Understanding SPSS for Effective Survey Analysis

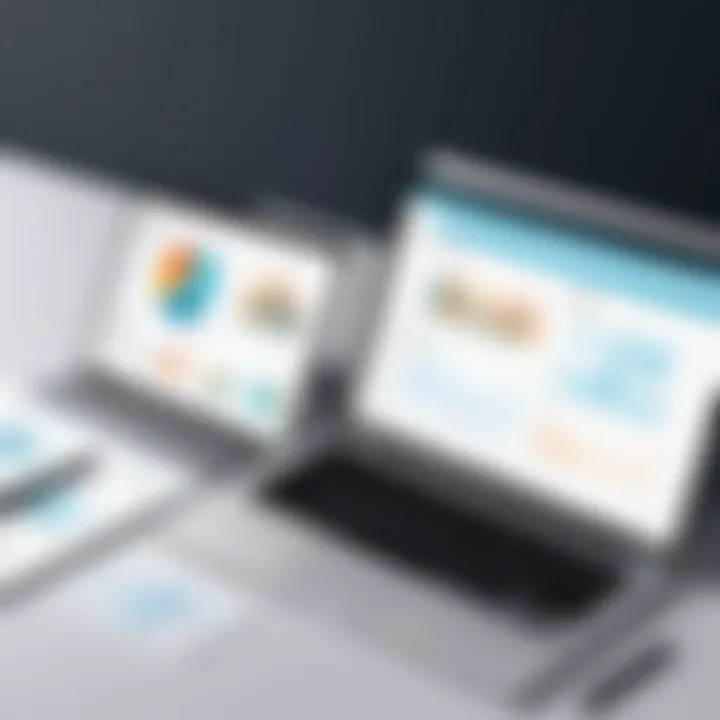
Intro
SPSS, which stands for Statistical Package for the Social Sciences, is a powerful software package widely used for statistical analysis in social science research. It enables users to perform complex data analysis, making it essential for anyone involved in survey research. In todayโs data-driven world, understanding how to navigate SPSS can greatly enhance the quality of survey data analysis and interpretation.
This article provides a comprehensive guide to understanding SPSS, emphasizing its functionalities relevant to survey analysis. We will cover various aspects of the software, from its purpose and key features to its performance and user interface. The goal is to equip professionals and students with the necessary skills to effectively analyze survey results, ensuring validity and actionable insights.
Foreword to SPSS and Surveys
The intersection of SPSS and survey research plays a pivotal role in deriving insights from collected data. SPSS, or Statistical Package for the Social Sciences, is a powerful tool that simplifies the analysis process for researchers across various fields. Understanding how to utilize SPSS effectively can significantly enhance the quality of survey analysis, making it essential for software developers, IT professionals, and students involved in data analysis.
When researchers conduct surveys, they gather valuable information that can inform decisions or drive changes in policies. However, the mere act of collecting data does not imply understanding or usefulness. Thus, processing this information accurately and meaningfully becomes critical. SPSS offers functionalities that aid in transforming raw survey data into comprehensible results.
Key points to consider in this section include:
- The necessity of software like SPSS in handling complex datasets.
- The role of surveys in collecting structured insights.
- The ability to derive actionable conclusions through statistical methods.
This section delves into the essential tools and methodologies encompassed within SPSS, providing a framework that supports rigorous analysis of survey data.
Setting Up SPSS for Survey Data Analysis
Setting up SPSS for survey data analysis is a critical step in ensuring that your data is prepared for meaningful insights. This process involves several important elements that directly impact the efficacy of your analysis. Correct installation and configuration of SPSS are foundational. Additionally, understanding file formats is vital, as different formats can dictate how data is imported and manipulated within SPSS. Effective setup helps in managing the data lifecycle, from entry to analysis, making it easier to derive conclusions from survey results.
Installation and Configuration
The installation of SPSS is a straightforward process, but careful attention to detail is essential. Start by obtaining the official SPSS installer from IBMโs website or a licensed distributor. Ensure your system meets the softwareโs requirements regarding hardware and operating systems. After downloading, run the installer. It will prompt you through a series of steps, which include accepting the license agreement and choosing installation preferences. It is recommended to select the default settings unless you have specific configurations in mind.
