Top Data Analysis Software Reviewed for 2023
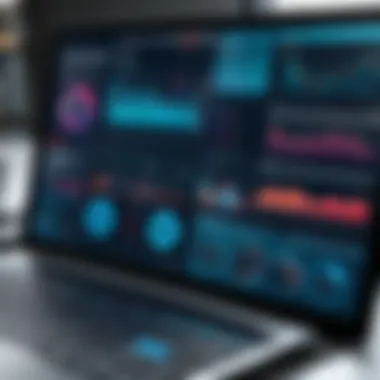
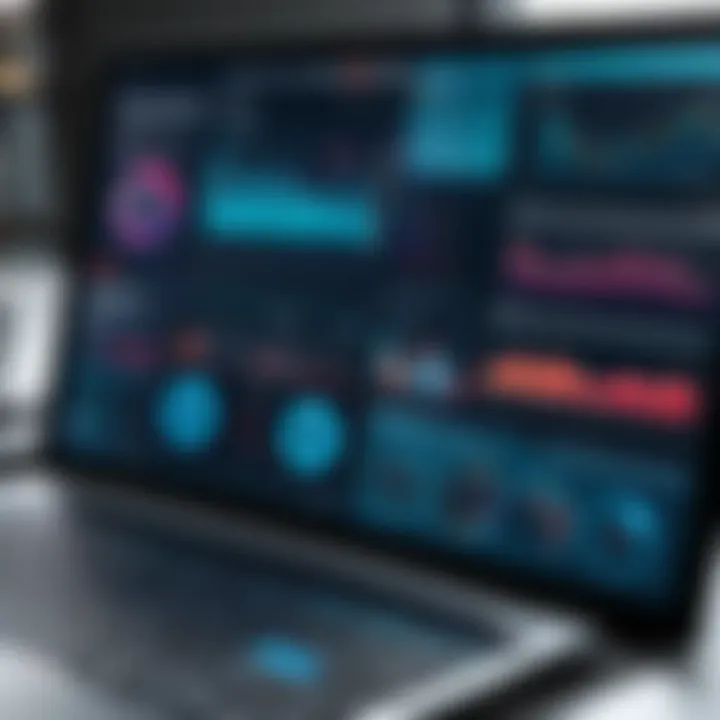
Intro
Data analysis has become a cornerstone of decision-making across various sectors. With the vast volumes of data generated daily, the need for effective software that can analyze, visualize, and interpret this data is essential. The landscape of data analysis software is incredibly diverse, catering to different needs and levels of expertise. This article aims to provide a comprehensive overview, guiding users through the essential features, usability, pricing, and compatibility of leading tools in the field.
In this review, we will dissect the variety of software available, indicating which tools best serve particular purposes. Whether you are a seasoned analyst or just stepping into the world of data, understanding these tools can elevate your capabilities and enhance your data-driven decisions.
Overview of Software
Purpose and Use Cases
Data analysis software serves multiple purposes. It allows users to clean, manipulate, and analyze data efficiently. Some common use cases include:
- Business Intelligence: Organizations use software like Tableau and Microsoft Power BI to generate reports and gain insights that drive strategic decisions.
- Statistical Analysis: Tools such as R and SAS are designed for in-depth statistical computations and are often employed in academic or research settings.
- Data Visualization: Visualization tools present complex data graphs and charts, enhancing understandability that supports narratives in presentations.
- Machine Learning: Software like Python's scikit-learn and TensorFlow enables analysts to build predictive models based on historical data.
Each tool fills a unique niche that appeals to varied audiences, from data scientists to executives needing quick insights.
Key Features
The effectiveness of data analysis software often hinges on several key features:
- Data Connectivity: The ability to connect with various data sourcesโdatabases, spreadsheets, and cloud storageโenhances the software's utility.
- User-Friendly Interface: An intuitive UI can make a significant difference. Software with clear layouts and accessible functionalities is easier to navigate, especially for novices.
- Automation Capabilities: Automated data processing and reporting capabilities streamline workflows, reducing manual intervention and errors.
