping organizations allocate resources effectively based on dynamic forecasts.
- Scenario Planning: Allowing users to simulate different budgeting scenarios, assessing their impacts on financial health.
These use cases are not just limited to corporations. Non-profits can manage funding more efficiently, while governmental bodies can better predict expenditure requirements.
Key Features
When evaluating predictive budgeting software, certain features are crucial:
- Data Integration: Ability to pull data from various sources, ensuring comprehensive analysis.
- Analytics Tools: Providing insights through advanced analytics, including statistical models and machine learning.
- Collaboration Features: Enabling team members to collaborate on budget discussions in real time.
- User-Friendly Interface: Important for quick adoption among team members.
In-Depth Review
To understand predictive budgeting software thoroughly, we must analyze its performance and user experience in detail.
Performance Analysis
Performance is vital in predictive budgeting software. This includes:
- Accuracy: The precision of financial forecasts based on historical data.
- Scalability: The software's ability to handle growing datasets and user demands.
- Speed: Efficiency in processing data and generating reports.
Organizations often measure software performance through these metrics, seeking options that consistently deliver reliable outputs.
User Interface and Experience
A quality user interface enhances user experience significantly. Key aspects include:
- Navigation Simplicity: Ensuring that users can easily locate features and data inputs.
- Visualizations: Offering clear graphical representations of data for better comprehension.
- Feedback Mechanisms: Providing users with immediate input about their actions within the software.
By focusing on these elements, firms can ensure that their teams can adopt predictive budgeting practices smoothly, leading to informed and timely decisions.
The timely integration of technology and data analytics into budgeting processes signifies a shift towards more agile financial planning.
In summary, predictive budgeting stands on the brink of transforming financial landscapes. Its blend of cutting-edge software and innovative methodologies promises to equip organizations with the tools necessary for strategic growth. Understanding this evolution is vital for professionals navigating this complex and changing environment.
Prelude to Predictive Budgeting
Predictive budgeting has emerged as a crucial tool in the financial planning landscape, particularly due to its ability to provide a nuanced approach to resource allocation. Traditional budgeting often relies on historical data and fixed budgets, limiting flexibility and responsiveness. In contrast, predictive budgeting allows organizations to adapt to changing circumstances by leveraging data analytics and forecasting techniques. This ability to anticipate future financial scenarios positions predictive budgeting as an indispensable strategy in contemporary finance. By utilizing predictive budgeting, firms can enhance their decision-making processes and improve financial performance.
Defining Predictive Budgeting
Predictive budgeting can be defined as the process of using data analysis to generate forecasts for future financial performance. This involves assessing trends, identifying patterns, and utilizing statistical models to project outcomes. The methodology integrates various sources of data, including quantitative metrics and qualitative insights. The goal is to create a budgeting framework that is proactive rather than reactive. In essence, predictive budgeting helps organizations to think ahead, aligning their strategies with projected financial realities.
The application of predictive algorithms and tools is essential to this process. It considers various economic variables and organizational metrics. This approach enables businesses to understand potential financial trajectories and allocate resources accordingly.
A Brief History of Budgeting Approaches
A historical perspective on budgeting illustrates the evolution of financial planning strategies. Traditional budgeting methods have roots in the early 20th century, pivoting mainly around incremental budgeting practices. Incremental budgeting involves adjusting previous budgets based on the prior year's performance. However, this historical method often leads to inefficiencies, as it may not account for shifting market conditions or organizational changes.
In response to these shortcomings, newer approaches like zero-based budgeting emerged. This method requires organizations to justify every expense for each new period, promoting a more rigorous analysis of spending. Despite its effectiveness, zero-based budgeting can be time-consuming and complex.
As businesses began to embrace technology and data analytics, predictive budgeting came into prominence. This approach reflects a broader trend towards data-driven decision-making, offering organizations the flexibility to adapt and thrive in dynamic environments. Predictive budgeting represents a significant shift from static methods, aligning closely with modern technological advancements.
Core Principles of Predictive Budgeting
Predictive budgeting stands out as a crucial methodology in modern financial planning due to its focus on data and analysis. It serves as a framework that empowers organizations to adapt and thrive in an environment characterized by uncertainty. By integrating predictive analytics into the budgeting process, organizations can better anticipate future needs based on historical data and current trends. This foresight not only enhances accuracy but also promotes strategic thinking among financial teams.
