Mastering SPSS: Quick Guide for Effective Learning
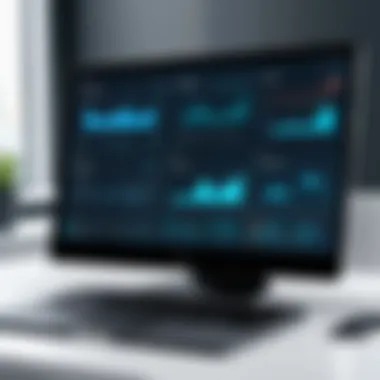
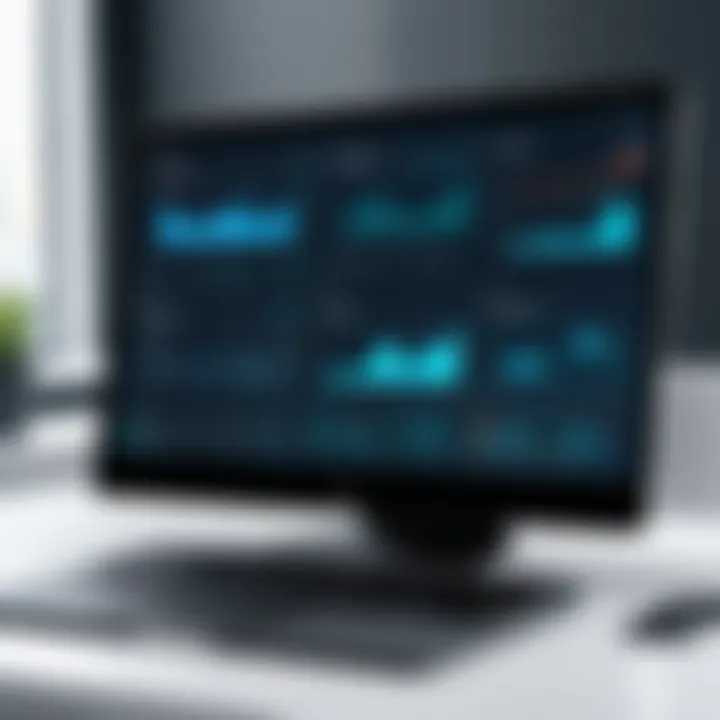
Intro
Understanding statistics and data analysis is a crucial competency in today’s data-driven world. SPSS, or Statistical Package for the Social Sciences, is one of the primary tools available for these purposes. This guide aims to equip you with the necessary skills to effectively utilize SPSS, allowing you to harness its capabilities for your projects. This guide is designed for users at varying levels of expertise, ensuring that everyone can find value within these pages.
Overview of Software
Purpose and Use Cases
SPSS serves as a versatile software package commonly used for statistical analysis in social science, but its applications extend beyond this realm. Researchers, business analysts, and academics frequently employ SPSS for different types of data manipulation and analysis. The software is particularly useful in areas such as:
- Academic Research
- Market Research
- Health and Social Sciences
- Survey Data Analysis
- Quality Improvement Projects
By mastering SPSS, users can make more informed decisions, validate research findings, and develop actionable insights from complex datasets.
Key Features
SPSS boasts various features tailored to simplify high-level statistical computations while remaining user-friendly. Here are some of the prominent features:
- Data Handling: SPSS allows for efficient data entry, manipulation, and organization, facilitating easier analysis of large data sets.
- Statistical Procedures: The software supports a vast array of statistical tests, including t-tests, ANOVA, regression analysis, and more.
- Graphical Output: Users can create visually appealing charts and graphs to communicate results effectively.
- Customizable Syntax: For advanced users, SPSS provides the option to use syntax commands for precise control over data processes and operations.
- Integration with Other Tools: SPSS can interface with various programming languages such as R and Python, enhancing its functionality and versatility.
In-Depth Review
Performance Analysis
In performance considerations, SPSS delivers robust capabilities thanks to efficient algorithm designs. Users generally report quick execution times, even with sizable datasets. The software is capable of handling multiple simultaneous analyses without significant loss of performance, which makes it suitable for comprehensive data exploration.
User Interface and Experience
The user interface of SPSS is primarily designed for ease of use. New users can find their way around the software relatively quickly thanks to intuitive menu structures and options. Some key points about the interface include:
- Menu-Driven: Most features are accessible through drop-down menus, ideal for beginners looking for straightforward navigation.
- Help Resources: SPSS contains integrated help options, providing guidance on statistical techniques and software functions directly within the application.
- Customizable Workspace: Users can tailor their workspaces for improved efficiency, allowing them to prioritize tools they frequently use.
"Mastering SPSS can empower users to unlock analytical insights that drive impactful decisions in research and industry settings."
In summary, SPSS combines powerful statistical capabilities with user-friendly interfaces, making it a favored choice among various professionals. Each feature lends itself to practical applications, further underscoring the necessity for a comprehensive understanding of the software.
Foreword to SPSS
SPSS, which stands for Statistical Package for the Social Sciences, is a powerful statistical software widely used for data analysis in various fields. This section is essential for readers to understand SPSS's role in modern data analytics and research. Familiarizing oneself with SPSS presents not only an opportunity for learning statistics but also a way to become proficient in data interpretation and decision-making based on empirical evidence.
