Advanced Social Media Analytics for Business Success
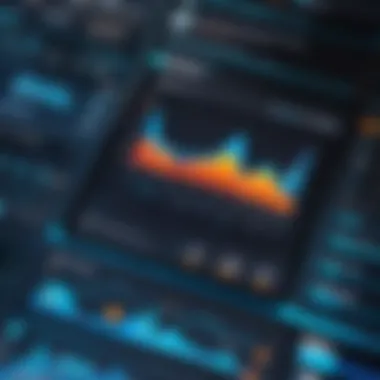
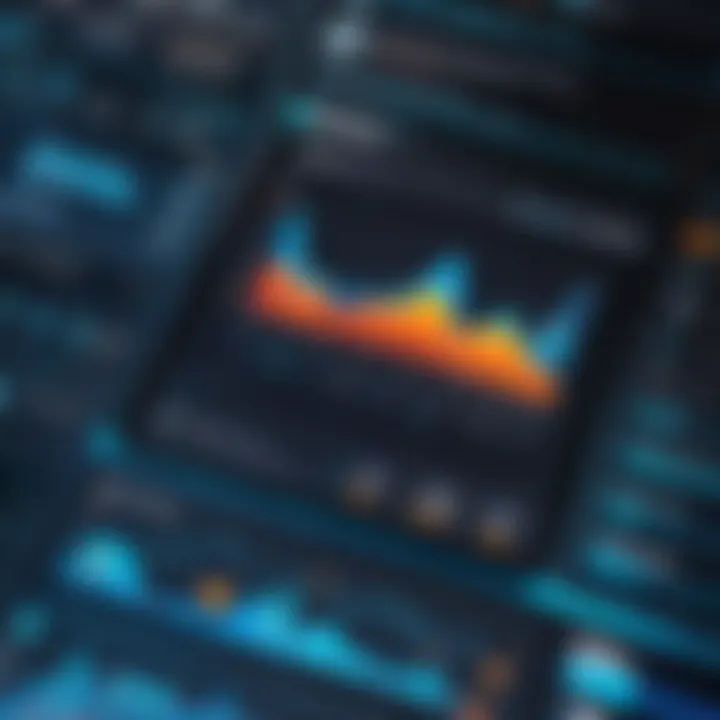
Intro
In today's hyper-connected world, social media has transformed into more than just a platform for sharing life updates. It is a treasure trove of data waiting to be mined for insights. Understanding this landscape can be the key for businesses and individuals looking to make informed decisions. Why is advanced social media analytics gaining traction? Simply put, it empowers organizations to extract actionable intelligence from an ocean of user-generated content.
As we delve into the realm of advanced social media analytics, we'll break down methodologies, tools, and applications that can unlock the potential of social platforms. The discussion will touch on how one can harness data to improve marketing strategies, engage effectively with users, and keep a keen eye on competitor behavior. This exploration also addresses the ethical dimensions of data interpretation and highlights trends that are shaping the future.
Cognizant of the importance of this topic, we now step into the first significant aspect of our exploration.
Overview of Software
Understanding the sheer volume of data churned out by social media necessitates sophisticated analytical tools. The array of software solutions available play a crucial role in fine-tuning how businesses approach these vast data sets.
Purpose and Use Cases
Advanced social media analytics software primarily serves to:
- Monitor Brand Health: Retrieve and analyze mentions across platforms like Facebook and Twitter to gauge public sentiment.
- Enhance Customer Engagement: Identify trends and feedback to foster stronger connections with target audiences.
- Measure Campaign Effectiveness: Evaluate metrics tied to marketing initiatives, driving real-time adjustments for better results.
Different software caters to distinct needs. Moreover, the deployment of such tools ranges from vibrant startups aiming to capture niche markets to large enterprises looking to solidify their brand's dominance.
Key Features
While features vary by software, some common functionalities are:
- Sentiment Analysis: Tailored algorithms interpret user posts or comments, indicating positive, negative, or neutral feelings.
- Real-time Analytics: Immediate insights allow brands to react swiftly to audience interactions.
- Customizable Dashboards: Users can arrange displays to focus on what matters most to specific campaigns or data sets.
Incorporating these features can lead to improved data-driven decision-making processes.
In-Depth Review
A thorough examination of social media analytics software helps determine the right fit for an organization's needs. Here, we'll explore key dimensions of this software category.
Performance Analysis
When considering performance, metrics such as speed and accuracy play a pivotal role. Some platforms may excel in streamlining data from various sources instantly, while others might prioritize in-depth analysis over a longer period.
It's important to consider:
- Scalability: As social media environments evolve, can the software keep up with new data inflows?
- Integration Capabilities: Does it communicate effectively with existing tools like CRM or email marketing platforms?
These factors largely determine an organization's ability to glean meaningful insights without lag or loss of data fidelity.
User Interface and Experience
User experience often defines how effectively teams can use these tools. An intuitive interface can drastically reduce the learning curve.