Once installed, open SPSS and ensure that all features function as expected. Check for updates immediately after installation; this step guarantees that you have the most recent features and security patches. You may also want to explore the configuration settings within SPSS. Setting default options for data handling, output displays, and graphical representations can streamline your analysis processes significantly.
Understanding File Formats
File formats in SPSS are crucial for proper data handling. SPSS can import and export several formats, including but not limited to .sav (SPSS data file), .por (SPSS portable file), .csv (comma-separated values), and .xls or .xlsx (Excel files). Each format serves different purposes and has unique characteristics.
- .sav is the standard file format for SPSS data, preserving variable setups and value labels.
- .por is designed for sharing files across different SPSS versions, ensuring compatibility.
- .csv offers simplicity in structuring data but lacks the metadata typically associated with SPSS files.
- .xls and .xlsx facilitate data manipulation in Excel prior to importation into SPSS, allowing early checks.
Understanding these formats is essential for data input, enabling efficient data manipulation and versatility in analysis. Each format comes with its own limitations regarding metadata and structure, so choosing the appropriate one according to your dataset's needs will significantly influence the analysis outcomes.
Data Entry and Management in SPSS
In the realm of survey analysis, data entry and management in SPSS are foundational elements. Proper handling of survey data sets the stage for successful analysis and enhances the reliability of results. Given the vast amount of data collected from surveys, effective entry and management strategies must be employed to ensure accuracy and efficiency. Mismanagement can lead to errors that distort analysis, which is why understanding these processes is vital.
Importing Survey Data
Importing survey data into SPSS involves bringing raw information from various sources into the software for processing. Data can come from spreadsheets, databases, or direct survey tools like Google Forms. The import process typically works well with file formats like CSV, Excel, and TXT. Here is how you can easily bring your data into SPSS:
- File Format Selection: Choose the correct file format. If you have been working with Excel, ensuring your data is saved as an .xlsx or .xls format is crucial.
- Open Data in SPSS: Start SPSS and navigate to "File". From there, select "Open" and choose "Data".
- Select the File: Browse to find your data file and ensure the settings match your data's structure.
- Check for Errors: After importing, itโs advisable to review the data for any potential issues, especially with variable types and missing values.
By following these steps closely, users can seamlessly import their survey data for further analysis.
Data Cleaning and Preparation
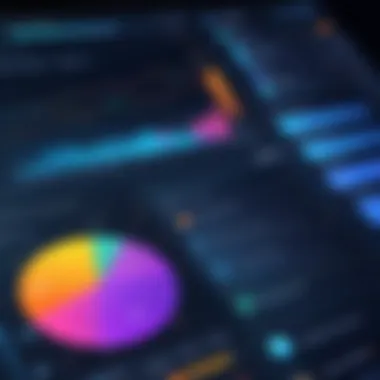
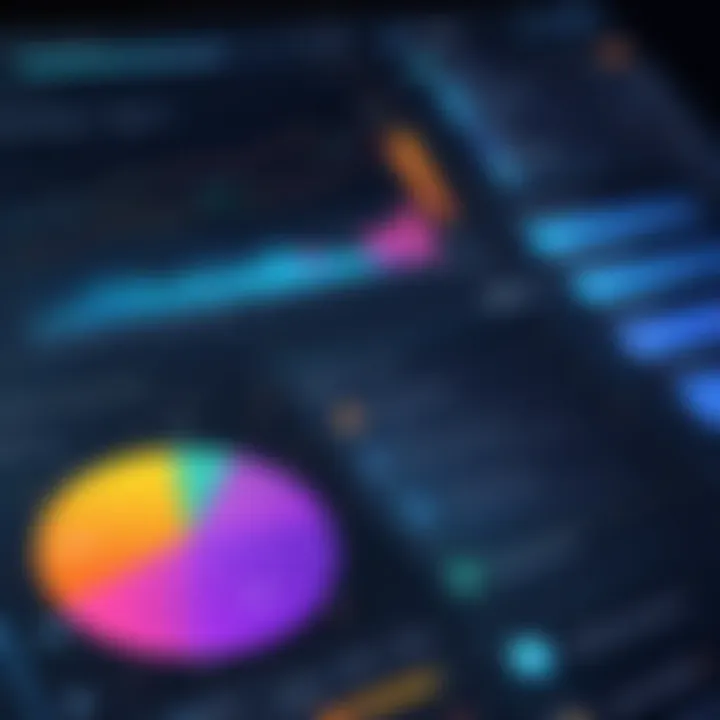
Once imported, the next step is data cleaning and preparation. This process is critical in ensuring that the data is fit for analysis. Several common tasks must be completed:
- Identifying and Handling Missing Values: Use SPSS tools to pinpoint where data may be missing. Depending on the research design, you may opt to exclude these cases or substitute values based on the context.