- Collaboration Tools: Integrated features that facilitate sharing insights among teams foster collaboration and collective decision-making.
Prioritizing these features helps users choose the right software that aligns with their data analysis needs.
In-Depth Review
Performance Analysis
Performance is another chief concern when evaluating data analysis software. Metrics related to speed, load times, and processing capability vary widely. High-performance tools can handle significant amounts of data without compromising efficiency. Considerations here include:
- Scalability: As data grows, the software should adapt without a decline in performance.
- Processing Speed: Fast computation times contribute to a smoother user experience. Companies often favor software that minimizes downtime.
User Interface and Experience
User experience drives software adoption. A well-designed interface can lower the learning curve and enhance productivity. Key considerations include:
- Usability Layout: Clear menus and categorization of features simplify the analysis process.
- Interactive Visualizations: Users appreciate tools that allow manipulation of data graphs in real-time, leading to better insight generation.
A positive user experience significantly impacts the effectiveness of data analysis tools, promoting efficient engagement and deeper learning.
In closing, the scope of data analysis software is broad and continually evolving. Each tool offers unique advantages tailored to different user needs. Understanding these distinctions can profoundly impact how organizations and individuals interpret their data, leading to more informed and strategic decisions.
Prelims to Data Analysis Software
The significance of data analysis software in today's world cannot be overstated. Businesses across sectors are increasingly reliant on data to drive decision-making. The right tools can empower organizations to glean insights from vast datasets, enhancing efficiency, performance, and profitability. This section delves into the relevance of data analysis software and its critical role in enabling deeper understanding and strategic foresight in business contexts.
Importance of Data Analysis in Modern Business
In the contemporary business landscape, data analysis has become a cornerstone of organizational success. Companies that harness data are better positioned to understand market trends, customer preferences, and operational inefficiencies. This understanding leads to informed decision-making that contributes to competitive advantage.
- Informed Decisions: Businesses can make choices based on empirical evidence rather than intuition. This mitigates risks and optimizes outcomes.
- Customer Insights: Data analysis reveals customer behavior patterns, enabling personalized marketing strategies that enhance customer engagement.
- Operational Optimization: Through data scrutiny, organizations can identify bottlenecks and streamline processes, saving time and resources.
- Market Trends: The capability to analyze data trends helps businesses anticipate market shifts, allowing for proactive rather than reactive strategies.
Overall, data analysis directly influences capacity for growth and sustainability in modern businesses.
Overview of Data Analysis Software
Data analysis software comprises tools designed to process, analyze, and visualize data. These systems help data professionals manage complex datasets, execute statistical analyses, and draw meaningful conclusions. With the growth of big data, the market for such software has evolved to include a diverse range of solutions.
Key considerations when looking at data analysis software include:
- Functionality: The ability to support various analytical methods and techniques, from simple descriptive statistics to complex predictive modeling.
- Compatibility: Integration with existing software systems ensures a seamless workflow. A lack of compatibility can lead to inefficiencies and data silos.
- Support for Programming Languages: Many advanced tools support programming languages like R or Python, expanding what users can accomplish in analysis.
In sum, having a solid grasp of available data analysis software is essential for making informed decisions about the appropriate tools for specific business needs. As data continues to proliferate, proficiency in these software solutions becomes increasingly important.
Key Features to Look for in Data Analysis Software
When selecting data analysis software, it is paramount to understand the key features that significantly contribute to its effectiveness. This section elucidates crucial aspects that the user needs to consider. Understanding these features allows professionals to make judicious choices that cater to their specific analysis requirements.
Data Visualization Capabilities
Data visualization is a vital feature of any robust data analysis software. This capability enables users to present complex data in a visual format. Charts, graphs, and dashboards help in deciphering patterns and trends in data that are often difficult to identify in raw numbers. For example, Tableau is recognized for its intuitive drag-and-drop interface which simplifies the creation of interactive visualizations. Users should look for software that supports a variety of visualization types, ensuring inclusivity of diverse datasets. Also, consider if the software allows for customization to match the requirements in reporting or presentation.