By understanding SPSS, users can harness the capabilities of this software to transform raw data into meaningful insights. Moreover, mastering SPSS bolsters career prospects in fields such as social sciences, healthcare, marketing, and education, making it a valuable skill in today's data-driven world.
Overview of SPSS
SPSS is designed to perform a wide range of statistical analyses. It incorporates a user-friendly interface that allows both novice and experienced users to interact with complex statistical data without extensive coding knowledge. The software offers various functionalities, from basic descriptive statistics to more advanced modeling techniques. Users can input data from various sources, seamlessly perform analyses, and export results for reporting purposes.
The software supports multiple data formats, making it versatile in handling different datasets. SPSS features several built-in functions for statistical tests including T-tests, ANOVA, and regression analysis, enriching the data analysis process. Additionally, SPSS includes graphical tools that help in visualizing results, adding another layer of understanding to the data.
Importance in Data Analysis
In any data analysis project, the methodology employed determines the quality of the insights gained. SPSS provides a structured environment where users can apply statistical principles effectively. The importance of utilizing SPSS goes beyond simply obtaining numbers; it allows researchers to identify patterns, relationships, and trends within the data.
Using SPSS can lead to more informed decision-making processes. For instance, businesses can analyze consumer behavior, while researchers can evaluate the effectiveness of interventions. The ability to accurately analyze data is crucial; therefore, SPSS plays a significant role in the integrity of research results.
SPSS not only facilitates complex statistical analyses but also helps produce insightful visual representations of data, essential for effective communication of findings.
Target Audience for Learning SPSS
The primary audience for learning SPSS includes students in fields such as psychology, sociology, and education, where quantitative research methods are commonly employed. However, the utility of SPSS extends to professionals working in data analysis, marketing research, and business analytics.
Another important group consists of software developers and IT professionals. Understanding how to manipulate and analyze data using SPSS can enhance their work with databases and data processing applications.
Setting Up SPSS
Setting up SPSS is a crucial first step in utilizing this powerful statistical software for your data analysis needs. Proper setup ensures that users can efficiently access the features and functions that SPSS offers. Furthermore, understanding the requirements and processes involved in the setup can save time and reduce frustration. By following the systematic approach to installation and configuration, users will prepare a solid foundation for their analytical work.
System Requirements
Before installing SPSS, it is essential to check the system requirements. SPSS requires a compatible operating system. Generally, SPSS can run on Windows and macOS platforms.
Key components include:
- Operating System: Windows 10 or later / macOS Catalina or later.
- Processor: At least a dual-core processor is recommended.
- RAM: A minimum of 4 GB is essential; however, 8 GB or more improves performance.
- Disk Space: Ensure at least 2 GB of available hard disk space.
It is wise to verify if your machine meets these requirements before proceeding.
Installation Process
Installing SPSS is straightforward if users follow the correct steps. Start by downloading the latest version from the official IBM SPSS website.
- Locate the installer: Once downloaded, double-click the installer file to initiate installation.
- Follow prompt instructions: The setup wizard will guide users through the necessary steps.
- License Agreement: Accept the license agreement to proceed with installation.
- Select installation type: Choose between a typical or custom installation based on your needs. The typical option is suitable for most users.
- Finalize installation: Once the installation completes, restart your computer if prompted.
Initial Configuration and Preferences
After installation, users should configure SPSS settings to streamline their workflow. The initial configuration allows users to customize preferences according to their specific requirements.
- Datapath Configuration: Go to Edit > Options and set the default directory for saving files.
- Variable and Data View Setup: Learn how to switch between Data View and Variable View, as this is important for managing datasets.
- Customizing Output Viewer: Under Preferences, adjust the Output Viewer settings to enhance data displays.
By taking these steps, users can ensure that SPSS is set up correctly and tailored to their specific analytical tasks. This preparation is crucial for effective data analysis and will enable you to focus on insights rather than technical hiccups.
Fast and proper SPSS setup can greatly enhance your experience and efficiency with the software.
Navigating the User Interface
Understanding the user interface of SPSS is essential for efficient data analysis. A well-organized interface aids in easier navigation and quicker access to needed features. This familiarity can significantly enhance productivity, reduce errors, and streamline workflows. SPSS is equipped with a versatile layout that supports both novice and experienced users, making the learning curve less daunting.
Understanding Menus and Toolbars
SPSS organizes its functionalities into menus and toolbars, creating a structured environment. The main menu bar includes essential options like , , and . Under these menus, various commands and subcommands are categorized, helping users find the needed functions swiftly.
Key elements of the menus are:
- File Menu: Here, you manage your data files and output. It provides options like opening, saving, and exporting files.
- Edit Menu: This contains basic options for manipulating data, such as cut, copy, and paste.
- Analyze Menu: Critical for statistical analysis, this menu offers tests, procedures, and descriptive options.
Toolbars provide quick access to frequently used commands. Users can customize these to include their most-used tools, enhancing efficiency. Learning to navigate these menus and toolbars is foundational for harnessing the power of SPSS.
Working with Data View and Variable View
In SPSS, data is presented primarily in two views: Data View and Variable View. Understanding these views is crucial for effective data management.
- Data View shows the actual data that has been entered. Each row represents a case, and each column represents a variable. This view is similar to a spreadsheet, allowing users to edit and manipulate data directly.
- Variable View displays metadata regarding the variables. Users can define attributes such as the variable name, type, width, decimals, and labels. This organizational structure prevents mistakes during analysis. Knowing when to switch between these views is important for accurate data management.