- Validating Data Accuracy: Check for outliers and incorrect entries. Validating ensures that the data points represent the intended responses accurately and that anomalies do not skew results.
- Consistency Checks: Standardize entries such as categorical variables. For example, practicing uniformity in how responses like 'Yes/No' are recorded is essential for effective analysis.
Data cleaning might seem tedious but is necessary to establish a robust dataset for analysis.
Creating Variables for Analysis
In SPSS, creating appropriate variables is crucial for efficient analysis of survey data. Variables serve as the functional pillars of your dataset. They can represent various aspects of respondent data. Here are important considerations:
- Define Variable Types: Identify whether each variable is nominal, ordinal, interval, or ratio. Understanding this allows SPSS to treat the data correctly during analysis.
- Labeling for Clarity: Assign clear and meaningful labels to each variable. This improves readability and makes it easier to interpret results in the analysis phase.
- Creating New Variables: Often, it may be necessary to derive new variables from existing ones. For example, if analyzing age data, you might categorize age ranges into groups such as "18-24" or "25-34". In SPSS, this can be accomplished by utilizing the "Transform" menu to compute new variables.
Creating variables effectively ensures that researchers can analyze data in a way that is relevant and tailored to their specific questions.
Proper data entry and management is essential for deriving valid insights from surveys. Neglecting this can compromise the entire analysis process.
Statistical Techniques for Survey Analysis
Statistical techniques are pivotal in analyzing survey data. They help extract meaningful patterns from raw data, thereby equipping researchers and stakeholders with insights that guide decision-making processes. With SPSS, users can employ a variety of statistical techniques tailored to their specific research objectives. Choosing the right technique is fundamental. It depends on the nature of the data and what the researcher aims to uncover. Statistical analysis enhances the validity of survey results and provides a framework for rigorous research.
Descriptive Statistics
Descriptive statistics summarize and describe the characteristics of data. This area is significant to this article as it allows for a concrete understanding of the data before any advanced analysis. The key characteristic is its ability to present data in a clear and concise manner.
Frequency Distributions
Frequency distributions depict how often each value appears in the dataset. This aspect is essential because it provides a clear snapshot of the data. Frequency distributions are considered beneficial for initial data review because they help identify patterns or anomalies swiftly. A unique feature of frequency distributions is the ease with which they can be visualized in SPSS, either through graphs or tables. This visualization aids in quick analysis but should not be the sole aspect relied on since it may overlook nuances in distribution.
Central Tendency Measures
Central tendency measures include the mean, median, and mode. These measures are vital for understanding the average or typical response within survey data. Their popularity stems from how effectively they provide a central point around which data values cluster. The unique aspect of these measures is that they can guide the interpretation of results in context. For example, if a survey question produces a notably high or low mean, it can trigger further investigation. However, central tendency measures can be misleading if the data distribution is skewed, as they donโt account for variability among responses.
Dispersion Measures
Dispersion measures, such as range, variance, and standard deviation, quantify the variability within data. This aspect is crucial, as understanding variability is as important as knowing central values. Dispersion measures are beneficial because they offer insight into the spread of data points. The unique feature is that they help to understand how consistent or inconsistent responses are within the dataset. On a downside, some dispersion measures can be sensitive to outliers, which may skew results and lead to incorrect conclusions.