Data-Driven Decision Making
Data-driven decision making is the cornerstone of predictive budgeting. This principle emphasizes the significance of utilizing quantitative data to inform budgeting decisions. Organizations collect and analyze vast amounts of data, transforming raw numbers into insights that guide their financial plans. Access to accurate historical data and real-time analytics helps decision-makers identify patterns, trends, and potential risks. This approach enables a proactive stance in financial planning, where decisions are based on solid evidence rather than assumptions or guesswork. Practitioners who embrace data-driven methods are more likely to allocate resources efficiently and optimize their overall financial strategy.
Forecasting Techniques
Forecasting techniques represent another essential principle of predictive budgeting. These encompass various methodologies that help anticipate future financial scenarios. Techniques such as time series analysis, regression analysis, and machine learning provide valuable tools for finance professionals. Each method has its strengths and can be selected based on the specific context. For example, time series analysis might be ideal for identifying seasonal trends, while regression analysis can reveal relationships between different financial variables. By leveraging these forecasting techniques, organizations can generate more accurate budget projections.
Scenario Planning
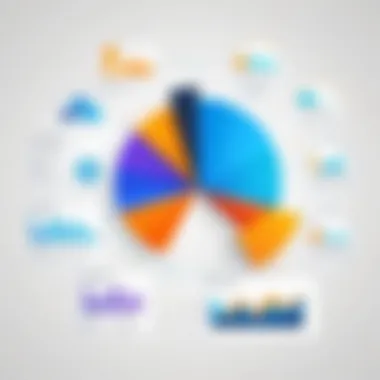
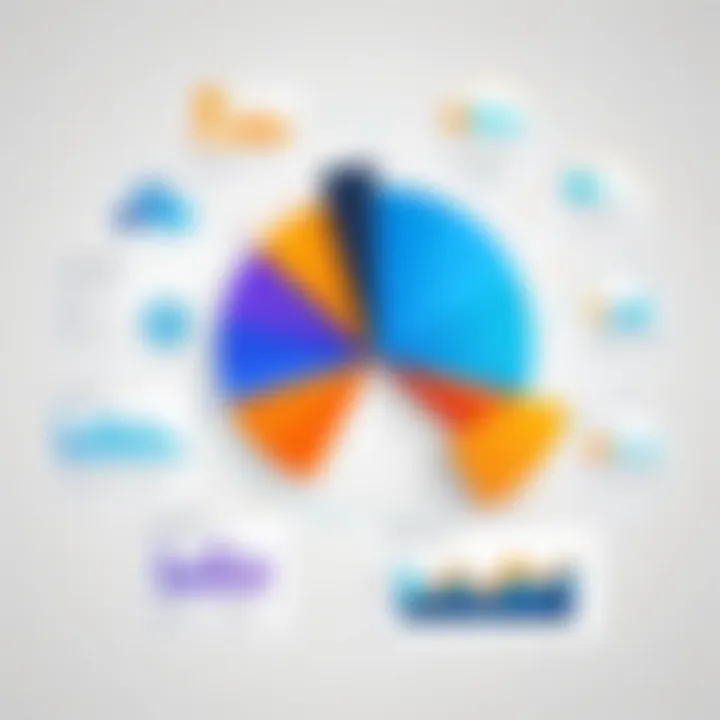
Scenario planning adds depth to predictive budgeting by allowing organizations to develop different financial outcomes based on varying assumptions. This method involves creating several plausible future scenarios that consider factors such as economic changes, technological advancements, or shifts in consumer behavior. By evaluating the potential impact of each scenario on the budget, organizations can prepare for a range of possible futures. This flexibility is vital in an era of rapid change, enabling firms to respond effectively to unforeseen challenges. As organizations engage in scenario planning, they gain a clearer understanding of potential risks and opportunities, which ultimately supports more resilient financial planning.
Comparative Analysis: Predictive vs. Traditional Budgeting
In the realm of financial planning, understanding the differences between predictive budgeting and traditional budgeting is crucial. This comparative analysis will highlight the methodologies, advantages, and challenges associated with both approaches. By examining these elements, organizations can make informed decisions that align with their unique financial objectives.
Key Differences
Predictive budgeting and traditional budgeting differ significantly in various aspects:
- Approach to Planning: Traditional budgeting often relies on historical data and fixed allocations, whereas predictive budgeting uses real-time data and forecasts to create dynamic financial plans.