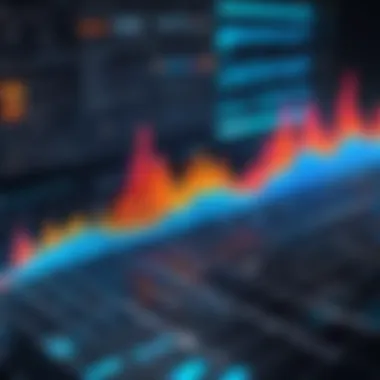
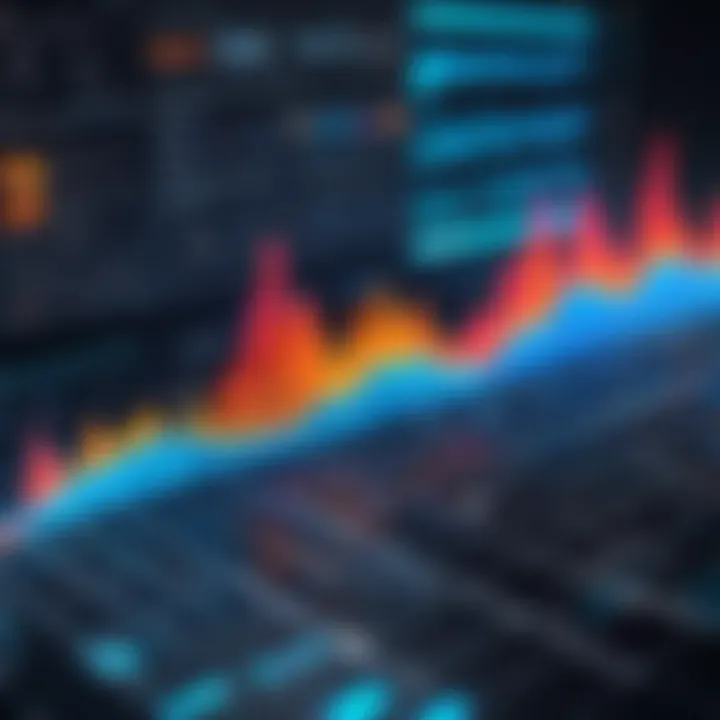
Navigating Output Window
The Output Window is where results from your analyses are displayed. It can hold tables and charts created from the commands executed in SPSS. Familiarity with this window allows users to interpret and present results effectively.
- Structure of the Output Window: Each output can be organized into different sections. These sections can be expanded or collapsed, helping manage visibility.
- Exporting Results: Professionals often need to share their findings. The Output Window allows for easy exporting to formats like PDF or Word, making sharing straightforward.
"The ability to navigate through the Output Window smoothly is crucial for presenting data analysis results clearly."
In summary, becoming adept at navigating the user interface, including menus, data views, and the Output Window, lays a solid foundation for successful SPSS usage. Promoting familiarity with these components could lead to more efficient data analysis and reporting.
Data Handling in SPSS
Data handling is a critical component when working with SPSS, as it lays the foundation for effective data analysis. Without proper management of data, the results obtained can be misleading or even incorrect. Understanding how to import, clean, and transform data will not only enhance the user’s proficiency but also ensure the reliability of outcomes. In this section, we will cover these essential aspects in detail.
Importing Data
Importing data into SPSS is the initial step in the analysis process. It is crucial to bring in data from various sources such as Excel, CSV, or database management systems seamlessly. SPSS supports multiple formats, which allows for flexibility and convenience.
When importing data, it is important to follow these steps:
- Select the appropriate file type: Choose the correct option within SPSS to match the data file you are working with.
- Verify the data structure: Ensure that variable names and data types are correctly interpreted during the import process.
- Check for errors: After importing, review the data for any discrepancies or issues that can affect your analysis.
Using these practices will facilitate a smooth transition into SPSS and help maintain data integrity.
Data Cleaning Techniques
Data cleaning is a vital process that involves identifying and rectifying errors in the dataset. This step is necessary to improve accuracy and ensure analysis yields valid results.
Typical data cleaning techniques include:
- Removing duplicates: Eliminate any repeated entries that can skew analysis.
- Handling missing values: Decide how to address gaps in data, whether to remove records, adjust with mean values, or use regression methods.
- Standardizing data formats: Ensure all data follows a consistent format, especially for variables like dates and categories.
Each of these actions contributes to the overall quality of the analysis by providing a reliable dataset as the starting point.
Transforming Data for Analysis
Transforming data involves applying various techniques to prepare it for deeper analysis. This process can include creating new variables, recoding existing ones, and summarizing data, tailored to fit analytical needs.
Key transformation methods include:
- Recoding variable values: Changing the values of a variable to better represent what they signify, often useful in simplifying categories or adjusting scales.
- Creating computed variables: Deriving new variables from existing data to provide additional insights, for example, calculating a score based on several factors.
- Aggregating data: Summarizing data to a higher level to analyze trends over time or across different groups.
Transforming data allows for more meaningful interpretations and paves the way for advanced analytics.
Understanding the steps to handle data correctly in SPSS not only increases efficiency but also enhances the outcomes of your analysis.
By mastering data handling techniques, users can elevate their skills in SPSS, leading to more accurate interpretation of data and informed decision-making.