Integration with Other Tools
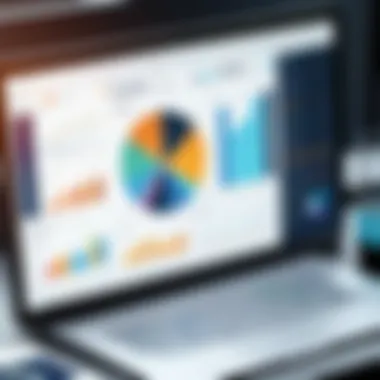
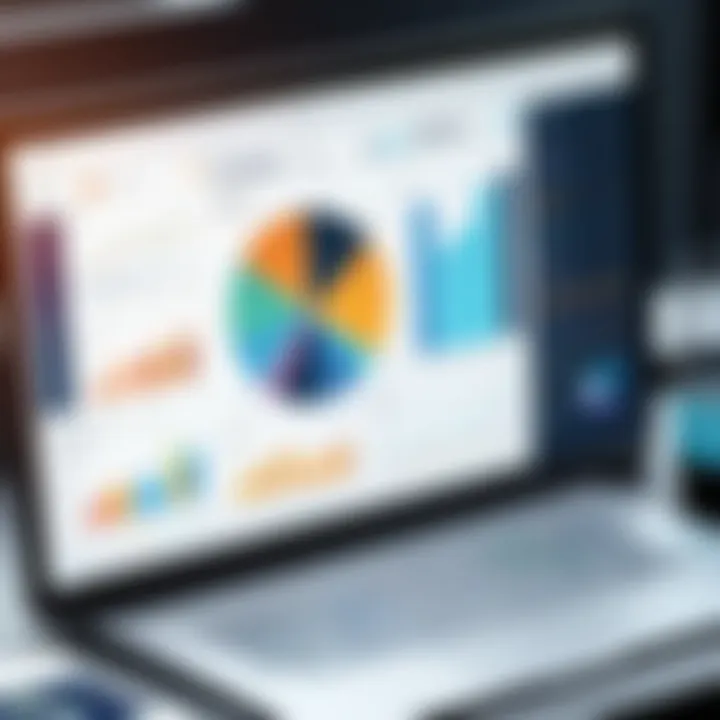
Integration is key in maximizing data analysis efficiency. The ability to synchronize with other tools and platforms can greatly enhance productivity. Software like Microsoft Excel integrates easily with various business intelligence tools and databases. This compatibility fosters a seamless workflow, allowing analysts to combine insights from multiple sources without unnecessary manual intervention. Therefore, check if the software can connect with existing systems like CRM or ERP systems, as this can streamline processes and improve data accuracy.
User-Friendly Interface
A user-friendly interface is essential, especially for those who may not possess advanced technical skills. Software like RStudio has made strides in providing intuitive layouts that accommodate both beginners and experts alike. The interface should not only be aesthetically pleasing but also functionally straightforward. It is important to evaluate the learning curve associated with the software. A steep learning curve can deter effective use, leading to frustration and inefficiencies. Ideally, the software should offer tutorials or robust support communities to assist users in navigating its features efficiently.
"Choosing data analysis software with key features tailored to your needs will enhance your productivity and insights."
In summary, when evaluating data analysis software, focus on data visualization capabilities, integration options, and user-friendly interfaces. These features are critical in ensuring effective analysis and interpretation of data, which ultimately drives informed decision-making.
Top Data Analysis Software Solutions
In the world of data analysis, selecting the right software solution is crucial. Various tools exist, each offering different capabilities and characteristics. This section will explore some of the most noted data analysis software, focusing on their key features, strengths, and limitations. Understanding these tools helps businesses and analysts choose the right one according to their needs.
Microsoft Excel
Key Features
Microsoft Excel is a widely acknowledged tool for data analysis thanks to its spreadsheet functionality. One notable feature is its pivot tables, which simplify data summarization. Excel supports various formulas and functions that facilitate complex calculations. Its integration with other Microsoft Office tools greatly enhances its usability for analysts.