- Flexibility: Predictive budgeting is inherently more adaptable. Organizations can adjust their financial strategies based on changing market conditions or operational needs. In contrast, traditional budgeting tends to be rigid, often locking organizations into predetermined budgets for the year.
- Focus on Future Outcomes: Predictive budgeting emphasizes forward-thinking. It does not just analyze past performance; rather, it projects future trends based on collected data. Traditional budgeting focuses more heavily on historical trends and fixed expenditure categories.
- Ease of Adjustment: In a predictive budgeting framework, adjustments can be made easily as new data emerges. Traditional budgets may require significant effort and time to amend once they are set.
Understanding the key differences allows businesses to choose a budgeting approach that is best suited to their objectives and needs.
Strengths and Weaknesses
Both predictive and traditional budgeting come with their own strengths and weaknesses, which are important to understand when deciding which approach fits a particular organization.
Strengths of Predictive Budgeting:
- Enhanced Decision-Making: Predictive budgeting provides insights that help decision-makers anticipate future needs and allocate resources accordingly.
- Resource Optimization: By relying on data analytics and forecasting, organizations can identify areas for cost savings and potential investment.
- Improved Agility: Companies can respond quickly to market fluctuations and operational changes, ensuring that financial plans remain relevant.
Weaknesses of Predictive Budgeting:
- Data Quality Dependence: The accuracy of predictive budgeting relies heavily on the quality of the data used. Poor data can lead to flawed projections.
- Resistance to Change: Transitioning from traditional budgeting to predictive methods can face challenges, especially in organizations accustomed to established practices.
Strengths of Traditional Budgeting:
- Simplicity: Traditional budgets are straightforward and easy to implement, making them suitable for smaller organizations or those with limited financial complexities.
- Control Mechanism: It provides a controlled spending framework, allowing organizations to maintain a clear oversight of their financial resources.
Weaknesses of Traditional Budgeting:
- Inflexibility: With a static budget, any unexpected changes can create significant challenges.
- Limited Insights: Traditional methods can overlook emerging trends and opportunities because they focus primarily on past performance.
This comparative analysis underscores the need for organizations to carefully evaluate their budgeting strategies, taking into consideration their unique context and the distinct advantages and disadvantages presented by both approaches.
"Understanding the nuances of predictive and traditional budgeting is essential for optimizing financial strategy."
By thoroughly assessing these factors, firms can better navigate the complexities of financial planning and choose a path that aligns with their long-term goals.
Applications of Predictive Budgeting
Predictive budgeting has become a crucial practice for organizations aiming to refine their financial planning. It is not limited to a single field. Its applications span across various sectors including corporate finance, public institutions, and nonprofit entities. Each sector employs predictive budgeting to enhance their efficiency and effectiveness in resource allocation.
The benefits of predictive budgeting include improved financial forecasting accuracy, the ability to adapt to market changes, and better risk management. These factors provide organizations with a competitive edge in an increasingly data-driven world. Understanding how predictive budgeting functions in different sectors can help professionals adopt best practices that align with their specific goals.
Corporate Finance
In corporate finance, predictive budgeting allows organizations to plan for growth while managing resource constraints. This method enables finance teams to analyze past performance metrics and forecast future revenues. By leveraging historical data and advanced analytics, companies can establish realistic budgets that reflect projected economic conditions.
Key Advantages:
- Informed Decision Making: Companies can make strategic choices based on accurate forecasts.
- Risk Management: Identifying potential financial risks becomes easier through scenario analysis.
- Resource Allocation: Predictive models guide organizations in strategically allocating funds, ensuring priority projects receive the necessary resources.
Ultimately, predictive budgeting transforms the traditional budgeting process into a more dynamic and responsive financial strategy, aiding in fulfilling corporate objectives.
Public Sector Budgeting
In the public sector, predictive budgeting plays a pivotal role in enhancing transparency and accountability. Governments, from local to national levels, use this approach to estimate future expenses and consider potential budgetary gaps. This practice is vital for ensuring that public funds are used efficiently.
Key Considerations:
- Public Accountability: With the ability to anticipate funding needs, public officials can be answerable to citizens regarding resource allocations.
- Service Optimization: Predictive budgeting helps in planning public services, ensuring that essential programs receive financial backing as needed.