- Navigation Ease: Users should find it simple to traverse complex features without feeling overwhelmed.
- Customization Options: Can users personalize reports to reflect their unique KPIs and performance markers?
"Data is the new oil, but like oil, it needs refining."
- Unknown
The journey through analytics is rich with possibilities, and the next steps will further illuminate its dynamics.
Preface to Social Media Analytics
Social media analytics has emerged as a cornerstone of modern marketing and brand management strategies. In a world where information flows faster than the blink of an eye, understanding how to interpret and leverage social media data is crucial for businesses and individuals alike. The insights drawn from social interactions not only guide content strategies but also enhance customer engagement and foster brand loyalty. By exploring the nuances of social media analytics, this article aims to provide a comprehensive toolkit for readers looking to refine their marketing efforts through data-driven decisions.
Defining Social Media Analytics
Social media analytics refers to the measurement, collection, and analysis of data from social platforms like Facebook, Twitter, Instagram, and others. The goal? To understand user behavior, track engagement levels, and derive actionable insights from social conversations. At its core, it's about turning raw data into a narrative that informs business decisions. For example, a brand may track mentions of its products in online discussions. If negative sentiments outweigh positive mentions, this could indicate the need for a product revision or a shift in marketing strategy. In simple terms, it transforms chatter into clear communication for brands.
As the digital landscape evolves, the definitions around social media analytics have become more refined. It’s not just about counting likes and shares anymore; the focus has shifted towards understanding how these interactions translate to real-world outcomes, such as increased sales or improved customer loyalty. Essentially, it's about understanding the why and how behind social media activity, rather than just the what.
The Evolution of Social Media Metrics
The journey of social media metrics is an intriguing one. Initially, the primary focus was on vanity metrics—numbers that made brands look good but didn’t necessarily connect with their broader business goals. Metrics such as follower counts and likes reign supreme in the early days of social platforms. It was like measuring popularity at a high school; impressive numbers, but they didn’t reflect true engagement or influence.
Over time, as businesses became more sophisticated in their analytics approach, there was a palpable shift toward metrics that truly mattered. Engagement metrics, such as shares, comments, and click-through rates began to take center stage. Brands now realized that not all followers are created equal. A smaller group of highly engaged followers can be far more valuable than a larger, indifferent audience.
These days, metrics have ventured into more complex territories, incorporating elements of predictive analytics and sentiment analysis. This evolution equips brands to understand not just current trends, but forecast future behavior based on historical data. For instance, a predictive model might analyze past social media interactions to forecast a brand’s upcoming product launch success. Thus, evolving social media metrics align closely with business objectives, turning analytical endeavors into a competitive advantage.
"Metrics must align with strategies; mere numbers lack the power to drive meaningful decisions."
Through this evolution, the field of social media analytics has matured significantly. It’s not just data for data's sake; it's a vital component in crafting comprehensive marketing strategies.
Understanding Data Types in Social Media
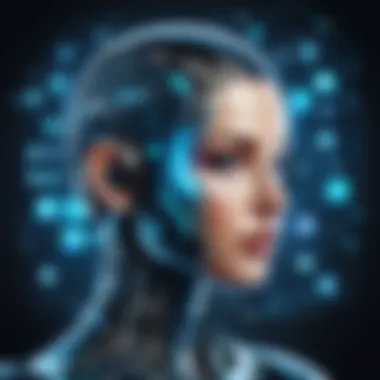
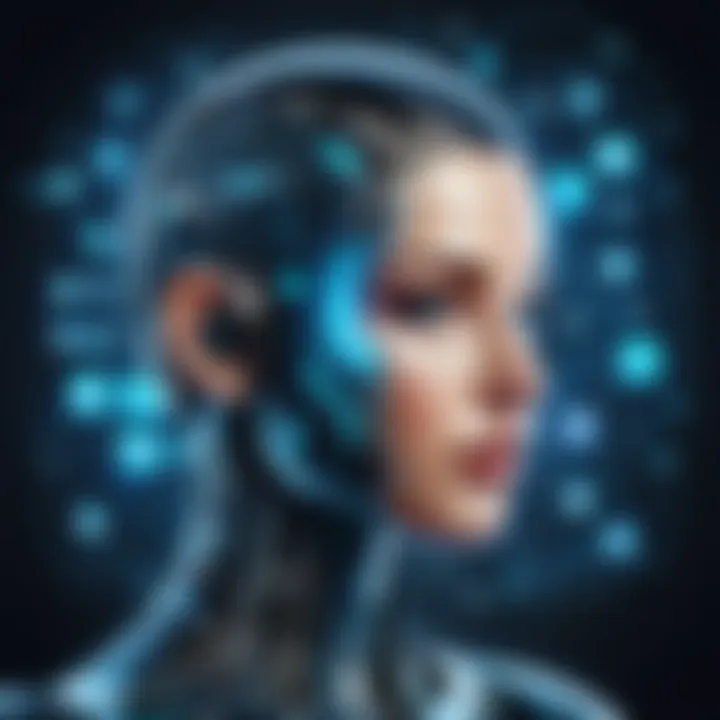
In the realm of social media analytics, grasping the type of data being worked with is crucial. Distinguishing between various data forms not only helps in weighing their relevance but also enables analysts to make informed decisions. This section elaborates on the significance of understanding data types, as well as how they can be effectively harnessed for better insights and targeted strategies.