Exploring Basic Statistical Functions
Understanding basic statistical functions is pivotal in employing SPSS effectively. These functions serve as the foundation for any analytical work performed in the software. By mastering these basics, users can unlock deeper insights from data sets. This section discusses essential elements including descriptive statistics, inferential statistics, and the methods for assessing data distributions. Such knowledge ensures accurate results and enhances decision-making in various fields, making it invaluable for professionals and students alike.
Descriptive Statistics
Descriptive statistics summarize and describe the features of a data set. They provide key insights into central tendencies, variability, and data distribution. Common descriptive statistics include mean, median, mode, range, and standard deviation. Each of these plays a specific role:
- Mean gives the average value.
- Median indicates the middle value when data is ordered.
- Mode reveals the most frequently occurring value.
- Range represents the difference between the highest and lowest values.
- Standard deviation measures data dispersion from the mean.
Descriptive statistics are essential for grasping basic patterns in data. Knowing the distributions and variability helps users recognize trends and anomalies, thus providing a clearer framework for further analysis.
Inferential Statistics
Inferential statistics allow users to make predictions or inferences about a larger population based on a sample. Techniques such as hypothesis testing, confidence intervals, and regression analysis fall into this category. These methods rely on probability theories and are crucial for validating the significance of findings in research. For instance:
- Hypothesis testing helps determine if there is enough evidence to support a specific claim.
- Confidence intervals provide a range of values capturing the true population parameter, fostering better insights.
- Regression analysis identifies relationships between dependent and independent variables.
Implementing inferential statistics equips users to draw conclusions and predict future outcomes, thus facilitating informed decision-making in various domains.
Assessing Data Distributions
Assessing data distributions is a critical step in any data analysis process. Understanding the shape of the data and how it behaves helps in choosing the right statistical methods. Key concepts include:
- Normal distribution: Data is symmetrically spread around the mean.
- Skewness: Measures the asymmetry of the distribution. A positive skew indicates longer tails on the right, while a negative skew shows longer tails on the left.
- Kurtosis: Indicates the steepness of the distribution. High kurtosis means more outliers, while low kurtosis suggests a flatter distribution.
Understanding distribution effectiveness is essential in statistical analyses, as it influences the selection and accuracy of statistical tests.
By utilizing SPSS to assess these distributions, users gain valuable insights into how their data behaves. This understanding is critical when reporting results and conducting further analyses.
Advanced Analytical Techniques
Advanced Analytical Techniques are crucial in attaining deeper insights from datasets, making them an integral part of statistical software like SPSS. These techniques go beyond basic statistics, providing the ability to analyze complex relationships and interactions among variables. They offer benefits such as enhanced prediction capabilities, improved decision-making, and a better understanding of underlying patterns within data. As practitioners in software development and IT-related fields, utilizing these advanced methods will significantly increase the rigor of your analyses.
Regression Analysis
Regression Analysis is a method used for modeling the relationship between one dependent variable and one or more independent variables. It is essential because it allows analysts to determine how changing one variable affects another. In SPSS, regression functions include multiple regression, logistic regression, and more. The main benefit here is the capacity to make informed predictions based on historical data.
Considerations when performing regression analysis in SPSS include:
- Properly selecting independent variables to avoid issues like multicollinearity.
- Checking assumptions, such as linearity and homoscedasticity, which are vital for valid results.
- Evaluating model fit through measures like R-squared.
ANOVA Procedures
ANOVA, or Analysis of Variance, is a statistical technique used to compare means across different groups. It can determine if at least one group mean is significantly different from the others. This is particularly important for comparing different categories in data that influence outcomes.
In SPSS, conducting ANOVA is straightforward, involving:
- One-way ANOVA for single factors affecting a dependent variable.
- Two-way ANOVA for examining interactions between two independent variables.
Key benefits of using ANOVA include identifying group differences without inflating the risk of Type I errors that occur in multiple t-tests. However, it is crucial to check for homogeneity of variances and consider post-hoc tests to delve deeper into group differences.
Factor Analysis
Factor Analysis involves identifying underlying relationships between variables. It is particularly useful for reducing data complexity and identifying latent constructs that explain the observed correlations between variables.
In SPSS, factor analysis can be employed through:
- Principal Component Analysis (PCA), which identifies the main components.
- Exploratory Factor Analysis (EFA), which helps reveal the structure of data without preconceived notions.
The main considerations with factor analysis involve:
- Determining the number of factors to extract, which can significantly impact interpretations.
- Assessing the adequacy of sample size and the appropriateness of the dataset.
Utilizing these advanced analytical techniques will enhance your ability to derive insights from data efficiently and effectively, ultimately leading to more robust findings and conclusions in your analytical endeavors.