Strengths
One strength of Excel is its user-friendly interface. Many users are already familiar with it, making the learning curve less steep. Excel offers powerful data visualization options, such as charts and graphs, allowing for clear representation of data. Additionally, it supports many data formats, which adds to its versatility in various business contexts.
Limitations
However, Excel does have limitations. It may struggle with large datasets, making it less effective for big data analysis. Furthermore, collaboration can be cumbersome, especially when multiple users need to edit the same file simultaneously. This restricts its application in some collaborative environments.
Tableau
Key Features
Tableau is renowned for its robust data visualization capabilities. Users can create interactive dashboards which allow stakeholders to explore data dynamically. It enables connections to various data sources, providing flexibility in analysis and reporting.
Strengths
The strength of Tableau lies in its visual appeal and intuitive drag-and-drop functionality. This feature enables users to create complex visualizations without extensive technical knowledge. Furthermore, Tableauโs ability to handle large volumes of data is impressive, making it suitable for organizations working with big datasets.
Limitations
Despite its advantages, Tableau is not without limitations. Its price can be prohibitive for smaller businesses or individual users. Additionally, some users may find that advanced features require a significant amount of time to learn properly, which could delay insights and decision-making processes.
R and RStudio
Key Features
R is a powerful programming language for statistical analysis, commonly used with RStudio as its integrated development environment. R supports numerous packages that extend its functionality for a wide range of statistical methods, making it highly flexible and adaptable for different analyses.
Strengths
One of R's key strengths is its statistical power. It enables users to perform sophisticated analyses, and its open-source nature means that users have access to a massive community and plethora of resources. R also excels in producing publication-quality visualizations, which can be critical for professional presentations.
Limitations
Nevertheless, R has a steep learning curve, particularly for those who are not already familiar with programming. Its speed may also be a concern when handling very large datasets, compared to other software solutions tailored specifically for big data applications.
Python for Data Analysis
Key Libraries
Python has gained popularity for data analysis largely due to its rich set of libraries. Libraries like Pandas and NumPy are essential for data manipulation and analysis. Matplotlib and Seaborn are favorable for visualizations, allowing for great flexibility and creativity in data presentation.
Strengths
The main strength of using Python is its versatility and ease of integration with web applications and databases. Python is also recognized for its support in machine learning, which is increasingly relevant as organizations seek to adopt more advanced analytic strategies.
Limitations
However, Pythonโs performance with large datasets can be suboptimal without additional optimization. Furthermore, not all libraries are beginner-friendly, which may pose a challenge for new users in the field.
SAS
Key Features
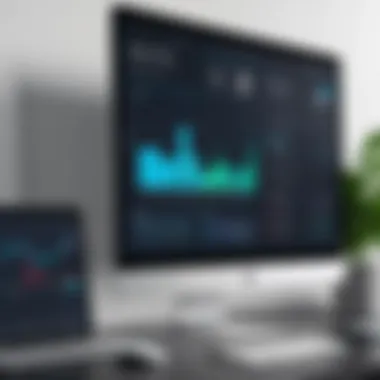
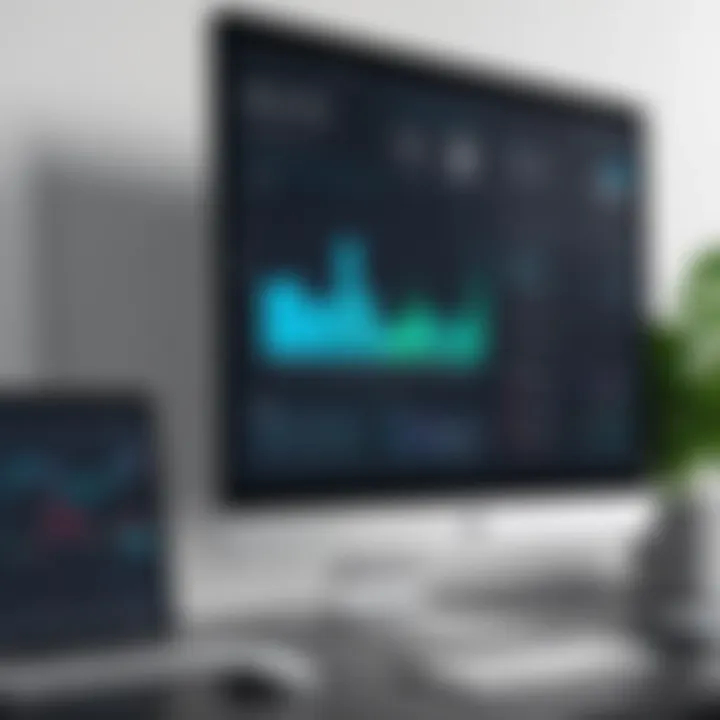
SAS offers a wide array of powerful analytical functions. It is designed for advanced analytics, business intelligence, and data management. Its built-in data manipulation capabilities can handle extensive datasets effectively.