- Policy Formulation: This method generates data that can guide policy initiatives and funding priorities.
Employing predictive budgeting in this context fosters a more informed approach to managing public finances.
Nonprofit Organizations
Nonprofit organizations face unique budgeting challenges because they often rely heavily on donations and grants. Predictive budgeting can address these challenges by providing insights on funding trends and enabling nonprofits to adjust their operations based on financial forecasts.
Benefits for Nonprofits:
- Sustainability: Understanding anticipated funding allows nonprofits to plan sustainable projects rather than relying on short-term funding.
- Enhanced Fundraising Efforts: Predictive insights can optimize fundraising strategies, allowing organizations to target potential donors effectively.
- Performance Evaluation: By comparing predictions with actual outcomes, nonprofits can evaluate the effectiveness of their programs, informing future strategies.
Incorporating predictive budgeting helps nonprofits maximize their impact while ensuring they remain financially viable in fluctuating economic conditions.
"Predictive budgeting is not merely a financial exercise; it is a strategic approach to managing resources that can transform how organizations prepare for the future."
Role of Technology in Predictive Budgeting
The integration of technology into predictive budgeting is increasingly vital in today's data-centric landscape. Organizations are leveraging advanced tools and methodologies that enable more accurate forecasting and efficient resource allocation. Technology greatly enhances the capacity to analyze vast amounts of data, leading to informed decision-making. The focus here includes perspectives on specific technology elements, benefits, and thoughtful considerations surrounding their role in predictive budgeting.
Data Analytics Tools
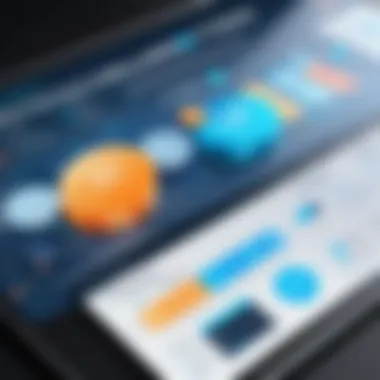
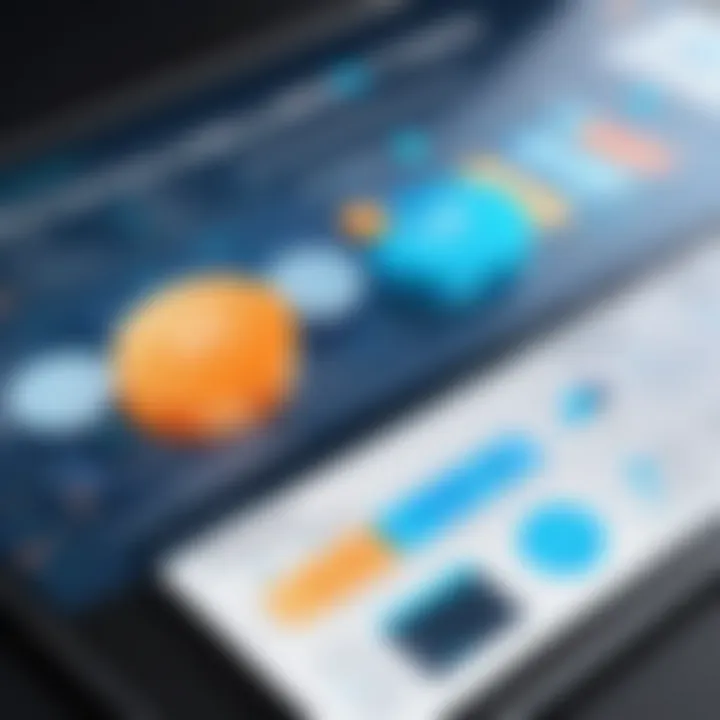
Data analytics tools are critical in processing and interpreting financial data. These tools allow for the collection of insights from historical data, which can be fundamental in identifying trends and patterns. Common tools in the field include Tableau, Microsoft Power BI, and Google Analytics. They help finance departments visualize complex data sets, making it easier to communicate findings to stakeholders.
- Advantages of Data Analytics Tools:
- Improved Accuracy: Automated tools minimize human error, providing more reliable outputs.
- Timely Insights: Real-time data analysis supports quick adjustments based on current economic conditions.
- Customized Reporting: Users can tailor dashboards to display relevant metrics that align with organizational goals.