Inferential Statistics
Inferential statistics enable researchers to make conclusions or predictions based on sample data. This section adds depth to survey analysis by allowing generalizations beyond just the collected data. It is popular because it informs decision-making based on statistical evidence from sampled data.
Hypothesis Testing
Hypothesis testing assesses the validity of assumptions about a population based on sample data. Its significance lies in its structured approach to establishing statistical significance. The key characteristic is its capability to provide a basis for making inferences about the population. This technique is beneficial for confirming or rejecting claims made in the research. However, reliance on p-values can mislead interpretations if not contextualized with other findings.
Regression Analysis
Regression analysis assesses relationships between variables, which is crucial for understanding causation. This technique is considered valuable because it allows researchers to predict the outcome of one variable based on another. A unique feature of regression analysis is its flexibly accommodating multiple independent variables. However, misinterpretations can occur if the assumptions underlying regression analysis are breached, leading to flawed conclusions.
Chi-Square Tests
Chi-square tests determine if there is a significant association between categorical variables. This technique contributes to this article by exploring relationships within non-numeric survey data. The key characteristic is its robustness in handling different data sizes and structures. It provides a straightforward method for testing relationships. However, it requires a large sample size for reliable results, as small samples can lead to unstable estimates.
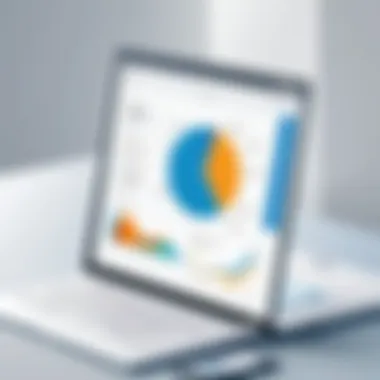
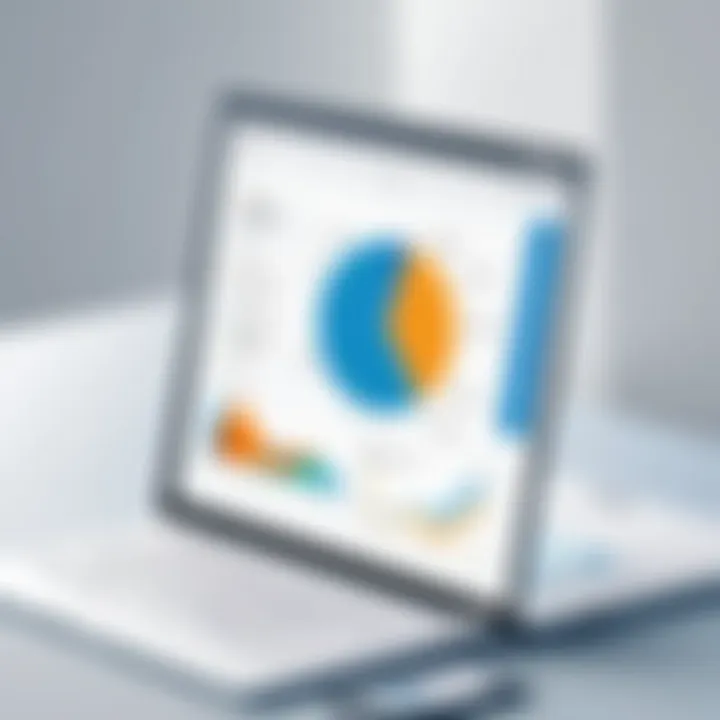
Advanced Statistical Procedures
Advanced statistical procedures expand on basic techniques, offering deeper analysis capabilities. These procedures are critical for uncovering complex relationships and patterns among variables.
Factor Analysis
Factor analysis reduces data dimensions by identifying underlying relationships between variables. Its contribution to survey analysis is significant because it simplifies data interpretation. This technique is beneficial for grouping correlated variables into factors, facilitating analysis. However, it requires careful interpretation as oversimplification can lead to lost relevant information.
Cluster Analysis
Cluster analysis categorizes data into distinct groups based on similarities. Its primary aspect is its ability to identify patterns and group respondents with similar characteristics. This technique is useful for market segmentation in surveys. Nonetheless, the challenge lies in determining the number of clusters, which can influence results drastically.