Strengths
SAS is strong in its customer support and comprehensive documentation. This makes it a strong choice for enterprise applications. Additionally, it offers solutions that comply with industry regulations, which is beneficial for sectors that require stringent data governance.
Limitations
However, SAS comes at a higher price point, making it less accessible for small businesses. It also has a steeper learning curve for users who are not already trained in SAS programming.
Overall, the choice of data analysis software can significantly impact workflow efficiency and output quality. It depends greatly on specific user needs, budget constraints, and the complexity of the data being analyzed.
Criteria for Selecting Data Analysis Software
Selecting the appropriate data analysis software is a critical process that can significantly impact the effectiveness of data-driven decisions in any organization. The landscape of data analysis tools is vast and diversified. Without a clear set of criteria, businesses may find themselves investing in solutions that do not meet their specific analytical requirements. This section addresses essential criteria that should be thoroughly evaluated before making a choice. Understanding your organization's unique demands, budget limitations, and anticipated growth is foundational.
Assessing Specific Business Needs
Before diving into the sea of available data analysis tools, it is vital to first assess the specific needs of your business. Every organization has unique processes, data types, and analytical goals. Identifying these elements can help narrow down options effectively.
Engaging in a detailed exploration of your current workflows and desired outcomes is essential. Ask critical questions:
- What types of data do we primarily work with?
- Are we looking for basic analytical capabilities or advanced features, like predictive modeling?
- Who will be using this software, and what is their skill level?
Evaluating these factors can aid in selecting software that not only meets technical requirements but is also user-friendly for staff. The wrong software can complicate processes, leading to inefficiency and frustration.
Understanding Budget Constraints
Budget is often a deciding factor in the software selection process. Data analysis tools come in various pricing models, from free open-source options to high-cost enterprise solutions. Understanding the total cost of ownership is crucial. This includes not just the initial purchase price but ongoing costs for maintenance, additional features, or training.
Organizations must ask:
- What is our maximum budget for data analysis tools?
- Are there any hidden costs we need to be aware of?
- Will we require support services that may impact our total expenditure?
By having clear budget parameters, organizations can filter out tools that exceed financial capabilities while still finding effective solutions.
Evaluating Scalability and Flexibility
As businesses grow, their data needs can evolve. Therefore, it's critical to choose software that is not only suitable for current needs but also adaptable to future demands. Scalability is a fundamental aspect of this evaluation.
Considerations include:
- Can the software handle increased data volume as the organization expands?
- Will it accommodate additional users without significant performance loss?
- Are there options for adding features or modules in the future?
A flexible software choice enables smoother transitions and helps avoid costly replacement down the line. Not only does this help maintain continuity but also ensures the organization can respond promptly to changing data landscapes.
"Choosing the right data analysis software is not just about the features it offers, but how well it aligns with the evolving needs of the business."
In summary, evaluating specific business needs, understanding budget limitations, and considering scalability are essential steps in the software selection process. These criteria will guide organizations to make more informed choices that enhance their data analysis capabilities and ultimately lead to better decision-making.