In essence, data analytics tools set a foundation for successful predictive budgeting strategies by fostering greater clarity and control over financial data.
Integration with Financial Systems
The successful application of predictive budgeting requires seamless integration with existing financial systems. A unified system ensures that data flows easily between budgeting, forecasting, and actual financial performance. Tools like Oracle NetSuite and SAP Business One exemplify sophisticated ERP solutions that facilitate this integration.
Key Benefits of Integration:
- Consistency: Having a single source of truth reduces discrepancies and enhances the reliability of financial data.
- Efficiency: Automated data transfers eliminate redundant manual processes, saving time and resources.
- Scalability: Organizations can adapt their budgeting processes as they grow, ensuring continued relevance to their financial strategies.
These integrations play a pivotal role in allowing predictive budgeting to evolve with organizational needs.
Artificial Intelligence and Machine Learning
Artificial intelligence (AI) and machine learning (ML) provide a new frontier in predictive budgeting. These technologies can analyze historical financial data and predict future trends more accurately than traditional methods. AI can learn from new data inputs, continually refining its predictions based on the ever-changing market conditions.
- Benefits of Utilizing AI and ML:
- Predictive Accuracy: Advanced algorithms can identify complex patterns not easily seen by human analysts.
- Automation: Repetitive tasks such as data entry and basic analysis can be automated, freeing financial professionals for higher-level tasks.
- Risk Management: AI can forecast potential financial risks, allowing organizations to proactively manage their budgets.
"The integration of AI and ML in predictive budgeting processes marks a significant shift in how organizations approach financial planning."
Challenges in Predictive Budgeting
The concept of predictive budgeting carries numerous advantages, but it also comes with significant challenges. Understanding these obstacles is crucial for effective implementation and maximizing the potential benefits. The challenges can undermine the effectiveness of predictive budgets, leading to inaccuracies and misinformed decisions. Addressing these issues requires a strategic approach, as the consequences can extend beyond just financial implications.
Data Quality Issues
One of the fundamental challenges in predictive budgeting is ensuring the quality of data used in the budgeting process. Poor data quality can result in misleading forecasts and unreliable financial models. If the data is incomplete, outdated, or inaccurate, the analysis will lead to flawed conclusions. Organizations need to establish robust data management practices to ensure data integrity.
Key Aspects to Consider:
- Data Sources: Understanding where data is coming from and vetting its reliability is essential. Utilizing multiple sources can enhance accuracy.
- Cleaning Data: Regular data cleaning is necessary to remove duplicates, correct errors, and fill in gaps.
- Monitoring Changes: Continuous monitoring of data changes is vital, as it can drastically impact predictive modeling.
Resistance to Change
Organizations often face resistance to adopting predictive budgeting methods, primarily due to cultural issues. Employees may find it challenging to shift from traditional budgeting methods to more dynamic approaches. This resistance can stem from a lack of understanding about the benefits of predictive budgeting or fear of unsuitability.
Strategies to Overcome Resistance:
- Education and Training: Providing comprehensive training can help staff understand predictive budgeting advantages.
- Transparent Communication: Clearly articulating why changes are necessary can reduce anxiety and build consensus.
- Pilot Programs: Initiating small-scale pilot projects can demonstrate the effectiveness of predictive budgeting without demanding large-scale changes initially.
Complexity in Implementation
Implementing predictive budgeting systems can be complex. The process requires various components, such as technological investments and skilled personnel. Organizations must navigate these challenges, ensuring the adoption of suitable tools and strategies.
Challenges to Implementation:
- Integration with Existing Systems: Aligning new budgeting tools with current financial systems can be cumbersome and requires careful planning.
- Data Skills Gap: Professionals need specific skills to analyze data effectively. The lack of such skills can narrow the utility of predictive budgeting.
- Resource Allocation: Ensuring enough resources, both financial and human, is typically a balancing act that requires ongoing attention.
Effective management of these challenges is essential to harnessing the true potential of predictive budgeting. Without overcoming these hurdles, organizations may struggle to fully reap the benefits of this innovative approach.
Best Practices for Implementing Predictive Budgeting
Implementing predictive budgeting effectively can significantly enhance financial planning processes in various sectors. Best practices guide organizations in establishing a robust predictive budgeting framework, thereby increasing accuracy and improving decision-making. In this section, we will explore critical elements that contribute to successful implementation.