Quantitative vs Qualitative Data
Data can primarily be categorized into two buckets: quantitative and qualitative. Quantitative data comprises numeric values that lend themselves to statistical analysis. It can often be counted or measured, making it easier to analyze through traditional methods. For instance, the number of likes, shares, retweets, and followers are all quantitative metrics. They provide a clear picture of engagement volume, which aids in assessing the breadth of a campaign's reach.
On the flip side, qualitative data deals with non-numeric information that speaks to the depth of user interaction. Think of this as the emotional undertone in social media conversations. Comments, reviews, and feedback encapsulate user sentiment and opinions, which are rich in nuance but harder to quantify. This type of data is invaluable for understanding user motivations and feelings towards a brand or campaign.
Both data types hold their own weight and can complement each other remarkably well. For example, a spike in followers might be consoled by qualitative feedback revealing discontent over a recent campaign. Thus, blending both data types provides a fuller perspective, allowing marketers to shape their strategy more appropriately.
Engagement Metrics
Engagement metrics serve as the pulse of social media interactions. These are critical indicators that reveal how users interact with content. They go beyond mere numbers, as they reflect user interest and brand affinity. Important engagement metrics include:
- Likes: Indicate approval or enjoyment of a post.
- Shares: Show the extent to which content resonates with users, prompting them to spread it further.
- Comments: Provide direct insight into user opinions and foster community dialogue.
- Click-Through Rates (CTR): Measure the percentage of users who clicked on a post versus who viewed it.
Understanding these metrics helps in evaluating content performance. For instance, if a particular post enjoys a high number of shares but low comments, it suggests that while the content is shareable, it may not elicit much conversation. Thus, strategizing around the content style can be incredibly effective, directly impacting engagement levels moving forward.
Sentiment Analysis Metrics
Diving deeper, sentiment analysis metrics provide a lens through which brands can assess user sentiments and emotions. This entails dissecting qualitative data to categorize emotions expressed in user interactions. Here's where it gets interesting; sentiment can be broken down into three key categories:
- Positive: Indications of approval and satisfaction,
- Negative: Outcries of dissatisfaction and critique,
- Neutral: Commentary that lacks strong emotional charge.
Tools like Natural Language Processing (NLP) come into play here, enabling analysts to programmatically scour through thousands of comments and feedback to draw significant conclusions. Understanding sentiment can guide further actions—whether it’s addressing user complaints directly or amplifying positive reactions through content strategy.
Engaging with users based on their expressed sentiment can transform a good marketing strategy into a great one.
Tools and Technologies for Advanced Analytics
Understanding the landscape of tools and technologies used in advanced social media analytics is crucial for any enterprise looking to leverage data effectively. This section highlights the advantages these tools offer, the different types available, and how they can significantly enhance an organization's analytic capabilities. Moreover, as social media platforms become more complex, the need for sophisticated tools has never been greater. Organizations that invest in these technologies position themselves better to draw meaningful insights from their data.
Leading Software Solutions
When it comes to software solutions for social media analytics, the market is flooded. A few standout platforms include Hootsuite, Sprout Social, and Brandwatch. Each of these tools provides unique features that cater to different needs:
- Hootsuite allows users to manage multiple social accounts, schedule posts, and track performance metrics, making it user-friendly for teams.
- Sprout Social is known for its robust reporting capabilities and user engagement tools, invaluable for businesses focused on customer service.
- Brandwatch specializes in social listening, giving businesses insight into brand sentiment across the web.
These platforms provide not just data collection but also essential analytic functionalities that can unveil trends and user behavior patterns. As a result, the right software can turn raw data into strategic insights.
Open Source vs Proprietary Tools
Organizations must decide between open-source and proprietary tools based on their specific needs and capabilities. Open-source tools like R and Python libraries offer great flexibility and customization options. They can be particularly appealing to developers who wish to tailor solutions closely aligned with their processes. However, open-source tools often require a more significant upfront investment in time and skill.
On the other hand, proprietary tools generally provide more out-of-the-box solutions with user-friendly interfaces. They come with customer support, which can be vital for teams without extensive technical skills. Some examples include Tableau and Adobe Analytics, which provide comprehensive analytics with minimal setup. Ultimately, the choice depends on the company’s resources and analytical objectives.
Integrating Analytics with Marketing Platforms
Integrating analytics tools with existing marketing platforms can create a coherent ecosystem of data and insights. This connection can facilitate better decision-making through real-time insights, allowing teams to be agile in their strategies.