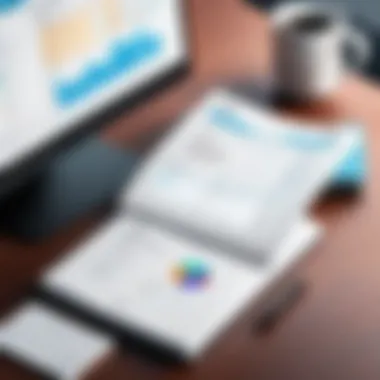
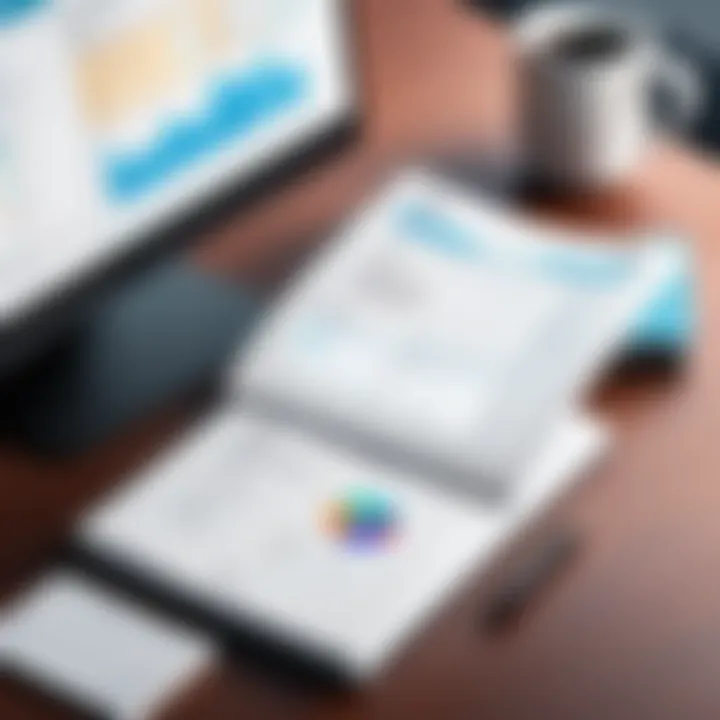
Visualizing Data
Visualizing data is a crucial step in any data analysis process. It helps to transform complex numerical data into easily understandable graphical representations. Through visualization, patterns and trends can be identified, making it easier to communicate insights to others. SPSS provides a variety of tools for visualizing data, enabling users to create effective representations of their dataset.
Creating Graphical Representations
Creating graphical representations is one of the primary functions of SPSS. Users can generate bar charts, histograms, scatter plots, and pie charts to illustrate data findings. Each type of graph serves a specific purpose. For example:
- Bar Charts are ideal for comparing discrete categories.
- Histograms assist in understanding the distribution of a continuous variable.
- Scatter Plots allow for the observation of the relationship between two variables.
Using these visual tools, data analysts can highlight significant findings that may not be obvious from numerical data alone. Additionally, graphs can be customized in SPSS to suit the audience's preferences, ensuring clarity and precision in communication.
Using Charts and Plots Effectively
To utilize charts and plots effectively, it is essential to understand the context and audience. Avoid cluttered visuals that may confuse the audience. Instead, strive for clarity and focus by selecting the most relevant data points. Also, think about the story behind the data. What are the key messages to convey? For example:
- When presenting survey results, bar charts may be most effective.
- For showing correlations, scatter plots can illustrate how two variables relate to each other.
Always consider color choices and labels. Good labeling and a thoughtful color palette enhance the readability of charts and plots. Well-designed visuals speak directly to the viewer, guiding them to interpret the data without excessive explanation.
Customizing Visual Outputs
Customization of visual outputs can significantly impact how well data is understood. In SPSS, there are various options for adjusting elements of your charts and graphs. Here are some features to consider:
- Color Schemes: Choose colors that resonate with your audience, ensuring they are easy to distinguish.
- Fonts and Sizes: Proper font selection and sizing improve readability.
- Legends and Titles: Clear titles and legends prevent confusion about what the visual represents.
"A well-customized graph removes barriers to understanding data, making insights accessible to all stakeholders."
By paying attention to these details, users can create visuals that not only inform but also engage their audience, driving home the significance of the data presented. Properly visualized data can lead to better decision-making and encourage informed discussions about findings.
Utilizing Syntax for Efficiency
Utilizing syntax within SPSS offers a level of efficiency that can significantly enhance the data analysis process. While SPSS provides a graphical user interface (GUI) that is user-friendly, mastering syntax can streamline repetitive tasks, reduce errors, and improve productivity for those who use the software regularly. Learning to effectively use SPSS syntax is not only beneficial for data manipulation but also empowers users to execute complex analyses with precision and speed.
Preamble to SPSS Syntax
SPSS syntax consists of a structured command language that allows users to perform data analysis operations through written code. This command language provides a way to access functions and features that may not be fully exposed in the graphical interface. By using syntax, users can automate repetitive tasks, which is especially useful in situations where the same analyses need to be conducted across multiple datasets. Additionally, syntax files can be saved and reused, providing a valuable resource for future projects.
Writing Your Own SPSS Commands
Writing SPSS commands involves understanding the basic structure of syntax, which typically consists of a command, followed by options and subcommands. For instance, a command might look like this:
In this case, the command 'FREQUENCIES' generates frequency tables for the specified variables. Users can customize commands to suit their data needs, making it essential to familiarize oneself with SPSS command syntax. The SPSS Command Reference Guide is a useful tool for understanding the detailed function of each command.
Benefits of Syntax Over GUI
The advantages of using syntax over GUI in SPSS include:
- Efficiency: Syntax is faster for executing multiple commands compared to repetitive clicks in a GUI.
- Reproducibility: Syntax ensures that analyses can be replicated exactly, which is crucial for scientific research.
- Documentation: Written commands serve as a clear record of the analysis performed, aiding both interpretation and communication of findings.