Establishing a Baseline
Establishing a baseline is crucial for predictive budgeting. A baseline provides a point of reference from which future forecasts can be evaluated. This reference could be historical data reflecting past trends and performance. To set a solid foundation, organizations should:
- Collect Historical Data: Gather relevant financial records and operational data from previous years. These records help understand patterns and variances.
- Adjust for Anomalies: Review data for one-time events that may skew results, such as unusual expenses or income spikes. Adjusting for these factors refines future predictions.
- Define Clear Metrics: Establish specific metrics that align with business goals. These indicators include revenue targets, operational costs, and other key performance indicators.
A firm foundation based on accurate historical data allows organizations to produce reliable forecasts, paving the way for effective future planning.
Continuous Data Monitoring
Continuous data monitoring is an essential practice in predictive budgeting. The financial landscape is constantly changing, and ongoing assessment ensures adaptability. Key practices involved include:
- Real-Time Data Analytics: Utilize data analytics tools that enable real-time analysis of financial metrics. Regular monitoring helps identify trends promptly and allows for swift adjustments.
- Feedback Loops: Create mechanisms for collecting feedback on budgeting performance. These insights help refine future forecasts and strategies.
- Adjust Budgets Regularly: Instead of sticking to an annual budget, organizations should revise budgets periodically. Such flexibility fosters a dynamic environment that responds to market changes.
Effective continuous monitoring of data ensures that the predictive budgeting process remains relevant and accurate.
Stakeholder Involvement
Engaging stakeholders is vital for successful predictive budgeting implementation. Stakeholders include department heads, financial analysts, and other key personnel. Their involvement can enhance buy-in and improve the accuracy of forecasts. Consider the following strategies:
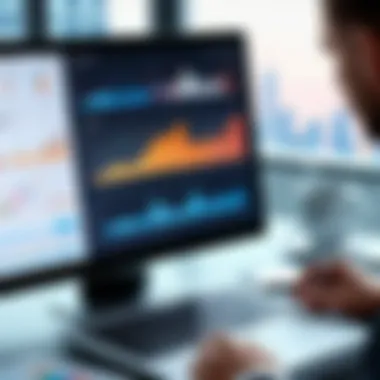
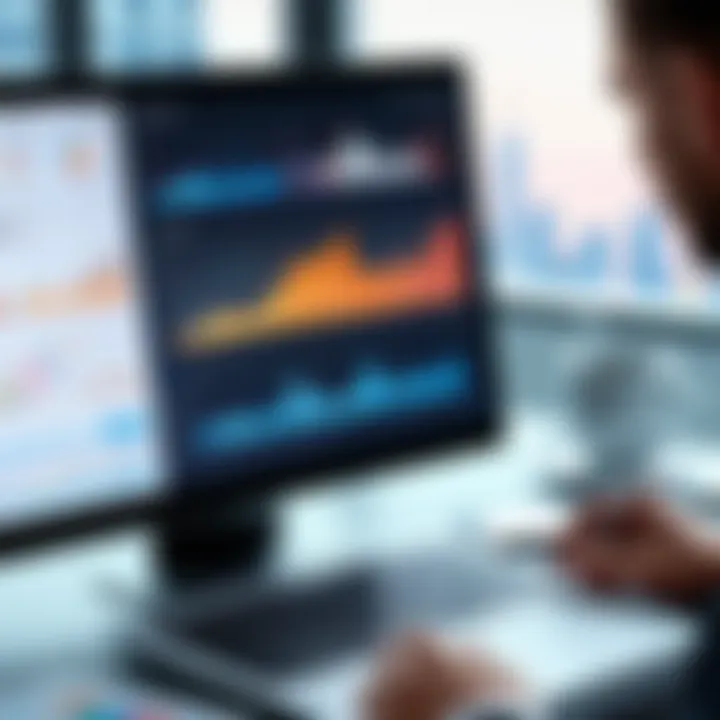
- Collaborative Workshops: Organize workshops where stakeholders can discuss their insights and challenges. This fosters teamwork and ensures diverse perspectives are included in the budgeting process.
- Transparent Communication: Clearly communicate the objectives and methods of predictive budgeting across the organization. Transparency builds trust and encourages feedback.
- Regular Updates and Involvement: Keep stakeholders informed throughout the predictive budgeting cycle. Consistent updates foster a sense of ownership and encourage active participation.