For instance, connecting Google Analytics with Facebook Ads can help marketers understand how their campaigns perform in terms of driving traffic and engagement. Moreover, many modern platforms allow for the integration of APIs, which means data from various sources can be collated and analyzed seamlessly. This capability not only simplifies workflow but also enhances the accuracy of data-driven decisions.
Effective integration is key. When analytics tools talk to your marketing platforms, your strategy can pivot based on what the data is really saying.
In summary, the right combination of tools and technologies can pave the way for better insights and more successful social media strategies, ultimately achieving higher returns for businesses.
Data Interpretation and Analytics Strategies
Data interpretation and analytics strategies play a pivotal role in the vast realm of social media analytics. They act as the bridge between raw data and actionable insights, transforming the numbers and metrics collected into coherent narratives that inform decision-making processes. Without proper interpretation, even the most sophisticated tools and technologies can fall flat, yielding results that are merely interesting but not particularly useful.
Transforming Data into Insights
To transform data into insights, one must start by understanding its context. Raw data—be it likes, shares, comments, or clicks—can tell a story, but only if one knows how to interpret it correctly. The first step in this transformation is filtering through the noise. This means identifying relevant data points that align with specific goals.
Consider a brand launching a new product on Instagram. They might track engagement metrics such as the number of likes, comments, and direct messages related to the launch post. However, without a framework for interpretation, it's easy to overlook critical insights. For example, an increase in comments might indicate that the audience has questions or concerns, which could be a goldmine for understanding customer sentiment. By analyzing these indicators, the brand can adjust its messaging or develop FAQs to address potential issues, thereby enhancing user experience.
"Data only becomes valuable when it's transformed into insights that drive action."
Moreover, visualizations can significantly aid this process, allowing for patterns and trends to emerge more clearly. Tools such as Tableau or Microsoft Power BI can illustrate data through graphs or dashboards, making it easier to spot anomalous patterns or emerging trends.
Establishing KPIs for Success
Key Performance Indicators (KPIs) serve as quantifiable measures that gauge a company's performance against its goals. Establishing effective KPIs in social media analytics is crucial, as they allow businesses to objectively assess their strategies. Properly defined KPIs not only reflect the effectiveness of social media efforts but also guide future initiatives.
For instance, a digital marketing team might establish KPIs such as
- Engagement Rate per Post
- Conversion Rate from Social Platforms
- Customer Feedback Score
- Follower Growth Rate.
These indicators provide benchmarks that are both actionable and informative. They allow companies to decipher whether they are on track to meet company objectives or require adjustments to their approach. A focused KPI assessment can also reveal any gaps in strategy, suggesting that perhaps more resources should be allocated to certain platforms or content types based on user response.
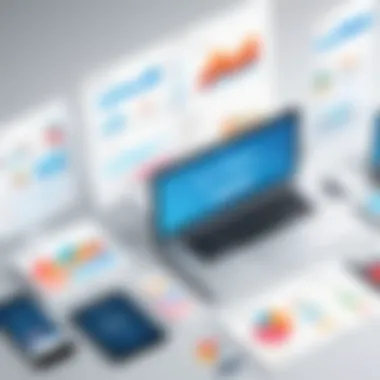
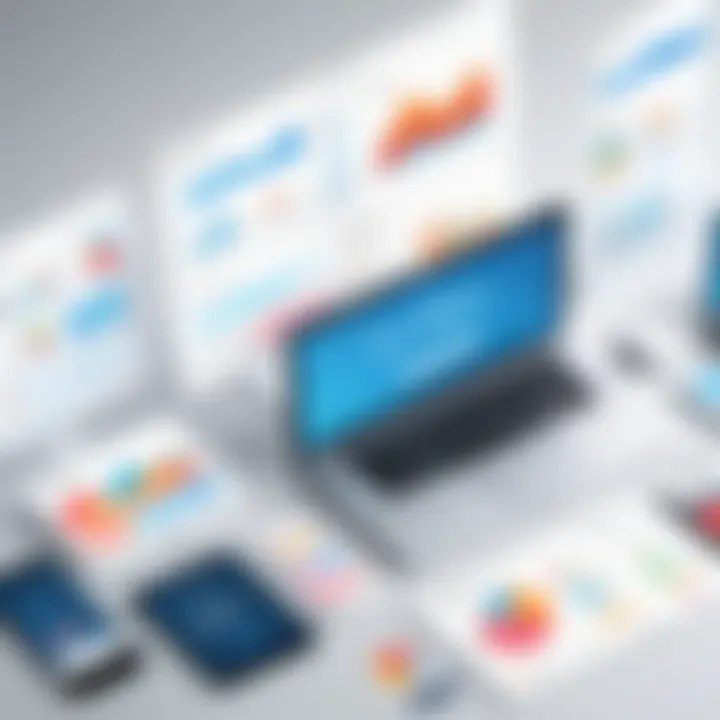
A/B Testing and Experimentation
A/B testing, also known as split testing, is a fundamental strategy in the toolkit of any data-driven marketer. It involves comparing two versions of content against each other to determine which performs better. In the context of social media analytics, A/B testing can apply to everything from ad copies to imagery, posting times, or call-to-action phrases.