- Complex Operations: Some analyses may require options not available through the GUI. Syntax allows users to leverage the full capabilities of SPSS.
"Learning to write SPSS syntax can transform your approach to data analysis. It reduces time spent in manual input, allowing for more focus on interpretation and insights."
Practical Applications of SPSS
Understanding practical applications of SPSS is crucial for those aiming to use it effectively in real-world situations. This section explores how SPSS can assist in various fields, emphasizing its versatility and the insights it can provide. Practitioners, researchers, and analysts can harness SPSS to draw meaningful conclusions from their data. The benefits of skillful usage are significant, impacting decision-making and strategy development.
Case Studies in Various Fields
SPSS has been applied in diverse fields such as healthcare, education, marketing, and social sciences. For instance, in healthcare, it is used to analyze patient data to improve treatment efficacy. Decision-makers can evaluate the success of different medical procedures using statistical methods available in SPSS. In education, SPSS helps analyze student performance, revealing trends that can drive curricular changes.
In marketing, organizations use SPSS to analyze customer behavior. Companies can segment their customers based on purchasing patterns, enabling targeted marketing campaigns. Social scientists employ SPSS to analyze survey data, which offers insights into public opinion and social phenomena. These case studies exemplify the efficacy of SPSS across sectors, highlighting its relevance.
Using SPSS in Academic Research
Academic research heavily relies on rigorous data analysis to validate hypotheses. SPSS offers tools specifically tailored for researchers to conduct statistical tests, manage datasets, and visualize results. By utilizing SPSS, researchers can achieve reproducibility and accuracy in their findings.
In performing meta-analysis, researchers can combine results from multiple studies, increasing the robustness of their conclusions. SPSS provides advanced functionalities for this kind of statistical work, allowing for detailed evaluations of existing literature.
Furthermore, SPSS enhances collaboration among researchers. By exporting SPSS output to common formats, findings can be shared with peer reviewers or collaborators efficiently. This exposure can lead to valuable feedback, improving the overall quality of research output.
Employing SPSS in Business Analytics
In the realm of business analytics, SPSS is a powerful tool for informed decision-making. It equips businesses with the ability to analyze sales trends, customer feedback, and market research data. Understanding these metrics is vital for developing effective business strategies.
Additionally, SPSS supports risk analysis and forecasting. Companies can predict future trends based on historical data, which helps in planning and resource allocation. For example, a retail business can use SPSS to analyze seasonal sales data, allowing it to stock inventory effectively during peak times.
Moreover, companies leverage SPSS to evaluate employee performance metrics. Businesses can identify factors contributing to employee satisfaction and productivity, crafting policies that benefit both the company and its workforce.
Proper application of SPSS transforms data into actionable insights, driving innovation and efficiency.
Resources for Further Learning
In the realm of statistical software, mastering SPSS requires more than just hands-on practice. It involves a solid understanding of the theoretical underpinnings as well as the practical applications. Learning resources play a crucial role in this journey. They assist learners in bridging knowledge gaps and enhancing their proficiency.
Utilizing a variety of learning materials is beneficial. Each type offers unique advantages that can cater to different learning styles. Recommended textbooks and guides provide foundational knowledge. Online courses and tutorials present structured learning paths. Community forums and support foster exchange of ideas and troubleshooting. Together, these resources offer a comprehensive arsenal for effective learning.
"Education is the most powerful weapon which you can use to change the world."
Recommended Textbooks and Guides
Textbooks are essential for in-depth knowledge of SPSS. They often break down complex concepts into manageable segments. Choosing the right textbook can significantly impact learning efficiency. Some notable titles include:
- "SPSS for Beginners" by Daniel J. Steinberg, which lays a strong foundation for new users.
- "Discovering Statistics Using SPSS" by Andy Field, known for its practical approach and clarity in explanations.
- "Applied Multivariate Statistical Analysis" by Richard A. Johnson, which serves advanced users looking to expand their understanding.
These texts often include practical examples, exercises, and valuable insights into interpreting data.
Online Courses and Tutorials
Online courses present structured learning opportunities. Platforms like Coursera, Udemy, and LinkedIn Learning offer various SPSS courses, often with video lectures and interactive components. This format is advantageous for visual learners and allows for flexibility.
Tutorials on platforms such as YouTube also offer an informal yet informative way to learn specific functionalities. For instance, creators often share step-by-step guides on conducting specific analyses or troubleshooting common issues. These resources not only educate but also engage learners in diverse ways.
Community Forums and Support
Engaging with peers and experts enhances the learning experience. Community forums such as Reddit and specialized SPSS user groups on Facebook provide platforms to ask questions and share experiences.
Benefits of participating in these forums include:
- Problem-solving through collective knowledge and experience.
- Networking opportunities with professionals in the field.
- Access to real-world applications and insights from diverse users.
Moreover, these forums can be a source of continual support, ensuring that learning does not stop after initial mastery. Collaboration in these environments can lead to greater understanding and innovative approaches to using SPSS.