Engaging stakeholders leads to a more comprehensive forecasting process, resulting in better-informed financial decisions.
Future Trends in Predictive Budgeting
Future trends in predictive budgeting present crucial insights into how organizations approach financial planning. As businesses navigate an increasingly complex economic landscape, it becomes essential to adopt methodologies that not only forecast financial realities but also adapt to evolving circumstances. This section will cover significant technologies and changing business needs that shape the future of predictive budgeting.
Emerging Technologies
Emerging technologies play a pivotal role in transforming predictive budgeting processes. The integration of advanced data analytics tools enables businesses to process vast amounts of data quickly and accurately. These tools allow organizations to identify patterns and trends that were previously obscured in more traditional budgeting methods. For instance, cloud computing facilitates real-time data access and collaboration, promoting faster decision-making.
Moreover, artificial intelligence and machine learning contribute greatly by automating data analysis. They can generate predictive models that adapt to new data continuously. This ability to learn from changes in consumer behavior or market dynamics enhances the forecasting accuracy of budgets.
These technological advancements also bring forth challenges, such as ensuring data integrity and promoting user-friendly interfaces for employees who utilize them. Nonetheless, the benefits greatly outweigh the challenges, leading to improved budgetary outcomes.
Evolving Business Needs
Business demands are shifting rapidly due to globalization and technological advancements. With these changes come new expectations for financial planning frameworks. Companies are increasingly required to be agile and responsive. Traditional budgeting methods, which often rely on historical data and fixed assumptions, fall short in this dynamic environment.
To meet evolving business needs, predictive budgeting must become more flexible. Organizations must allow for adjustments to budgets based on real-time feedback. This adaptability enables firms to capitalize on new market opportunities or mitigate potential risks more effectively.
Utilizing predictive budgeting processes not only enhances financial control but also fosters a culture of continuous improvement. Businesses can engage stakeholders more effectively by incorporating their insights into the budgeting process, encouraging collaboration and shared accountability.
"Predictive budgeting is not just about making estimates; it is about anticipating future scenarios and aligning resources effectively."
In summary, the importance of recognizing trends in technology and business needs cannot be overstated. As enterprises strive to refine their predictive budgeting practices, understanding these future trends is crucial for sustained competitive advantage in the market.
Case Studies of Predictive Budgeting
Case studies of predictive budgeting provide valuable insights into how organizations implement this approach in real-world scenarios. They showcase both successful implementations and lessons learned during less favorable outcomes. Understanding these case studies is essential to grasping the practical applications of predictive budgeting and its impact across varying sectors.
Successful Implementations
Successful implementations of predictive budgeting often highlight key success factors that contribute to organizational efficiency and financial accuracy. Here are a few notable examples:
- Walmart: By employing advanced data analytics, Walmart has significantly improved its budget forecasting. The company uses historical data and current trends to make precise estimates, resulting in optimized inventory levels and reduced waste. This proactive budgeting approach helps Walmart remain competitive in the retail space.
- Netflix: Netflix utilizes predictive budgeting to enhance its content investment decisions. By accurately forecasting viewer engagement and subscription growth, Netflix aligns its budget allocation with strategic goals, ensuring a better return on investment.
- Coca-Cola: Through predictive budgeting, Coca-Cola has streamlined its marketing expenditures. The company applies predictive models to assess past campaign effectiveness and market dynamics, allowing it to allocate budgets more wisely and enhance overall performance.
These examples demonstrate how successful predictive budgeting leads to informed decision-making and resource optimization within large institutions.
Lessons Learned from Failures
While many organizations successfully implement predictive budgeting, failures also provide critical lessons. Understanding common pitfalls helps to mitigate risks in future endeavors. Consider the following examples:
- A Major Airline: A significant airline attempted to implement predictive budgeting without robust data analytics tools. The reliance on outdated data led to inaccurate forecasts, resulting in budget overruns and operational inefficiencies. This case illustrates the necessity of using up-to-date and comprehensive data in predictive budgeting.
- A Nonprofit Organization: A nonprofit organization faced challenges when trying to adopt predictive budgeting due to insufficient stakeholder engagement. The lack of communication among departments resulted in poorly planned budgets that were not aligned with the organizationโs mission. This highlights the value of including all relevant parties in the budgeting process.