When executing an A/B test, it’s essential to follow a systematic approach:
- Define the Objective: Clearly state what you aim to accomplish—higher click-through rates, increased engagement, etc.
- Create Variants: Develop two variations (A and B) of the content to test. Maintain all elements the same except for one variable (e.g., the headline).
- Set a Testing Period: Determine how long you'll run the test to gather enough data for reliable conclusions.
- Analyze Results: Use statistical methods to determine the winner—did variant A outperform variant B? Why or why not?
A real-world example could illustrate a well-known brand like Coca-Cola, which might test two different advertising approaches on Twitter: one emphasizing their health-conscious beverages and the other celebrating nostalgia with classic branding. By analyzing user engagement, click rates, and conversion statistics, Coca-Cola could refine its future advertising strategies based on solid evidence.
In summary, a comprehensive understanding of data interpretation combined with strategic analytics ensures that businesses can make informed decisions. This intersection not only empowers organizations to make sense of the data at their fingertips but also optimally aligns their social media activities with broader business objectives. Proper transformation of data, diligent KPI establishment, and clever A/B testing all play vital roles in crafting effective data-driven strategies.
The Role of Artificial Intelligence in Analytics
Artificial Intelligence (AI) stands at the forefront of advanced social media analytics, transforming how data is analyzed and interpreted. Its relevance in this field cannot be overstated. By automating complex processes and improving accuracy, AI empowers businesses to glean insights that are not only actionable but also significantly more profound than what was possible before.
Incorporating AI into analytics hinges on several key elements. Firstly, it enhances the ability to process vast amounts of data quickly. Traditional methodologies can often be likened to a snail's pace when compared to AI efficiencies. Algorithms can sift through terabytes of data, identifying patterns and trends that are indicative of user behavior and engagement.
Moreover, the benefits of AI extend into nuanced areas like creative content generation. For example, machine learning algorithms analyze what type of posts resonate most with target audiences, thereby aiding marketers in crafting content that aligns with consumer preferences. This smart data-driven approach not only improves response rates but optimizes resource allocation in campaigns, which is paramount in today’s fast-paced digital environment.
However, with great power comes great responsibility. The use of AI also prompts critical considerations around ethical and privacy issues, which can't be overlooked. Businesses must tread carefully to ensure compliance with regulations while maintaining transparency. The balance between leveraging AI for insight and protecting user rights is delicate. Striking this balance is essential to foster trust not only with consumers but with regulatory bodies as well.
Machine Learning Models in Social Media
Diving into the specifics, machine learning models represent a significant component of the AI toolkit in social media analytics. These models, built through algorithms that learn from data, have various applications. They can predict user behavior based on past interactions, offering marketers a roadmap for future campaigns. For instance, a popular model used in this domain is the decision tree, which breaks down complex decisions into simpler parts, enabling a more straightforward analysis of outcomes.
Another effective machine learning approach is clustering, which groups users based on similarities in behavior or demographics. This segmentation enables brands to tailor their messaging, ensuring that campaigns are not a one-size-fits-all approach. Instead, they become laser-focused, increasing engagement and driving conversions.
This code demonstrates a basic clustering algorithm that groups user behaviors, allowing businesses to identify trends among different segments. The implications of using such models can significantly enhance engagement strategies, transforming the analytical landscape for social media.
Predictive Analytics Applications
Predictive analytics is another realm where AI shines brilliantly in social media. It allows businesses to anticipate future trends based on historical data. Think of it as a crystal ball that provides insights into what might happen next, guiding marketers to craft proactive strategies.
For example, a brand tracking the launch of a new product can utilize predictive models to ascertain the best time for promotions. By analyzing similar past campaigns, insights can inform the timing and nature of subsequent efforts, ensuring higher visibility and engagement.
The predictive capabilities extend to identifying potential customer churn. By analyzing factors that lead to customer disengagement, businesses can intervene before it's too late. Targeted retention campaigns can be launched based on these forecasts, improving customer loyalty metrics and, subsequently, the bottom line.
"AI’s role in predictive analytics allows businesses to not just react to trends, but to anticipate and shape them."
To wrap it up, the integration of Artificial Intelligence into social media analytics is shaping a new era of understanding consumer behavior. It allows businesses to create meaningful engagements, tailor content, and predict trends with unprecedented accuracy. As this field evolves, practising ethical use and ensuring data protection will remain pivotal in harnessing the full potential of AI-driven analytics.
Case Studies in Advanced Social Media Analytics
Understanding advanced social media analytics is not just about the numbers and metrics; it’s also about real-world applications and the tangible effects these tools and practices have on businesses. Case studies provide critical insights into how theories translate into practice. They serve as powerful proof of concept, illustrating both effective strategies and common pitfalls that companies face when harnessing the vast amounts of data generated online. By examining successful examples and those that turned out to be failures, professionals gain a well-rounded perspective on the landscape of social media analytics.