Path Analysis
Path analysis examines causal relationships among variables in a model framework. This method contributes by providing insights into direct and indirect paths between variables. The key characteristic is its visualization capabilities in SPSS, allowing users to depict relationships graphically. However, path analysis assumes linear relationships, which may not always be appropriate, leading to potential misinterpretations.
Key Insight: Understanding and employing these statistical techniques ensures reliable conclusions drawn from survey data. They not only reveal patterns but also guide effective decision-making strategies.
Interpreting SPSS Output
Interpreting the output from SPSS is crucial in deriving meaningful insights from survey data. The software generates various tables and charts that summarize the results of statistical analyses. Understanding how to read this output accurately enables researchers to make informed decisions based on the data. Whether in an academic, corporate, or professional setting, grasping the nuances of SPSS output is essential for credibility in data interpretation and presentation.
Reading SPSS Output Tables
SPSS organizes its output into tables that present data in a structured format. Each table conveys specific information about the analysis performed. Here are some key aspects to note when reading these tables:
- Variable Names: At the top of each table, you will find the names of the variables analyzed. This is the entry point to understand what data is being viewed.
- Statistics: Each table includes statistical measures relevant to the analysis. For example, in descriptive statistics tables, you might see means, medians, and mode listed alongside other measures.
- Significance Values: For inferential statistics, pay attention to p-values. A p-value less than 0.05 typically indicates a statistically significant result, guiding researchers in their conclusion-making.
Familiarity with these elements will enhance your ability to extract insights quickly from the output tables.
Understanding Statistical Significance
Statistical significance helps to determine if the results of the analysis are due to random chance or indicative of true relationships in the data. In SPSS, this is primarily assessed through p-values. An understanding of the following concepts is beneficial:
- P-Values: A p-value is the probability of observing the results given that the null hypothesis is true. A lower p-value suggests stronger evidence against the null hypothesis.
- Confidence Intervals: These provide a range of values that is likely to contain the population parameter. If a confidence interval does not include zero for a mean difference, it suggests a statistically significant effect.
- Effect Size: It offers insight into the magnitude of the difference rather than just its significance. Effect size can be more informative than p-values alone.
Recognizing these components enables researchers to evaluate the relevance and reliability of their analysis rigorously.
Presenting Results Clearly
Effective communication of findings is as important as the analysis itself. When presenting SPSS output, consider these techniques:
- Simplify Tables: Adjusting the layout to highlight key results can aid the audience's understanding. Avoid cluttering tables with excessive detail that may distract from the core findings.
- Visual Aids: Integrating charts and graphs based on SPSS output can make complex data more accessible. Visual representations can often convey messages in a clearer manner compared to numerical tables alone.
- Narrative Commentary: Provide context around the data. Explanation of key findings in conjunction with the tables helps in making the data more relatable.
"Clear results presentation enhances understanding and facilitates better decision-making."
In summary, the skill of interpreting SPSS output is fundamental in turning raw data into actionable insights. Acknowledge the significance of each output component to elevate your survey analysis effectively.
Common Challenges in Survey Analysis with SPSS
Survey data analysis often presents several challenges that users must overcome to ensure accurate and meaningful results. Understanding these common challenges is central to effectively utilizing SPSS for survey analysis. Addressing aspects such as missing data and non-normal data distributions is crucial because they can profoundly influence the integrity of the analyses performed. By recognizing these issues, researchers and analysts can adopt strategies that contribute to the validity and reliability of their findings.