Usability and Learning Curve
When evaluating data analysis software, usability and learning curve are critical factors. These aspects determine how effectively users can leverage the software to extract meaningful insights from data. A user-friendly tool can significantly shorten the time it takes for beginners to become proficient and allow advanced users to maximize their analytical capabilities. A solid understanding of usability and a manageable learning curve can enhance productivity and ensure that organizations derive better value from their data analysis efforts.
Ease of Use for Beginners
For those just starting in data analysis, ease of use is paramount. Beginners are often intimidated by complex interfaces that require a steep learning curve. Therefore, software that offers intuitive navigation and clear instructions can inspire confidence.
Some features that facilitate ease of use for novices include:
- Simple Interface: Clean and organized layouts help users locate tools and functions without overwhelming them.
- Tutorials and Resources: Effective tutorials and helpful resources such as forums or support documentation ease the onboarding process for new users.
- Drag-and-Drop Functions: Many data analysis tools now incorporate drag-and-drop functionalities, making it easier to manipulate data without extensive coding knowledge.
These elements contribute to a smoother transition into data analysis tasks while minimizing frustration and errors. As users become more comfortable with the software, they can gradually explore more advanced features, allowing for a natural progression in skill development.
Advanced Features for Expert Users
While ease of use is vital for beginners, advanced users often seek more complex functionalities to enhance their analysis capabilities. Data analysis software must strike a balance between simplicity for beginners and depth for experienced analysts. Advanced features may include:
- Scripting and Automation: Users proficient in programming can take advantage of built-in scripting capabilities to automate repetitive tasks, saving valuable time.
- Customizability: The ability to customize tools and create tailored workflows can significantly enhance productivity.
- Integration with Programming Languages: Software that integrates well with languages like Python or R enables experts to conduct sophisticated analyses and build predictive models using advanced statistical techniques.
Moreover, advanced features should not compromise usability. Well-designed software includes clear documentation for these functionalities, ensuring users can leverage advanced tools efficiently. This dual capability enriches the user experience and makes the software suitable for a wide range of analytical tasks across varying levels of expertise.
"A good data analysis tool empowers both novices and experts to make informed decisions based on data insights."
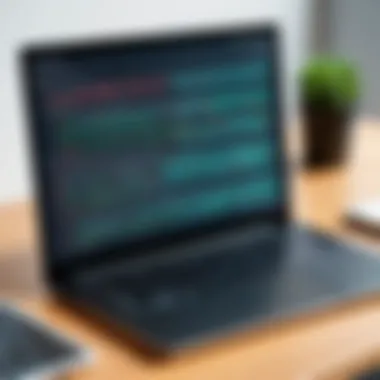
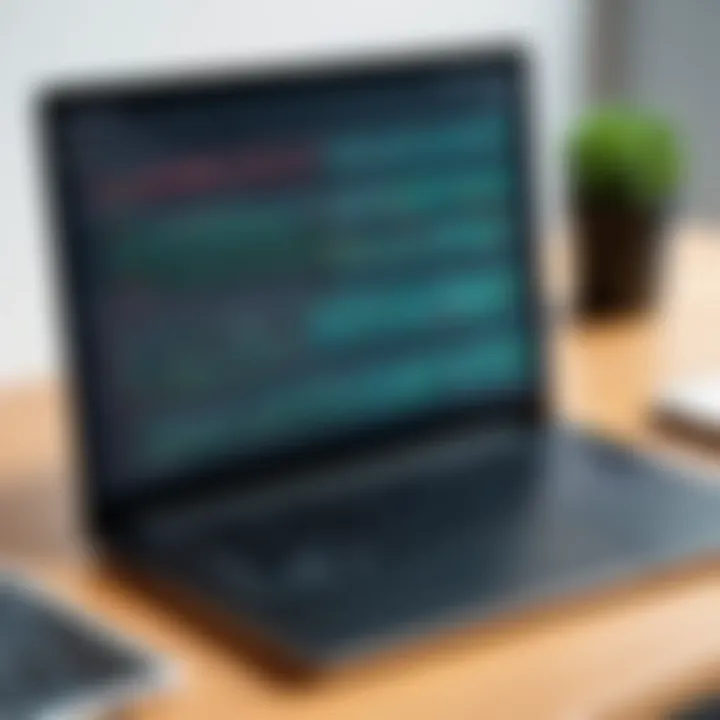
Usability and learning curve are not just features; they are essential components that dictate the effectiveness of data analysis software. By promoting ease of use for beginners while offering advanced functionalities for seasoned professionals, software can cater to a diverse audience and enhance overall productivity.