- Tech Startup: A tech startup launched predictive budgeting during a phase of rapid growth but failed to adjust its models in real-time. As market conditions changed, the startupโs budgeting became irrelevant, leading to financial shortfalls. This underscores the importance of continuous data monitoring and flexibility in budgeting strategies.
A case study serves as a real-life illustration, showcasing successes and revealing the risks of ineffective practices.
Ethical Considerations in Predictive Budgeting
Predictive budgeting is not only about numbers and forecasts. It encompasses broader themes that demand ethical scrutiny. Two main facets of ethical considerations in this context are data privacy and transparency. By addressing these elements, organizations can build trust while applying predictive budgeting methodologies effectively.
Data Privacy Concerns
Data privacy stands as a fundamental consideration in predictive budgeting. As organizations collect vast amounts of data for analysis, the risk of compromising personal information increases. Data breaches can have severe consequences, not just financially, but also in losing consumer trust. Organizations must ensure that they adhere to legal provisions such as the General Data Protection Regulation (GDPR) when handling personal information.
Beyond simple compliance, organizations should adopt best practices to safeguard data. This includes:
- Implementing encryption for sensitive data to prevent unauthorized access.
- Minimizing data collection to what is necessary for predictive budgeting.
- Educating staff about data protection protocols and consequences of data misuse.
Ultimately, the aim is to create a responsible framework for data usage that aligns with ethical standards while achieving accurate predictive outcomes.
Transparency and Accountability
Another critical ethical consideration lies in transparency and accountability. Predictive models can often seem like 'black boxes' where the decision-making process is opaque. This can lead to mistrust among stakeholders and users. It is vital for organizations to be transparent about how predictions are made and which data informs these forecasts.
Transparency can be promoted by:
- Documenting methodologies used in predictive modeling.
- Sharing results openly with stakeholders to foster trust.
- Encouraging feedback from users regarding predictive outcomes to improve accuracy and accountability.
Moreover, accountability mechanisms should be in place. This involves assigning responsibility for data handling processes and ensuring that any errors in predictive budgets are addressed promptly.
"In predictive budgeting, ethical considerations serve not just as a compliance checklist but as a pathway to ensuring responsible practices and trust."
By proactively addressing these ethical issues, organizations can not only enhance their predictive budgeting effectiveness but also lay the groundwork for sustainable practices that resonate well with all stakeholders, especially in an era increasingly focused on data integrity.
Ending
The topic of predictive budgeting holds significant importance within the realm of modern financial planning. This article has explored various aspects of predictive budgeting, focusing on its methodologies, applications, and technologies involved. Understanding its significance is essential for practitioners who seek to improve financial accuracy and operational efficiency in their organizations.
Summarizing Key Takeaways
- Data-Driven Approach: Predictive budgeting relies heavily on data analysis for decision-making. It enhances forecasting accuracy by utilizing historical data, thus enabling organizations to anticipate changes in finances effectively.
- Technological Integration: The role of technology in predictive budgeting is crucial. By incorporating advanced tools for data analytics and forecasting, organizations can achieve more reliable financial plans.
- Strategic Planning: This approach allows businesses to implement scenario planning, which prepares them for possible future conditions. This strategic element is vital in effectively managing resources based on predicted outcomes.
- Public and Private Applications: Predictive budgeting is applicable in various sectors, including corporate finance, public sector budgeting, and nonprofit organizations. Each area benefits from its intent to optimize resource allocation.
- Challenges: Despite its advantages, predictive budgeting faces obstacles, such as data quality issues and resistance to change. Recognizing these challenges enables organizations to devise strategies for efficient implementation.
The Future of Budgeting
The future of budgeting will likely revolve around continuous advancements in technology and data analytics. As organizations face increasing complexity in financial environments, predictive budgeting will grow more integral in their planning processes.
- Emerging Technologies: Innovations in AI and machine learning are set to revolutionize predictive budgeting. These tools will enhance capabilities for analysis and allow for real-time adjustments in financial plans based on evolving data trends.
- Informed Decision-Making: The future will require decision-makers to rely on data more than ever. Predictive budgeting will support this trend by providing accurate forecasts and financial models that guide businesses toward sustainable growth.
- Adaptability: Organizations will need to stay agile, adapting their strategies based on insights obtained through predictive budgeting. This adaptability will be crucial in navigating uncertainty in financial markets.