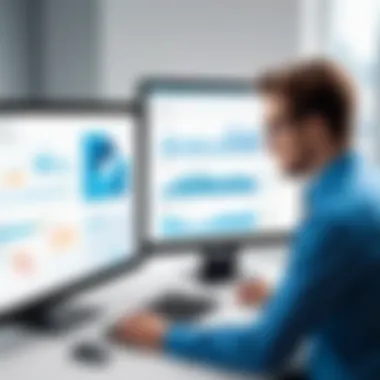
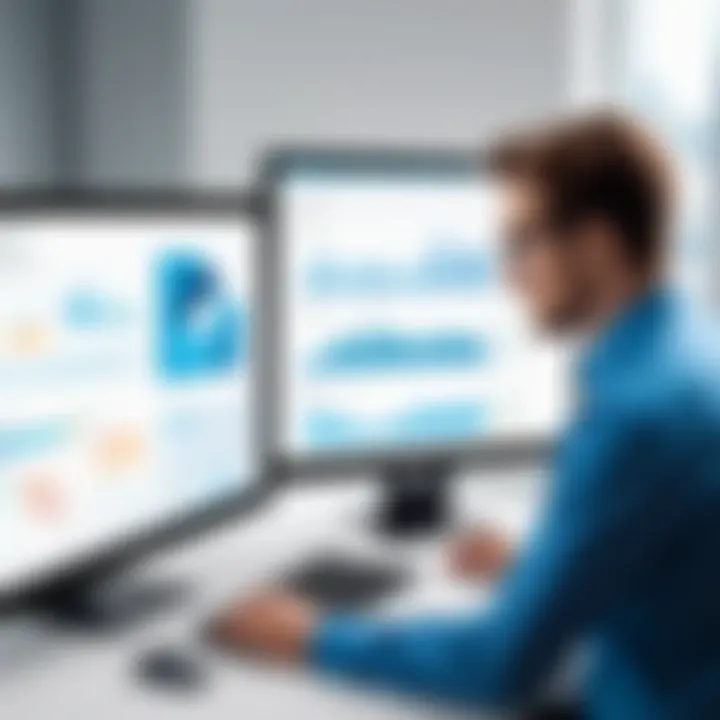
Dealing with Missing Data
Missing data is a prevalent issue in survey research. Respondents may skip questions or refuse to answer certain sections, leading to gaps in the dataset. This missing information can introduce bias or result in incomplete analysis. In SPSS, there are several methods to address missing data, including:
- Listwise Deletion: This method excludes any case (respondent) from the analysis if they have any missing values. While simple, this can significantly reduce the sample size and may not be ideal, especially if data loss is substantial.
- Pairwise Deletion: Unlike listwise deletion, pairwise deletion uses all available data points for analyses. This method keeps more data but can complicate interpretations when results vary across different analyses.
- Imputation Techniques: SPSS provides several imputation methods whereby missing values are estimated using available data. Techniques include mean substitution, regression imputation, or utilizing more complex models available in the software.
It is vital to assess the nature of the missing data before selecting a method. The source of missingness, whether it is random or systematic, can dictate the appropriateness of each strategy. Proper handling of missing data contributes to producing more credible and robust conclusions.
Navigating Non-Normal Data Distributions
Another challenge encountered in survey analysis is dealing with non-normal data distributions. Many statistical tests assume that the underlying data follows a normal distribution. However, real survey data often displays skewness or kurtosis, which can distort test outcomes.
SPSS offers various options to assess and address non-normal distributions, such as:
- Descriptive Statistics: Using SPSS, analysts can generate histograms and Q-Q plots to visually inspect distribution shapes. If the data is not normally distributed, this requires further action.
- Transformations: Application of transformations like logarithmic, square root, or reciprocal can sometimes normalize the distribution, making it suitable for traditional parametric tests.
- Non-Parametric Tests: If transformation is not effective, analysts can opt for non-parametric tests, which do not assume a normal distribution. SPSS contains various non-parametric tests such as the Mann-Whitney U test or Kruskal-Wallis test, which can provide robust alternatives.
Understanding these non-normal data characteristics is crucial. It allows researchers to choose appropriate analytical techniques that yield credible results, ultimately leading to informed decision-making based on survey findings.
Best Practices for Survey Analysis
In the realm of survey analysis, adhering to best practices is essential. These practices ensure that the data collected is reliable, valid, and ultimately useful for decision-making. Understanding these best practices can dramatically improve the quality of insights drawn from survey data, making this topic of great significance for those working with SPSS.