Comparative Analysis of Popular Data Analysis Tools
In the myriad of data analysis software available, a comparative analysis stands as a crucial element that guides users in selecting the most appropriate tool for their needs. This analysis allows users to make informed choices by examining key features, strengths, and limitations of various software solutions. Each tool often serves a different function and audience, highlighting the necessity for a thoughtful evaluation.
Considerations include functionality, integration capabilities, and user-friendliness. Moreover, a well-rounded comparison can illuminate aspects such as cost efficiency and scalability which are vital for organizations looking to grow. Evaluating the diverse data analysis tool options ultimately aids analysts and businesses in optimizing their workflows.
Feature Comparison Table
Creating a clear and effective feature comparison table can streamline the process of selecting data analysis software. This table presents side-by-side comparisons of key functionalities offered by different tools. By organizing attributes such as ease of use, visualization options, analytical capabilities, and support services, users can more readily identify which software aligns with their specific requirements.
Here is an illustrative example:
| Feature | Microsoft Excel | Tableau | R and RStudio | Python | SAS | | Data Visualization | Basic charts | Advanced visuals | Custom plots | Matplotlib, Seaborn | Advanced graphics | | Integration | Limited | High | Extensive libraries | Extensive libraries | Good | | Learning Curve | Moderate | Steep | Steep | Moderate | Moderate | | Price | Affordable | Premium | Free | Free | High | | User Community | Large | Growing | Extensive | Large | Niche |
This table is only a snapshot and should be extended with more detailed analysis as necessary.
Customer Feedback and Reviews
Customer feedback serves as an important metric in the evaluation of data analysis tools. Reviews can provide insight into real-world usability and satisfaction, offering potential users perspectives on their experiences.
Analysis of feedback often reveals valuable information regarding:
- Ease of onboarding: How quickly users can adapt to the software.
- Performance: Quality of results produced and speed of execution.
- Customer support: Availability and efficiency of user assistance.
- Community resources: Documentation and forums helping users troubleshoot issues.
Online platforms such as Reddit often feature discussions centered on these tools. Real conversations among users can reveal common pain points and highlights that formal reviews may overlook. Therefore, diving into customer reviews will yield a balanced view on how various data analysis tools serve their users.
Implementation and Support
The success of data analysis software hinges not just on its features but also on its implementation and support. After selecting a software tool, the way it is set up and the quality of support received can greatly influence its effectiveness in real-world applications. This section explores the critical aspects of implementation and support that professionals should consider to maximize their data analysis efforts.
Installation and Setup
Installing and setting up data analysis software can be a straightforward process or a complex task, depending on the tool. Many modern solutions are designed to streamline this phase for users. A guided installation process often enhances user experience, allowing seamless entry into the software's functionalities. Key aspects include:
- System Requirements: Each software has specific hardware and software prerequisites. Evaluating these requirements before installation is crucial for a smooth setup.
- User Interfaces: Many tools offer installation wizards that help navigate the process with step-by-step instructions. Familiarity with the interface can significantly speed up the initial setup.
- Documentation: Quality user manuals and online resources can reduce setup time. Comprehensive documentation should address common installation issues.
- Configuration Options: Post-installation, users may need to customize the tool. Options might include setting up preferences, connectivity with databases, or integrating with existing systems. Understanding these configurations is vital for optimal use.
In summary, paying attention to the installation and setup of data analysis software lays a strong foundation for successful utilization.