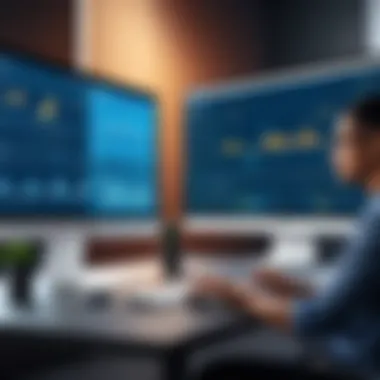
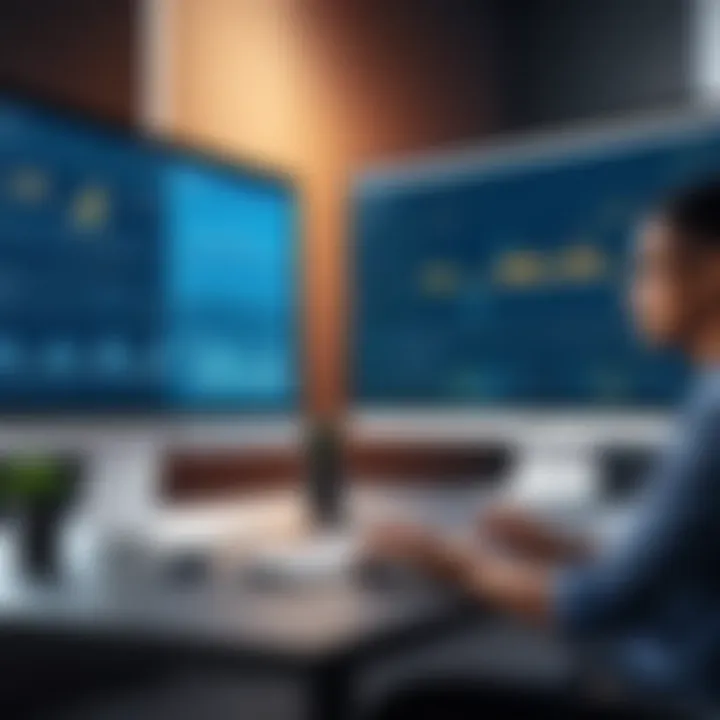
Troubleshooting Common Issues
In any software package, troubleshooting is vital. SPSS is no exception. This section addresses common problems users might face. Understanding these issues helps maintain workflow and improves user experience. Proficiency in resolving these matters can save time, reduce frustration, and enhance the use of SPSS for data analysis.
Resolving Data Import Errors
Data import errors can hinder the start of data analysis. When you cannot import your dataset, the consequences can be severe. This problem can stem from various sources such as incompatible file formats or corrupted files.
To resolve these issues:
- Check File Format: Ensure the file is in a format compatible with SPSS, like .sav, .csv, or .xls.
- Examine File Integrity: Open the file in its source application to check for corruption or formatting issues.
- Use Import Wizard: SPSS’s built-in Import Wizard aids in troubleshooting issues during import. Follow the prompts carefully.
If errors persist, examining error messages in detail can provide clues. You may also find help in SPSS user forums.
Debugging Syntax Problems
Syntax errors can be especially frustrating when they disrupt your scripts. Writing SPSS commands requires precision. A single typo or misplaced command can result in an error.
To debug syntax issues:
- Review Code Line by Line: Check for unclosed quotes, extra spaces, or incorrect punctuation.
- Use the Syntax Editor: SPSS provides a Syntax Editor that can highlight errors in real time. Use it wisely.
- Search for Documentation: SPSS documentation often provides advice specific to recognized syntax errors.
Adjusting your approach to syntax writing can improve your effectiveness. Coding becomes smoother with practice and attention to details.
Handling Output Display Issues
Output display issues can obscure results and confuse analysis. SPSS generates reports, graphs, and tables. If these do not display correctly, the interpretation becomes difficult.
To address these problems:
- Check Display Settings: Sometimes, settings in the output window may need adjustments. Verify they are set to default.
- Look for Updates: Running an outdated version of SPSS can lead to display problems. Ensure your software is up-to-date.
- Consult User Forums: Many users share their experiences with output problems. Exploring discussions on forums, such as reddit.com, can provide insights.
"To troubleshoot effectively, maintain documentation of issues faced and solutions applied. This can be a valuable resource for future reference."
By systematically addressing common issues in SPSS, users can navigate obstacles with greater ease. This ultimately contributes to a more productive analytical environment.
Collaborating with Others
In today's data-driven world, collaboration is key to efficient data analysis. When using SPSS, the ability to collaborate effectively with others can enhance productivity and lead to more accurate results. This section delves into the specific elements, benefits, and considerations when working alongside peers and colleagues in the SPSS environment.
Sharing SPSS Files
One major aspect of collaboration in SPSS is file sharing. SPSS files, which can include data sets, output files, and syntax, are essential for team projects. Properly sharing files ensures that all team members are on the same page and have access to the latest data and analyses.
Considerations for file sharing:
- File Format: SPSS allows saving files in formats such as .sav, .spv, and .sps. Understand which format is suited for your team’s needs.
- Access and Permissions: Ensure that file access levels are appropriate to protect sensitive data. Consider using shared drives or cloud services.
- Documentation: When sharing files, provide clear documentation. Explain any unique variables or syntax that others might not easily understand.
These considerations facilitate smoother workflows and reduce miscommunication among collaborators.
Working on Shared Projects
Collaborating on shared projects in SPSS can lead to richer analyses and innovative insights. Each team member can contribute their unique skills, whether it's data cleaning, statistical analysis, or visualization.
Key functionalities for shared projects include:
- Assigning Roles: Define roles for each team member based on their strengths. This specialization helps in managing the workload more efficiently.