Ensuring Validity and Reliability
Reliability and validity are cornerstone concepts in survey research. Reliability refers to the consistency of a measure. It means that if an identical survey were administered multiple times, the outcomes would yield similar results. Validity, on the other hand, assesses whether the survey truly measures what it intends to measure. To ensure both reliability and validity, researchers should follow several steps:
- Development of Clear Questions: Formulate questions that are simple and unambiguous. Avoid complex language or jargon.
- Pilot Testing: Conduct a pilot test of the survey on a small sample to identify potential issues. Analyze feedback to refine questions.
- Use Established Scales: When assessing constructs, consider using established measurement scales that have already demonstrated validity.
- Check for Response Bias: It is critical to identify any factors that might influence how respondents answer questions. Selecting a diverse sample can help mitigate bias.
Maintaining both reliability and validity throughout the analysis stage is equally crucial. Utilize SPSS tools to check for consistency and adjust the analysis of those variables as necessary.
Ethical Considerations in Survey Research
Ethical considerations in survey research are paramount and must not be overlooked. Researchers must ensure that they conduct surveys in a manner that respects participants' rights and integrity. Key ethical considerations include:
- Informed Consent: Participants should be fully aware of the nature of the survey, what it entails, and how their data will be used before they agree to participate.
- Anonymity and Confidentiality: Guarantee participants that their responses will remain anonymous and confidential. Using anonymous survey methods can enhance the likelihood of honest responses.
- Right to Withdraw: Participants should always have the option to withdraw from the survey without facing any penalties or repercussions.
- Debriefing: After participation, provide a debriefing that explains the purpose of the study. This can help participants feel appreciated and respected, even if they did not withhold information.
By prioritizing ethical standards, researchers ensure that the findings are not only credible but also respectable within the community. This enhances the overall quality of the analysis and its subsequent interpretations.
Remember: Ethical research practices do not just protect participants; they also enhance the quality of the data collected.
Incorporating best practices for survey analysis, such as ensuring validity and reliability alongside ethical considerations, lays the groundwork for robust survey outcomes. Doing so not only fosters public trust but also enhances the integrity of the resulting analysis.
Ending
In this section, we emphasize the significance of effectively concluding the discussion on SPSS for survey analysis. The conclusion acts as a synthesis point, where key insights gleaned throughout the article are summarized and reinforced. Its importance lies in helping readers reflect on the critical concepts discussed and how they can apply them in real-world situations.
Recapping Key Points
- Understanding SPSS: The software stands out as a powerful tool that simplifies the survey data analysis process. Learners and practitioners benefit from mastering its features.
- Survey Design and Integrity: Recognizing the importance of survey validity and reliability ensures that results are credible. Proper setup and ethical considerations are vital for meaningful insights in research.
- Statistical Techniques: Various statistical methods, including descriptive and inferential techniques, offer robust tools for data examination. Knowledge of these aids in interpreting findings accurately.
- Interpreting Results: The clarity of presentation can influence decision-makers. Extracting actionable insights from SPSS output is crucial for translating data into effective strategies.
- Addressing Challenges: Being aware of potential issues like missing data and non-normal distributions prepares analysts to handle complications effectively, ensuring reliable results.
Future Directions in Survey Analysis
As technology and methodologies evolve, so does the landscape of survey analysis. Below are several prospects to consider:
- Integration with AI: Utilizing artificial intelligence to improve data analysis capabilities can streamline processes, offering predictive insights from survey data.
- Emphasis on Real-time Analytics: Adopting real-time data analysis methods allows researchers to act swiftly on emerging trends, making analyses more dynamic and relevant.
- User Training: As SPSS becomes more sophisticated, comprehensive training programs will be essential. Educating users guarantees better adoption and effective use of the software's full capabilities.
- Mobile Survey Tools: Innovations in mobile technology can shift surveying methods. Respondents may prefer to participate via mobile devices, necessitating adjustments in data collection strategies.
- Collaboration Across Disciplines: Fostering cross-disciplinary collaborations can yield richer data insights, encouraging integration of diverse perspectives in survey analysis.
By being aware of these evolving factors, professionals can remain at the forefront of survey research. The journey through SPSS for analyzing survey data should not end here; ongoing learning and adaptation will strengthen outcomes and insights.