Success Stories from Leading Brands
When it comes to successful implementations of social media analytics, companies like Nike and Starbucks show how leveraging data can propel brands to new heights. For instance, Nike utilized a data-driven approach to enhance its customer engagement strategies. By analyzing social media interactions, they identified key consumer preferences and unlocked insights on how to tailor their advertising.
This strategy paid off—Nike saw a notable uptick in sales, directly linking their analytical efforts with revenue growth. Moreover, they also tapped into user-generated content, using hashtags to engage consumers and foster a community around their products.
Another remarkable case is that of Starbucks, which devised a loyalty program informed by extensive analysis of customer interactions on social media. They recognized patterns in purchasing behaviors and pieced together customer preferences through sentiment analysis. The results? An increase in loyal customers who constantly interacted with the brand online, further driving sales and customer satisfaction.
Lessons Learned from Analytics Failures
However, not all stories in the sphere of social media analytics are triumphant. Several major companies have faced setbacks that underscore the importance of a thoughtful approach towards data interpretation. Take the case of PepsiCo, which launched a social media campaign that went awry due to a lack of proper sentiment analysis. Their advertisement featuring Kendall Jenner faced significant backlash, with users branding it as tone-deaf and out of touch with contemporary issues.
This incident highlighted a critical lesson: relying solely on quantitative data without understanding the qualitative nuances can lead to disastrous outcomes. A failure like this serves to remind companies of the importance of fully grasping the context surrounding their data.
- Key Takeaways from these lessons include:
- Understanding your audience: It’s vital to comprehend not just what your data tells you, but what it implies about public sentiment and current events.
- Iterating strategies based on feedback: Continuous learning and adaptation from failures foster a more resilient approach to analytics.
- Integrating analytics across teams: Ensuring that marketing, data analysis, and communication departments work in tandem can improve overall strategy effectiveness.
In sum, case studies in advanced social media analytics offer invaluable lessons. They showcase how to apply theoretical concepts to real-world scenarios, serving both as guides to success and cautionary tales against the common pitfalls in the realm of data analysis.
Ethical Considerations in Social Media Analytics
Ethical considerations hold significant weight in the realm of social media analytics. As tools become increasingly sophisticated in gathering and interpreting data, it becomes critical for organizations to navigate the ethical landscape carefully. Ethical practices not only foster trust among users but also ensure compliance with regulations that are continuously evolving. Mismanagement or disregard for ethical standards can result in severe consequences, including reputational loss or legal repercussions.
Privacy Issues and Data Protection
Privacy is at the forefront of discussions surrounding social media analytics. Given that personal data can be very sensitive, safeguarding it is of utmost importance. Organizations must tread carefully, ensuring that they implement appropriate measures for data protection. Data breaches can have dire consequences, both for individuals and brands.
Several practices enhance data protection:
- Anonymization of Data: By removing personally identifiable information, companies can secure user privacy while still leveraging data to extract insights.
- Encryption Protocols: Utilizing advanced encryption methods for data storage and transmission protects sensitive information from unauthorized access.
- User Consent: Gaining explicit permission from users to collect and analyze their data is not just ethical; in many jurisdictions, it’s a legal requirement. Transparency about how data will be used promotes a sense of trust.
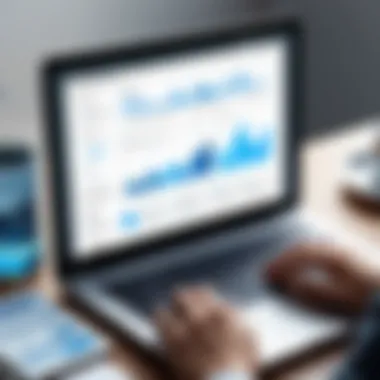
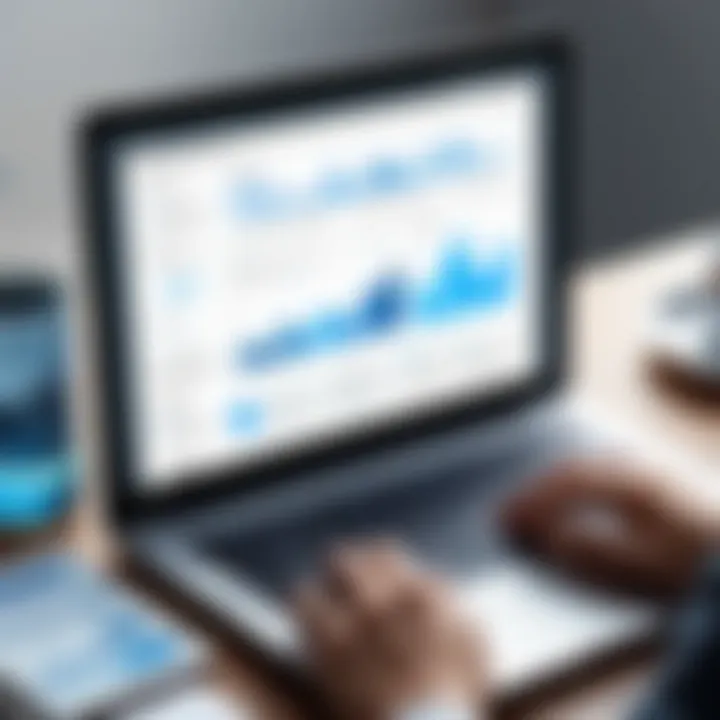
The stakes are high. An adverse incident can not only dismantle consumer trust but also attract scrutiny from regulatory bodies, drawing fines and sanctions. Thus, a proactive approach to privacy and data protection turns from a regulatory necessity to a competitive advantage.