- Communication Tools: Use software such as Slack or Microsoft Teams alongside SPSS for effective communication. Discuss methods and results in real-time.
- Regular Updates: Schedule regular meetings to discuss progress. Sharing findings prevents duplication of effort and allows for collective problem-solving.
These strategies can lead to a more organized and successful project completion, enhancing learning experiences for everyone involved.
Version Control Strategies
In collaborative environments, it's crucial to have a solid version control strategy. As multiple users work on the same SPSS files, tracking changes becomes difficult without proper management. Here are some strategies to consider:
Effective version control strategies include:
- Naming Conventions: Consistently name files to indicate the version and contributor. This practice helps identify newer files and reduces confusion.
- Change Logs: Maintain logs detailing changes made to files. This log should include the date, author, and nature of the changes.
- Backup Procedures: Regularly back up SPSS projects. Setting up automated backups to cloud storage can protect against data loss.
By implementing these version control strategies, teams can work more cohesively without losing track of their progress or introducing errors into their analyses.
Collaboration in SPSS not only improves the work done but also enhances individual learning by exposing team members to different perspectives.
Overall, effective collaboration using SPSS enhances the analytical process. It promotes shared knowledge, prevents errors, and ultimately leads to better and more insightful data analysis.
Staying Updated with SPSS
Remaining current with SPSS is critical for anyone who seeks to enhance their analytical capabilities. As users navigate through data problems, they need the most reliable tools and features. Software updates frequently addresses bugs, introduces new capabilities, and refines existing features. This ensures that users can perform their tasks efficiently and effectively. Moreover, industry trends evolve and adapting SPSS accordingly can give users a competitive edge.
Understanding Software Updates
SPSS software updates often patch bugs that exist in earlier versions. These updates may also come with enhancements that improve performance and security. Regular updates guarantee that users have access to the latest statistical techniques and methodologies. Ignoring updates could lead to compatibility issues, especially with newer data formats. Therefore, understanding how to install updates ensures smoother operations in data analysis.
Leveraging New Features
New features in SPSS can elevate analysis by simplifying complex tasks. Users should take the time to explore what’s new, as features are frequently added based on user feedback. For instance, enhanced graphical capabilities might make it easier to visualize data. A feature may also streamline the data cleaning process or enhance command syntaxes. Leveraging these tools means utilizing SPSS to its full potential, which ultimately improves the quality of analysis conducted.
Following Industry Trends
Staying in tune with industry trends is equally important. The landscape of data analysis is constantly evolving, with new methodologies and practices emerging. For example, topics like machine learning or big data analytics are gaining traction. Understanding these movements can adjust how SPSS is used. Finding online resources, such as community forums or educational platforms, can help uncover new methods to employ SPSS effectively. This ongoing education is essential to remain relevant in any field involving data.
"Continuous learning is the minimum requirement for success in any field."
Keeping SPSS updated, learning about new features, and following industry trends is a pathway to mastering data analysis with SPSS. Users should approach updates not just as maintenance tasks, but as opportunities for growth.
End
Drawing conclusions in any analytical endeavor is crucial, and the same applies to utilizing SPSS. The conclusion of this article synthesizes key elements discussed throughout, reinforcing the relevance of effective learning strategies in mastering SPSS. It emphasizes the importance of solidifying knowledge gained from the various sections, from navigating the user interface to implementing advanced analytical techniques.
The benefits of these conclusions extend beyond merely summarizing the topics. They encourage readers to harness their newfound skills through practical application in real-world scenarios. This creates an avenue for improved confidence and proficiency with statistical analysis software, which is essential for career advancement in data-oriented fields.
In today’s data-driven environment, the ability to draw accurate conclusions from analyzed data can significantly impact decision-making processes. SPSS provides the tools necessary for this analysis, and understanding how to apply them effectively sets a solid foundation for continuous learning and application.
"Learning is a journey, not a destination."
This quote reminds us that becoming adept in SPSS is a continuous process that involves regularly revisiting concepts learned and applying them in varied circumstances.
Recap of Learning Highlights
The journey through this guide has highlighted several critical aspects of SPSS. Key points include:
- User Interface Navigation: Mastering different views within SPSS simplifies data manipulation and analysis.
- Statistical Functions: A thorough understanding of both descriptive and inferential statistics enhances decision-making based on empirical data.
- Data Handling: Techniques for importing, cleaning, and transforming data are foundational skills that support accurate analysis.
- Syntax Use: Leveraging SPSS syntax allows for improved efficiency and repeatability in analyses.
Reflecting on these highlights allows users to identify their strengths and areas needing further development.
Emphasizing Continuous Improvement
Continuous improvement is essential in any learning journey, especially with a complex tool like SPSS. Regular practice and exploration of new features and techniques help maintain skills and adapt to evolving requirements in data analysis. Here are some strategies for continuous growth:
- Engage with Community Forums: Participating in platforms such as Reddit or Facebook groups can offer insights and solutions to common challenges faced by SPSS users.
- Online Resources: Taking advantage of online courses and tutorials provides additional learning opportunities and updates on new methodologies.
- Real-World Projects: Applying knowledge gained through this guide in practical situations reinforces learning and helps develop a portfolio that demonstrates proficiency.
Continuous improvement forms the backbone of mastery in SPSS. Practical application, reflection, and engagement with the broader community serve to enhance one’s skill set, promoting growth and expertise in statistical data analysis.