Customer Support Options
Even with well-designed software, challenges can arise during usage. This is where customer support plays a significant role. Individuals and organizations should examine a few critical elements regarding support options:
- Availability: Look for software providers that offer extensive support hours. Some companies maintain 24/7 support, which is particularly beneficial for businesses operating in different time zones.
- Channels of Communication: Diverse support channels, like phone, email, and live chat, can cater to varying user preferences. Quick response times in these channels signify a commitment to customer satisfaction.
- Community and Forums: Many popular tools have substantial user communities. Engaging with peers in forums like those on Reddit can provide insights not covered in official documentation. Users can share tips and solutions for common issues.
- Training Resources: Comprehensive training resources, such as webinars and tutorial videos, can empower users to tackle problems autonomously. This aspect of support is often underrated but can significantly reduce the dependency on direct customer support.
Future Trends in Data Analysis Software
The realm of data analysis software is constantly transforming. Recognizing trends that influence this field is crucial for users seeking tools that not only meet their current needs but also adapt to future demands. Future trends empower organizations to make informed decisions. Understanding these elements supports efficient operations and enhances competitive advantage.
Rise of Artificial Intelligence and Machine Learning Integration
Artificial Intelligence (AI) and Machine Learning (ML) are integral to the advancement of data analysis software. These technologies facilitate the automation of data processing and interpretation, significantly reducing the time to gain insights. AI-driven software can analyze vast data streams with unprecedented accuracy. Emerging tools leverage algorithms to predict outcomes based on historical data. This enables organizations to identify trends, optimize resources, and drive strategic decisions.
The implementation of AI also enhances user experience. By streamlining functionalities, software can provide recommendations tailored to user needs. This leads to an improved decision-making process and greater operational efficiency. Furthermore, companies can harness predictive analytics to innovate products or services based on market demand.
Cloud-Based Solutions on the Increase
Cloud technology is reshaping how data analysis software is deployed. Cloud-based solutions provide flexibility and enhance accessibility, allowing users to work from any location. This trend promotes collaboration among teams, as multiple users can access and analyze data simultaneosly. The infrastructure is generally more cost-effective, alleviating the burden of hardware maintenance.
Security has become a pivotal consideration with the growth of cloud-based tools. Reputable platforms invest heavily in security measures to protect sensitive data. Moreover, cloud-based solutions often come with automatic updates, ensuring users always have access to the latest features.
In summary, the integration of AI and the rise of cloud-based solutions significantly shape the future landscape of data analysis software. These trends not only enhance current capabilities but also prepare users for future challenges. By embracing these developments, organizations position themselves to leverage their data effectively, fostering a data-driven culture.
Ending
Understanding the nuances of selecting data analysis software is critical in todayโs data-driven world. This article has explored a range of tools, highlighting their key features, strengths, and limitations. Users must recognize that the right choice of software can significantly enhance the effectiveness of their data analysis processes.
It is important to consider essential elements such as usability, integration capabilities, and cost when making a selection. Each organization will have different needs based on their industry requirements, team expertise, and budgetary constraints. Hence, investment in the right software can lead to improved decision-making and operational efficiency.
Users should also be aware of the evolving landscape of data analysis tools. Keeping abreast of future trends like AI integration and cloud-based solutions can provide organizations with a competitive edge.
"The right data analysis software not only simplifies the analysis process but also opens doors to new insights that drive strategic decisions."
In making a final decision, stakeholders should outline their goals and requirements clearly. This helps in shortlisting tools that closely align with their objectives. Regular feedback and user reviews also play a critical role in evaluating the effectiveness of the chosen software.
By synthesizing information presented throughout this article, professionals can feel empowered to choose data analysis software that truly meets their needs. The consequences of this choice can extend beyond mere analysis, impacting overall business performance. The thorough analysis in this guide serves to equip software developers, IT professionals, and students alike with the crucial information needed to navigate the complex landscape of data analysis tools effectively.
With careful consideration and informed choices, organizations can harness the full potential of their data for enhanced insights and better outcomes.