Transparency and Ethics in Data Usage
Transparency is the cornerstone of ethical data usage. Companies should not only be clear about what data is being collected but also how it's being utilized. This openness cultivates an environment of trust and respect between the organization and its audience.
When discussing transparency, several key points arise:
- Clear Policies: Providing clear data consumption policies allows consumers to make informed choices. A transparent privacy policy reinforces a commitment to ethical practices.
- Regular Audits: Frequent evaluations of data usage practices ensure compliance with ethical standards and can identify potential areas for improvement.
- User Education: Informing users about how their data enriches their experiences can demystify the analytics processes. If users understand the benefits, they may feel more willing to engage with the data collection processes.
"Companies thrive not just on data but on trust. Transparency in data usage strengthens this bond."
In summary, the ethical considerations surrounding social media analytics are multifaceted and far-reaching. Balancing data collection with a commitment to privacy and transparency is essential for building lasting relationships with users. Organizations that prioritize ethical considerations shall not only survive but thrive in the complex digital landscape.
Future Trends in Social Media Analytics
In the fast-paced realm of social media, trends evolve quicker than you can refresh your feed. Understanding future trends in social media analytics is not just about keeping up; it’s about staying ahead. As businesses rely more on data-driven decision-making, they must recognize the importance of anticipating changes in analytics methodologies, user behavior, and regulatory landscapes. These trends will shape how organizations interact, market, and communicate with their audiences.
Evolving Algorithms and Metrics
As algorithms become smarter, they significantly alter the metrics by which success is measured. Social media platforms are constantly updating their algorithms to prioritize content that fosters genuine engagement rather than mere visibility. This shift means companies have to think critically about how they craft their content and the metrics they focus on.
- Engagement over Reach: Brands must now track more nuanced metrics like meaningful comments, shares, and active conversations rather than just likes and impressions. This evolution fosters a deeper understanding of audience engagement.
- Predictive Analytics: Machine learning models are gaining traction in social media analytics. These models analyze historical data to predict future user behaviors and preferences, allowing marketers to tailor their strategies proactively. Companies that leverage predictive analytics stand to gain significant advantages across their campaigns.
- Personalization Metrics: As users demand more tailored experiences, companies must develop metrics to measure the effectiveness of their personalized content. This entails not just tracking clicks but understanding conversion rates derived from specific audience segments.
These evolving metrics are not just numbers; they carry the potential to inform your brand’s narrative. The promise these trends hold lies in extracting actionable insights that directly affect outreach and engagement.
The Impact of Regulatory Changes
As social media analytics approaches a more regulated environment, businesses must acknowledge the implications of privacy laws and ethical considerations. Recent regulations, such as the General Data Protection Regulation (GDPR) in Europe, emphasize data protection, impacting how companies collect and analyze user data.
- Compliance Complexity: Navigating the legal landscape requires a profound understanding of how data can be harvested without infringing on privacy regulations. Firms will have to re-evaluate their data collection practices, which might complicate existing strategies but ultimately leads to more ethical standards.
- Transparency Requirements: Users today are more aware of their rights. Brands must be transparent about how they use data and engage in open discussions with their audience regarding their privacy. This approach builds trust, a valuable currency on social media.
- Evolving Standards for Consent: With increasing scrutiny, organizations will need to ensure they have the proper consent mechanisms in place before collecting and processing any data. This shift might mean adopting more stringent data capture practices, but it also creates opportunities for brands to lead the charge in ethical analytics.
Staying compliant with regulatory changes is not merely about avoiding fines; it’s about nurturing a relationship built on trust with your audience.
In summary, as we look to the future of social media analytics, staying informed of evolving algorithms and regulatory shifts is essential. These trends will not only govern how analytics is conducted but also redefine how companies engage with their audiences, pushing them to prioritize integrity and authenticity in their digital strategies.
Integrating Analytics into Business Strategy
In today’s digital age, businesses must recognize the profound impact that analytics can have on shaping their strategic direction. Integrating analytics into business strategy isn't merely a box to check off; it is an essential function that can enhance decision-making and drive growth. As organizations gather vast amounts of data from social media interactions, the wise integration of this information allows for a more nuanced understanding of market trends and customer preferences. This strategic integration aids businesses in identifying opportunities and mitigating risks through data-backed decisions.
When businesses align their analytical efforts with overarching objectives, they position themselves to capture valuable insights and adapt proactively to changes in the market landscape. One key benefit of this integration is the ability to make informed decisions that reflect real-time data. Being agile in response to market shifts can differentiate a business from its competitors, creating a significant advantage.
Moreover, the integration process demands a blend of expertise and vision from multiple departments, fostering a culture of collaboration within the organization. Every team—from marketing to product development—benefits from shared analytics insights, ultimately leading to a more unified business strategy.
"Analytics shouldn't just support operations; they should propel the business forward."
Aligning Analytics with Business Goals
Aligning analytics with business goals is critical for harnessing the full potential of data insights. This alignment ensures that analytical frameworks are not just technical exercises but instead resonate with the strategic priorities of the organization. For a business aiming for growth, whether it be increased market share, improved customer satisfaction, or enhanced operational efficiency, analytics must be intimately connected to these goals.
To achieve this, organizations should define clear KPIs (Key Performance Indicators) that reflect their objectives. For example:
- Customer Retention Rate: Understanding how analytics informs retention efforts enables businesses to tailor their products or services to meet evolving customer needs.
- Conversion Rates: By tracking how effectively leads are turned into customers, businesses can fine-tune their marketing efforts.
- Net Promoter Score (NPS): This measure, which captures customer loyalty and satisfaction, offers a direct reflection of the customer experience, providing a valuable feedback loop.
Once KPIs are established, businesses should continuously analyze their performance against these targets and adjust strategies accordingly. Adapting swiftly to data insights encourages a proactive mindset, keeping a business one step ahead of potential challenges.
Crafting Effective Marketing Campaigns
The journey from data collection to effective marketing campaigns is paved with thoughtful analysis and strategic planning. Successful marketing campaigns are not born in a vacuum; they are products of rigorous analytics. This involves understanding not only what audiences are saying, but also how they are engaging with content across platforms.
When crafting marketing tactics based on analytical insights, consider the following:
- Target Audience Segmentation: Utilize data to identify distinct audience segments, tailoring messages that resonate with each group's preferences and behaviors.
- Channel Optimization: Depending on where your audience hangs out—whether it’s on Facebook, Instagram, or even niche platforms—leverage analytics to determine where to focus marketing efforts.
- A/B Testing: Run tests on different campaign elements (like headlines, images, or calls to action) to discover what resonates most with your audience. Adjustments based on test performance can significantly improve campaign efficacy.
- Monitoring and Iteration: After launching a campaign, tracking its performance in real-time allows for quick pivots and changes based on audience interaction. This leads to more effective marketing efforts over time.
By integrating analytics into marketing campaign development, businesses can foster stronger connections with their audience and increase overall campaign success. This holistic approach to analytics not only optimizes short-term marketing activities but also builds a sustainable strategy for long-term engagement.
Closure
The examination of advanced social media analytics in this article underscores its profound significance in today’s digital environment. As businesses increasingly rely on data-driven decision-making, understanding the nuances of social media metrics becomes imperative. This conclusion encapsulates the journey through analytics, reaffirming key components and their far-reaching impacts on organizations and individuals alike.
Recap of Key Insights
Throughout the discussion, we’ve highlighted several pivotal elements that contribute to the effective utilization of social media analytics:
- Data Interpretation: The ability to sift through data and extract meaningful insights has evolved into an essential skill. Analytics transform raw numbers into narratives about customer behavior and market trends.
- AI Integration: Artificial Intelligence is not just a buzzword; it’s reshaping how we interpret data. From predictive analytics to sentiment analysis, AI technologies enable a deeper understanding of user interactions.
- Ethical Considerations: With great data comes great responsibility. Ethical practices in data collection and usage are not only legally mandated but also crucial for maintaining trust among users.
- Strategic Alignment: Integrating analytics into business strategies allows for targeted marketing efforts and more efficient resource allocation. When analytics align with business goals, success is often the result.
Thus, the key is not just gathering data but interpreting and utilizing it wisely to stay ahead of the curve.
The Path Forward
Looking ahead, the trajectory of social media analytics heralds exciting prospects for both businesses and industries:
- Continuous Learning: As platforms evolve and user behaviors change, staying updated with the latest tools and practices will be critical. Companies must adopt a culture of continuous learning to harness the full potential of available analytics.
- Collaborative Efforts: Interdisciplinary collaboration will become central to analytics initiatives. Teams from marketing, IT, and legal departments must work together to ensure that data initiatives are both innovative and compliant with regulations.
- Focus on Customer Experience: Future analytics strategies should center around enhancing user engagement and experience. Understanding the customer journey can offer valuable guidance on product development and service improvements.
In closing, as we stand at the crossroads of technology and marketing, the effective use of advanced social media analytics can be a game-changer. Organizations that embrace these methodologies are likely to foster deeper connections with their audiences, ultimately paving the way for sustained success.